A Virtual Private Network (VPN) is a technology that creates a secure, encrypted connection between a user’s device and a remote server. This connection allows users to access the internet privately and securely by routing their traffic through the VPN server. When connected to a VPN, the user’s device establishes an encrypted tunnel to the VPN server, which then acts as an intermediary between the user and the internet.
The primary functions of a VPN include:
1. Masking the user’s IP address, making it appear as if their internet traffic is originating from the VPN server’s location.
2.Encrypting data transmitted between the user’s device and the VPN server, protecting it from potential interception by third parties.
3. Providing privacy from internet service providers, hackers, and government agencies by concealing the user’s online activities.
VPNs also offer additional benefits:
1. Bypassing geo-restrictions and censorship by allowing users to connect to servers in different countries, granting access to region-locked content.
2.Enhancing security when using public Wi-Fi networks by encrypting data and protecting it from potential threats.
3. Enabling users to access content that may be blocked in their region, such as streaming services, websites, and social media platforms.
In summary, VPNs are valuable tools for maintaining online privacy, security, and freedom while browsing the internet, offering protection against various online threats and restrictions.
Key Takeaways
- A VPN (Virtual Private Network) is a secure connection that allows you to access the internet privately and securely by routing your connection through a server and hiding your online activities.
- When choosing a VPN service, consider factors such as security features, server locations, speed, and compatibility with your devices and operating systems.
- Installing and setting up a VPN on your device is usually a straightforward process, often involving downloading and installing the VPN app, logging in, and connecting to a server.
- Connecting to a VPN server can be done with a simple click or tap within the VPN app, and you can choose a server location based on your specific needs, such as accessing geo-restricted content.
- Using a VPN for secure browsing and streaming allows you to encrypt your internet connection, protect your data from hackers, and access content that may be restricted in your location.
- Common VPN issues such as slow connection, server connectivity problems, and DNS leaks can often be resolved by troubleshooting steps such as switching servers, updating the VPN app, or contacting customer support.
- To maximize your VPN experience, consider using a kill switch feature, regularly updating your VPN app, and being mindful of your online activities to ensure privacy and security.
Choosing the Right VPN Service for Your Needs
Server Location and Availability
A crucial aspect to consider is the number of servers offered by the VPN provider in various locations worldwide. This enables access to content from different regions and ensures a stable and fast connection.
Encryption, Security, and Logging Policy
The level of encryption and security protocols offered by the VPN service is also vital. Look for providers that offer strong encryption standards like AES-256 bit encryption and support for secure protocols such as OpenVPN or IKEv2. Furthermore, consider the logging policy of the VPN provider. Opt for a service with a strict no-logs policy, ensuring your online activities are not tracked or stored, and your data is not collected or shared with third parties.
Speed, Performance, and Compatibility
The speed and performance of the VPN service are also essential factors. Look for providers that offer fast and reliable connections, as slow speeds can be frustrating when streaming or downloading content. Additionally, consider the compatibility of the VPN service with your devices and operating systems. Choose providers that offer apps for various platforms, including Windows, Mac, iOS, Android, and even routers.
By taking these factors into account, you can select a VPN service that meets your specific needs and provides a secure and reliable browsing experience.
Installing and Setting Up the VPN on Your Device
Once you have chosen a VPN service, the next step is to install and set it up on your device. Most VPN providers offer user-friendly apps for various platforms, making the installation process relatively straightforward. To begin, you will need to sign up for a subscription with the VPN provider and download the appropriate app for your device from their website or app store.
Once the app is downloaded, follow the on-screen instructions to install it on your device. After the installation is complete, you will need to log in to the app using the credentials provided by the VPN provider. Once logged in, you can customize the settings according to your preferences, such as choosing a server location or enabling features like kill switch or split tunneling.
Additionally, some VPN apps offer advanced settings for more experienced users, allowing you to customize protocols, encryption standards, and DNS settings. Once you have configured the settings to your liking, you can connect to a VPN server with just a click of a button and start browsing the internet securely and privately.
Connecting to a VPN Server
Connecting to a VPN server is a simple process that can be done with just a few clicks using the VPN app on your device. Once you have installed and set up the VPN app, open it and log in using your credentials. From the app dashboard, you will typically see a list of available servers in different locations around the world.
You can choose a server location based on your specific needs, such as accessing geo-restricted content or optimizing for speed. After selecting a server location, simply click on the “connect” button within the app to establish a secure connection to the VPN server. Once connected, your internet traffic will be encrypted and routed through the VPN server, ensuring that your online activities are private and secure.
Some VPN apps also offer features like automatic server selection or favorites list for quick access to preferred servers. Additionally, some apps may display real-time data such as server load and ping times to help you make an informed decision when choosing a server. Overall, connecting to a VPN server is a quick and easy process that provides added security and privacy while browsing the internet.
Using the VPN for Secure Browsing and Streaming
Once connected to a VPN server, you can use the VPN for secure browsing and streaming with peace of mind knowing that your online activities are protected. A VPN encrypts your internet traffic, making it unreadable to anyone who may try to intercept it, such as hackers or government agencies. This means that sensitive information such as passwords, credit card details, and personal messages are kept safe from potential threats.
Furthermore, a VPN allows you to bypass geo-restrictions and access content that may be blocked in your region. For example, you can use a VPN to access streaming services like Netflix, Hulu, or BBC iPlayer from anywhere in the world. By connecting to a server in a different location, you can unblock content and enjoy unrestricted access to your favorite shows and movies.
Additionally, using a VPN can also help prevent bandwidth throttling by your internet service provider, ensuring smooth streaming and faster download speeds. Overall, using a VPN for secure browsing and streaming provides an added layer of protection for your online activities while also giving you access to global content without restrictions.
Troubleshooting Common VPN Issues
Slow Connection Speeds
If you’re experiencing slow connection speeds while using a VPN, there are a few steps you can take to improve your browsing experience. Try connecting to a different server location that’s closer to your physical location. This can help reduce latency and optimize your connection. Additionally, consider using a wired Ethernet connection instead of Wi-Fi when possible to ensure a stable and fast connection.
Difficulty Connecting to Servers or Unexpected Disconnections
If you’re having trouble connecting to servers or experiencing unexpected disconnections, try switching between different VPN protocols within the app settings. Some protocols may work better than others depending on your network environment. You can also try updating the VPN app to the latest version or reinstalling it if necessary.
Further Troubleshooting Steps
If the above steps don’t resolve the issues, don’t worry! You can reach out to the customer support team of your VPN provider for further assistance. They can provide guidance and support to help resolve any technical issues you may be experiencing.
Tips for Maximizing Your VPN Experience
To maximize your VPN experience and get the most out of your subscription, consider implementing the following tips: 1. Enable automatic startup: Set up your VPN app to launch automatically when you start your device to ensure that you are always protected when browsing the internet. 2. Use split tunneling: Some VPN apps offer split tunneling features that allow you to route only specific traffic through the VPN while leaving other traffic unaffected. This can be useful for optimizing performance or accessing local services while connected to the VPN. 3. Regularly update your app: Keep your VPN app up to date with the latest version to ensure that you have access to new features and security updates. 4. Use multi-device support: Take advantage of multi-device support offered by many VPN providers to protect all of your devices with a single subscription. 5. Stay informed: Stay informed about online privacy and security best practices by following reputable sources and staying up to date with current events related to cybersecurity. By following these tips and best practices, you can maximize your VPN experience and enjoy secure and private browsing while accessing global content without restrictions.
If you’re interested in setting up a home data center, you may also want to check out this comprehensive guide to setting up a home data center. It provides valuable insights and tips for creating a reliable and efficient data center in your own home.
FAQs
What is a VPN?
A VPN, or Virtual Private Network, is a technology that creates a secure and encrypted connection over a less secure network, such as the internet. It allows users to securely access a private network and share data remotely through public networks.
Why should I use a VPN?
There are several reasons to use a VPN, including protecting your online privacy, securing your internet connection, accessing geo-restricted content, and bypassing censorship.
How do I set up a VPN?
To set up a VPN, you can either use a VPN service provider and follow their specific instructions for installation and configuration, or set up your own VPN server using software like OpenVPN.
What devices can I use a VPN on?
You can use a VPN on a wide range of devices, including computers, smartphones, tablets, routers, and even some smart TVs and gaming consoles.
Is using a VPN legal?
In most countries, using a VPN is legal. However, it’s important to note that using a VPN for illegal activities, such as hacking or accessing illegal content, is still illegal regardless of whether you use a VPN or not.
How do I use a VPN?
Once you have set up a VPN, you can typically connect to it by opening the VPN app or software on your device, entering your login credentials, and selecting a server location to connect to.
A Virtual Private Network (VPN) is a technology that creates a secure, encrypted connection between a user’s device and a remote server. This connection forms a private tunnel through the public internet, enhancing privacy and security for online activities. VPNs mask the user’s IP address and encrypt their data, making it difficult for third parties to track or intercept their online communications.
VPNs function by routing internet traffic through a server operated by the VPN provider. When a user connects to a VPN, their data is encrypted before leaving their device. This encrypted data travels through the VPN server, which acts as an intermediary between the user and the internet.
The server then decrypts the data and forwards it to its intended destination on the internet. Responses from websites or online services follow the reverse path, being encrypted by the VPN server before reaching the user’s device. This process offers several benefits:
1.Enhanced privacy: By masking the user’s real IP address, VPNs make it harder for websites, advertisers, and other online entities to track user activities.
2. Improved security: The encryption used by VPNs protects data from interception by malicious actors, especially when using public Wi-Fi networks.
3. Access to geo-restricted content: VPNs can make it appear as if the user is accessing the internet from a different location, potentially bypassing geographical restrictions on content.
4.Circumvention of censorship: In countries with internet censorship, VPNs can help users access blocked websites and services. 5. Protection against some forms of cyber attacks: VPNs can help mitigate certain types of cyber threats, such as man-in-the-middle attacks.
VPNs are widely used by individuals concerned about online privacy, as well as by businesses to secure remote access to corporate networks and protect sensitive data transmissions. However, it’s important to note that while VPNs significantly enhance online privacy and security, they are not a complete solution and should be used in conjunction with other security measures.
Key Takeaways
- A VPN, or Virtual Private Network, is a tool that creates a secure connection over the internet, allowing users to protect their privacy and security while browsing.
- A VPN protects your privacy by encrypting your internet traffic and hiding your IP address, making it difficult for third parties to track your online activity.
- The encryption process in a VPN involves scrambling your data so that it becomes unreadable to anyone trying to intercept it, ensuring that your information remains secure.
- Using a VPN can help secure your online activity by preventing hackers, ISPs, and government agencies from monitoring your internet usage and accessing your personal data.
- When choosing a VPN, consider factors such as the level of encryption, server locations, speed, and compatibility with your devices to ensure it meets your specific needs.
How does a VPN protect your privacy?
Encryption for Secure Browsing
When you use a VPN, all of your internet traffic is routed through an encrypted tunnel, making it extremely difficult for anyone to intercept and decipher your data. This means that even when using a public Wi-Fi network, such as in a coffee shop or airport, your online activities are protected from potential hackers or snoopers who may be trying to access your personal information.
Hiding Your IP Address
In addition to encrypting your data, a VPN also hides your IP address. Your IP address is a unique identifier that can be used to track your online activities and determine your physical location. By using a VPN, you can mask your IP address and make it appear as though you are accessing the internet from a different location.
Accessing Geo-Restricted Content
This not only helps to protect your privacy, but it also allows you to bypass geo-restrictions and access content that may be blocked in your current location.
The encryption process in a VPN
The encryption process in a VPN is a crucial aspect of how it protects your online privacy and security. When you connect to a VPN server, all of your internet traffic is encrypted using various encryption protocols such as OpenVPN, L2TP/IPsec, or IKEv2/IPsec. These protocols use advanced encryption algorithms to scramble your data, making it unreadable to anyone who may try to intercept it.
This ensures that your sensitive information, such as passwords, credit card numbers, and personal messages, remains secure and private. The encryption process in a VPN involves the use of encryption keys, which are used to both encrypt and decrypt the data being transmitted. These keys are generated using complex mathematical algorithms and are unique to each VPN connection.
This means that even if someone were able to intercept your encrypted data, they would not be able to decipher it without the proper encryption key. Additionally, many VPN providers also offer features such as Perfect Forward Secrecy, which ensures that even if one encryption key is compromised, past and future communications remain secure.
Securing your online activity with a VPN
Securing your online activity with a VPN is essential for protecting your privacy and security while browsing the internet. When you use a VPN, all of your internet traffic is encrypted and transmitted through a secure tunnel, making it extremely difficult for anyone to intercept and decipher your data. This means that even if you are using an unsecured Wi-Fi network, such as in a hotel or airport, your online activities remain private and secure.
In addition to encrypting your data, a VPN also provides protection against potential threats such as hackers, identity thieves, and government surveillance. By masking your IP address and making it appear as though you are accessing the internet from a different location, a VPN helps to prevent tracking of your online activities and allows you to bypass geo-restrictions. This can be particularly useful for accessing streaming services, social media platforms, and other websites that may be blocked in certain countries.
Choosing the right VPN for your needs
When choosing the right VPN for your needs, there are several factors to consider in order to ensure that you get the best possible protection for your online activities. One of the most important factors to consider is the level of encryption offered by the VPN provider. Look for providers that offer strong encryption protocols such as OpenVPN or IKEv2/IPsec, as well as features like Perfect Forward Secrecy, which ensures that your data remains secure even if one encryption key is compromised.
Another important consideration when choosing a VPN is the provider’s logging policy. Some VPN providers may keep logs of your online activities, which could potentially compromise your privacy. Look for providers that have a strict no-logs policy, meaning that they do not keep any records of your online activities.
Additionally, consider the provider’s jurisdiction and whether they are subject to data retention laws or government surveillance.
Setting up and using a VPN
Getting Started with a VPN
Most VPN providers offer user-friendly apps that make it easy to connect to their servers and encrypt your internet connection with just a few clicks. After signing up for a VPN service, simply download the app onto your device, log in with your credentials, and choose a server location to connect to.
Enhanced Privacy and Security
Once connected to the VPN server, all of your internet traffic will be encrypted and transmitted through a secure tunnel, providing you with enhanced privacy and security while browsing the internet. You can also choose different server locations to access geo-restricted content or bypass censorship in certain countries.
Additional Features and Customization
Many VPN apps also offer additional features such as ad-blocking, malware protection, and split tunneling, which allows you to route only specific traffic through the VPN while leaving the rest unaffected.
Common misconceptions about VPNs
There are several common misconceptions about VPNs that can lead to misunderstandings about their capabilities and limitations. One common misconception is that using a VPN will make you completely anonymous online. While a VPN can help to mask your IP address and encrypt your data, it does not make you completely anonymous.
Your online activities may still be traceable through other means such as cookies, browser fingerprinting, or social media accounts. Another misconception is that all VPNs offer the same level of privacy and security. In reality, not all VPN providers are created equal, and some may offer weaker encryption or logging policies that could compromise your privacy.
It’s important to carefully research and choose a reputable VPN provider that offers strong encryption protocols and a strict no-logs policy. In conclusion, a VPN is an essential tool for protecting your online privacy and security. By encrypting your internet connection and hiding your IP address, a VPN helps to ensure that your online activities remain private and secure.
When choosing a VPN provider, consider factors such as encryption protocols, logging policies, and jurisdiction to ensure that you get the best possible protection for your needs. Setting up and using a VPN is relatively straightforward and can provide you with enhanced privacy and security while browsing the internet. However, it’s important to be aware of common misconceptions about VPNs in order to have realistic expectations about their capabilities and limitations.
If you’re interested in learning more about the benefits of cloud computing for business growth, check out this article on Unraveling the Pros and Cons of Cloud Computing for Business Growth. It provides a comprehensive overview of the advantages and disadvantages of cloud computing and how it can impact your company’s growth and efficiency. Understanding the potential of cloud computing can help you make informed decisions about your business’s digital infrastructure and security measures, including the use of VPNs to protect your privacy and secure your online activity.
FAQs
What is a VPN?
A VPN, or Virtual Private Network, is a technology that creates a secure and encrypted connection over a less secure network, such as the internet. It allows users to securely access a private network and share data remotely through public networks.
How does a VPN work?
When you connect to a VPN, your device creates a secure and encrypted connection to the VPN server. All of your internet traffic is then routed through this secure connection, protecting your data from being intercepted by hackers, government agencies, or other third parties.
What are the benefits of using a VPN?
Using a VPN can provide several benefits, including protecting your privacy and anonymity online, securing your internet connection when using public Wi-Fi, bypassing geographic restrictions on websites and streaming services, and preventing your internet service provider from tracking your online activity.
Are VPNs legal to use?
In most countries, using a VPN is legal. However, there are some countries where the use of VPNs is restricted or banned. It’s important to be aware of the laws and regulations regarding VPN usage in your country or the country you are visiting.
Can a VPN be used to access blocked content?
Yes, a VPN can be used to bypass geographic restrictions and access blocked content. By connecting to a VPN server in a different location, you can make it appear as though you are accessing the internet from that location, allowing you to access content that may be restricted in your actual location.
How do I choose the right VPN for me?
When choosing a VPN, it’s important to consider factors such as the level of security and encryption offered, the number and location of servers, the speed and reliability of the connection, and the provider’s privacy policy. It’s also important to consider whether the VPN provider has a no-logs policy, meaning they do not track or store your online activity.
Data center hosting refers to the practice of housing and managing computer systems and associated components, such as servers, storage devices, and networking equipment, in a secure and controlled environment. This is typically done by a third-party provider who specializes in data center services. Data center hosting is crucial for businesses of all sizes as it allows them to store, process, and manage their data in a reliable and secure manner.
One of the key benefits of data center hosting is the ability to offload the burden of managing and maintaining an on-premises data center. By outsourcing these responsibilities to a specialized provider, businesses can focus on their core competencies and leave the technical aspects of data management to the experts. Additionally, data center hosting offers scalability and flexibility, allowing businesses to easily expand their infrastructure as their needs grow.
Key Takeaways
- Data center hosting is crucial for businesses to ensure reliable and secure access to their data and applications.
- When choosing a data center hosting partner, consider factors such as location, uptime guarantees, and customer support.
- Security measures and compliance requirements are essential considerations for data center hosting, including physical security, network security, and regulatory compliance.
- Scalability and flexibility are important features of data center hosting solutions, allowing businesses to easily adjust their resources as needed.
- Network connectivity and latency are critical factors to consider in data center hosting, as they can impact the performance and user experience of applications.
Key Considerations When Choosing a Data Center Hosting Partner
When selecting a data center hosting partner, there are several key considerations that businesses should keep in mind. Firstly, the location of the data center is important as it can impact factors such as latency and accessibility. It is advisable to choose a data center that is geographically close to your business operations to minimize network latency.
Reliability and uptime are also crucial factors to consider. A reputable data center hosting provider should have redundant power sources, backup generators, and robust cooling systems in place to ensure uninterrupted operation. Service level agreements (SLAs) should be carefully reviewed to understand the provider’s commitment to uptime and response times.
Customer support is another important consideration. A reliable data center hosting partner should offer 24/7 customer support to address any issues or concerns that may arise. It is also advisable to research the reputation and experience of potential providers. Look for providers with a proven track record in the industry and positive customer reviews.
Security Measures and Compliance Requirements for Data Center Hosting
Data security is a top priority for businesses, especially in today’s digital landscape where cyber threats are becoming increasingly sophisticated. When choosing a data center hosting partner, it is important to ensure that they have robust physical and network security measures in place.
Physical security measures may include features such as biometric access controls, video surveillance, and 24/7 on-site security personnel. These measures help to prevent unauthorized access to the data center facility and protect the physical infrastructure.
Network security measures are equally important. A reputable data center hosting provider should have firewalls, intrusion detection systems, and other advanced security technologies in place to protect against cyber attacks. Regular vulnerability assessments and penetration testing should also be conducted to identify and address any potential weaknesses in the network.
Compliance requirements are another consideration for businesses, particularly those operating in regulated industries such as healthcare or finance. Data center hosting providers should be able to demonstrate compliance with relevant regulations such as HIPAA or PCI DSS. They should also have policies and procedures in place to ensure data protection and privacy.
Scalability and Flexibility of Data Center Hosting Solutions
Scalability is a key advantage of data center hosting. Businesses can easily scale their infrastructure up or down based on their needs without the need for significant capital investments. This flexibility allows businesses to respond quickly to changing market conditions and accommodate growth without disruption.
Data center hosting providers typically offer a range of scalability options, such as adding or removing servers, storage capacity, or network bandwidth. These options can be customized to meet the specific needs of each business.
Flexibility of infrastructure is another important consideration. Businesses should look for data center hosting providers that offer a wide range of infrastructure options, such as dedicated servers, virtual private servers (VPS), or cloud hosting. This allows businesses to choose the most suitable infrastructure for their specific requirements.
Customization options are also important for businesses with unique needs or specific compliance requirements. A reputable data center hosting provider should be able to offer customized solutions tailored to the specific needs of each business.
Future-proofing is another consideration when choosing a data center hosting partner. Businesses should look for providers that have a roadmap for technology upgrades and are committed to staying at the forefront of industry trends. This ensures that businesses can benefit from the latest advancements in technology without the need for frequent migrations or disruptions.
Network Connectivity and Latency: Factors to Consider in Data Center Hosting
Network connectivity is a critical factor in data center hosting. Businesses should ensure that their data center hosting partner offers reliable and high-speed network connectivity options.
Latency, or the delay in data transmission, is an important consideration. High latency can result in slow response times and poor performance, particularly for applications that require real-time data processing. Businesses should choose a data center hosting provider with low latency connections to ensure optimal performance.
Redundancy and failover are also important considerations. A reliable data center hosting provider should have redundant network connections and failover mechanisms in place to ensure uninterrupted connectivity in the event of a network outage.
Bandwidth and throughput are other factors to consider. Businesses should ensure that their data center hosting partner offers sufficient bandwidth to handle their current and future needs. High throughput is particularly important for businesses that require fast and efficient data transfer, such as those involved in media streaming or large-scale data analytics.
Disaster Recovery and Business Continuity Planning for Data Center Hosting
Disaster recovery and business continuity planning are crucial aspects of data center hosting. Businesses should ensure that their data center hosting partner has robust disaster recovery options in place to protect against potential disruptions.
Disaster recovery options may include features such as off-site backups, replication of data across multiple locations, and failover systems. These measures help to ensure that businesses can quickly recover from any unforeseen events, such as natural disasters or cyber attacks.
Business continuity planning is equally important. A reliable data center hosting provider should have comprehensive plans and procedures in place to ensure that critical business operations can continue in the event of a disruption. Regular testing and maintenance of these plans should also be conducted to identify and address any potential weaknesses.
Backup and recovery solutions are another consideration. Businesses should ensure that their data center hosting partner offers robust backup and recovery options to protect against data loss. These solutions should be customizable to meet the specific needs of each business.
Cost-Effective Data Center Hosting Solutions for Small and Medium-Sized Businesses
Data center hosting solutions can be cost-effective for small and medium-sized businesses (SMBs) as they eliminate the need for significant capital investments in on-premises infrastructure. There are several cost-effective options available for SMBs.
Shared hosting options allow multiple businesses to share the same physical server, reducing costs. This is a suitable option for businesses with low traffic websites or basic hosting needs.
Virtual private servers (VPS) offer a higher level of performance and security compared to shared hosting. With VPS, businesses have their own virtual server within a shared physical server, providing more control and flexibility.
Cloud hosting is another cost-effective option for SMBs. Cloud hosting allows businesses to access computing resources on-demand, paying only for what they use. This eliminates the need for upfront investments in hardware and allows businesses to scale their infrastructure as needed.
Hybrid hosting combines the benefits of both on-premises infrastructure and cloud hosting. This allows businesses to leverage their existing infrastructure while also taking advantage of the scalability and flexibility offered by the cloud.
Managed Services and Support Offered by Data Center Hosting Providers
Managed services are an important consideration when choosing a data center hosting partner. Managed hosting services allow businesses to offload the day-to-day management and maintenance of their infrastructure to a specialized provider.
A reliable data center hosting provider should offer comprehensive managed services, including server management, network monitoring, security updates, and backups. This allows businesses to focus on their core competencies while leaving the technical aspects of infrastructure management to the experts.
Technical support is another important aspect to consider. A reputable data center hosting provider should offer 24/7 technical support to address any issues or concerns that may arise. This ensures that businesses have access to timely assistance whenever they need it.
Monitoring and management are also crucial. A reliable data center hosting provider should have robust monitoring systems in place to proactively identify and address any potential issues. This helps to minimize downtime and ensure optimal performance.
Professional services are another consideration. A reputable data center hosting provider should have a team of experienced professionals who can provide guidance and support in areas such as architecture design, migration planning, and optimization. These services can be particularly valuable for businesses with complex infrastructure requirements.
Cloud Computing and Virtualization Options for Data Center Hosting
Cloud computing and virtualization technologies are increasingly being used in data center hosting to provide greater flexibility and efficiency.
Cloud computing options allow businesses to access computing resources on-demand over the internet. This eliminates the need for upfront investments in hardware and allows businesses to scale their infrastructure as needed. Cloud computing also offers benefits such as high availability, automatic backups, and disaster recovery options.
Virtualization technologies allow businesses to run multiple virtual machines on a single physical server, maximizing resource utilization and reducing costs. Virtualization also provides greater flexibility and agility, allowing businesses to quickly provision or deprovision resources as needed.
Benefits of cloud and virtualization include improved scalability, reduced hardware costs, increased efficiency, and simplified management. These technologies also enable businesses to easily migrate workloads between different environments, such as on-premises infrastructure and the cloud.
Choosing the Right Data Center Hosting Partner: Best Practices and Recommendations
Choosing the right data center hosting partner is a critical decision that can have a significant impact on your business operations. To ensure a successful partnership, there are several best practices and recommendations to follow.
Firstly, it is important to assess your business needs and requirements. Consider factors such as the size of your business, the nature of your operations, and your growth projections. This will help you determine the specific features and capabilities you need from a data center hosting partner.
Next, evaluate potential partners based on factors such as location, reliability, customer support, reputation, and experience. Look for providers that have a proven track record in the industry and positive customer reviews. It is also advisable to visit the data center facility in person to assess its physical security measures and infrastructure.
When evaluating potential partners, ask the right questions to ensure that they can meet your specific needs. Inquire about their uptime guarantees, disaster recovery options, compliance certifications, and scalability options. It is also important to understand their pricing structure and any additional fees or charges that may apply.
Finally, make the final decision based on a combination of factors such as cost-effectiveness, reliability, security, scalability, and support. It is advisable to choose a data center hosting partner that aligns with your long-term business goals and can support your growth plans.
Conclusion: Recap of the importance of data center hosting and the key considerations when choosing a partner.
In conclusion, data center hosting is crucial for businesses of all sizes as it allows them to store, process, and manage their data in a reliable and secure manner. When choosing a data center hosting partner, businesses should consider factors such as location, reliability, customer support, reputation, and experience. Security measures and compliance requirements are also important considerations. Scalability and flexibility are key advantages of data center hosting solutions. Network connectivity and latency should be carefully evaluated to ensure optimal performance. Disaster recovery and business continuity planning are crucial aspects of data center hosting. Cost-effective solutions are available for small and medium-sized businesses. Managed services and support offered by data center hosting providers are important considerations. Cloud computing and virtualization technologies offer greater flexibility and efficiency. Choosing the right data center hosting partner requires assessing your business needs, evaluating potential partners, asking the right questions, and making the final decision based on a combination of factors.
If you’re interested in learning more about data center hosting and how to set up your own home data center, check out this comprehensive guide: “The Best Guide to Setting Up a Home Data Center.” This article provides valuable insights and practical tips for individuals looking to create their own data center environment. Whether you’re a small business owner or a tech enthusiast, this guide will help you navigate the complexities of building and managing your own data center. Don’t miss out on this informative resource!
FAQs
What is data center hosting?
Data center hosting refers to the practice of housing and maintaining servers, storage, and networking equipment in a secure and reliable facility. This allows businesses to outsource their IT infrastructure and focus on their core operations.
Why should businesses consider data center hosting?
Data center hosting offers several benefits, including improved security, reliability, scalability, and cost-effectiveness. By outsourcing their IT infrastructure, businesses can reduce their capital and operational expenses, while also gaining access to advanced technologies and expertise.
What factors should businesses consider when choosing a data center hosting partner?
When choosing a data center hosting partner, businesses should consider factors such as location, security, reliability, scalability, connectivity, support, and cost. They should also evaluate the provider’s track record, certifications, and compliance with industry standards and regulations.
What types of data center hosting services are available?
Data center hosting services can vary depending on the provider and the needs of the business. Some common types of services include colocation, managed hosting, cloud hosting, and hybrid hosting. Each type of service offers different levels of control, customization, and management.
What are the benefits of colocation?
Colocation allows businesses to house their own servers and equipment in a secure and reliable data center facility. This offers several benefits, including improved security, reliability, scalability, and cost-effectiveness. Colocation also allows businesses to retain full control over their IT infrastructure, while also gaining access to advanced technologies and expertise.
What are the benefits of managed hosting?
Managed hosting allows businesses to outsource the management and maintenance of their IT infrastructure to a third-party provider. This offers several benefits, including reduced operational expenses, improved reliability, scalability, and security. Managed hosting also allows businesses to focus on their core operations, while also gaining access to advanced technologies and expertise.
What are the benefits of cloud hosting?
Cloud hosting allows businesses to access computing resources and services over the internet, without the need for on-premises hardware or software. This offers several benefits, including improved scalability, flexibility, and cost-effectiveness. Cloud hosting also allows businesses to access advanced technologies and services, such as artificial intelligence, machine learning, and big data analytics.
What are the benefits of hybrid hosting?
Hybrid hosting allows businesses to combine multiple hosting solutions, such as colocation, managed hosting, and cloud hosting, to create a customized and flexible IT infrastructure. This offers several benefits, including improved scalability, reliability, and cost-effectiveness. Hybrid hosting also allows businesses to leverage the strengths of each hosting solution, while also addressing their specific needs and requirements.
The data center market refers to the industry that provides physical infrastructure and services for storing, processing, and managing large amounts of data. In the digital age, data centers play a crucial role in supporting the growing demand for data storage and processing capabilities. They are essential for businesses and organizations that rely on technology to store and analyze data, run applications, and deliver services to their customers.
Data centers have a long history that dates back to the early days of computing. In the 1960s, large mainframe computers required dedicated rooms with specialized cooling and power systems to operate. These rooms eventually evolved into what we now know as data centers. Over the years, data centers have become more sophisticated and efficient, with advancements in technology enabling higher densities of computing power in smaller spaces.
Key Takeaways
- The data center market is a rapidly growing industry that provides critical infrastructure for businesses and organizations.
- Key players in the data center market include Amazon Web Services, Microsoft, and Google, among others.
- Challenges in the data center market include rising energy costs, security concerns, and the need for constant upgrades and maintenance.
- Opportunities in the data center market include the adoption of emerging technologies such as artificial intelligence and the Internet of Things.
- The future outlook for the data center market is positive, with continued growth and innovation expected in the coming years.
Growth and Trends in the Data Center Market
The data center market is currently experiencing significant growth due to the increasing demand for digital services and the proliferation of connected devices. According to a report by MarketsandMarkets, the global data center market is expected to grow from $40.32 billion in 2017 to $77.18 billion by 2022, at a compound annual growth rate (CAGR) of 13.7%.
Several trends are driving this growth in the data center market. One of the key trends is the rise of cloud computing. Cloud computing allows businesses to access computing resources on-demand over the internet, eliminating the need for on-premises infrastructure. This has led to a shift from traditional data centers to cloud-based solutions, which offer scalability, flexibility, and cost savings.
Another trend driving growth in the data center market is the increasing adoption of big data analytics. As businesses collect and analyze large volumes of data, they require more storage and processing capabilities. Data centers provide the infrastructure needed to handle these massive amounts of data and enable real-time analytics.
Key Players in the Data Center Market
The data center market is highly competitive, with several major players dominating the industry. Some of the key players in the market include Amazon Web Services (AWS), Microsoft Azure, Google Cloud, IBM, and Oracle. These companies offer a range of services, including infrastructure-as-a-service (IaaS), platform-as-a-service (PaaS), and software-as-a-service (SaaS).
Amazon Web Services (AWS) is currently the largest player in the data center market, with a significant market share. AWS offers a wide range of cloud services, including compute power, storage, and databases. Microsoft Azure is another major player, providing a comprehensive suite of cloud services for businesses of all sizes. Google Cloud and IBM also have a significant presence in the market, offering cloud computing solutions and AI capabilities.
Challenges in the Data Center Market
While the data center market presents significant opportunities for growth, it also faces several challenges. One of the main challenges is rising energy costs. Data centers consume a large amount of electricity to power and cool their infrastructure. As energy prices continue to rise, data center operators are under pressure to find more energy-efficient solutions to reduce costs and minimize their environmental impact.
Another challenge in the data center market is related to infrastructure and design. Data centers require specialized facilities with redundant power supplies, cooling systems, and physical security measures. Designing and building these facilities can be complex and costly. Additionally, as technology advances and computing demands increase, data centers need to continually upgrade their infrastructure to keep up with the latest requirements.
Opportunities in the Data Center Market
Despite the challenges, there are several opportunities in the data center market that can drive growth and innovation. One of the key opportunities is the growth potential in emerging markets. As more countries around the world embrace digital transformation, there is a growing demand for data center services. Emerging markets such as India, China, and Brazil offer significant growth opportunities for data center operators.
Another opportunity in the data center market is related to cloud computing. As businesses increasingly adopt cloud-based solutions, there is a growing need for data centers to support these services. Cloud computing offers scalability, flexibility, and cost savings, making it an attractive option for businesses of all sizes. Data center operators can capitalize on this trend by offering cloud services and partnering with cloud providers.
The Impact of Cloud Computing on the Data Center Market
Cloud computing has had a significant impact on the data center market, transforming the way businesses store, process, and manage data. Cloud computing allows businesses to access computing resources on-demand over the internet, eliminating the need for on-premises infrastructure. This has led to a shift from traditional data centers to cloud-based solutions.
The growth of cloud computing has also had an impact on data center infrastructure. Traditional data centers were designed to support a specific workload and had limited scalability. With cloud computing, data centers need to be able to handle fluctuating workloads and scale up or down as needed. This has led to the development of hyper-scale data centers that can support massive amounts of computing power.
While cloud computing presents opportunities for data center operators, it also brings challenges. One of the main challenges is related to security and privacy. With data being stored and processed in the cloud, there is a need for robust security measures to protect sensitive information. Data center operators need to invest in advanced security technologies and implement strict access controls to ensure the confidentiality and integrity of their customers’ data.
Data Center Infrastructure and Design
Data center infrastructure refers to the physical components that make up a data center, including servers, storage devices, networking equipment, cooling systems, and power distribution units. Designing an efficient and reliable data center infrastructure is crucial for ensuring optimal performance and minimizing downtime.
Key components of data center infrastructure include:
– Servers: These are the computers that process and store data. Data centers typically have racks of servers that are connected to each other and to the network.
– Storage devices: These are used to store data, such as hard disk drives (HDDs) and solid-state drives (SSDs). Data centers often use redundant storage systems to ensure data availability.
– Networking equipment: This includes switches, routers, and firewalls that enable communication between servers and the outside world. Data centers need high-speed and reliable networking infrastructure to handle large amounts of data traffic.
– Cooling systems: Data centers generate a significant amount of heat, so cooling systems are essential to maintain optimal operating temperatures. This can include air conditioning units, liquid cooling systems, or even immersion cooling technologies.
– Power distribution units (PDUs): These devices distribute power to the servers and other equipment in the data center. Redundant power supplies and backup generators are often used to ensure uninterrupted power supply.
Best practices for data center design include:
– Redundancy: Data centers should have redundant systems in place to minimize the risk of downtime. This includes redundant power supplies, cooling systems, and network connections.
– Scalability: Data centers should be designed with scalability in mind, allowing for easy expansion as computing demands increase. This can include modular designs that can be easily expanded or upgraded.
– Energy efficiency: Data centers consume a significant amount of energy, so it is important to design for energy efficiency. This can include using energy-efficient hardware, implementing efficient cooling systems, and optimizing server utilization.
Security and Compliance in the Data Center Market
Security is a critical concern in the data center market, as data centers store and process sensitive information for businesses and organizations. Data center operators need to implement robust security measures to protect against unauthorized access, data breaches, and other security threats.
Data center security measures can include:
– Physical security: Data centers should have strict access controls, including biometric authentication, surveillance cameras, and security guards. Only authorized personnel should be allowed to enter the data center.
– Network security: Data centers should have firewalls, intrusion detection systems, and other network security measures in place to protect against cyber threats. Regular security audits and vulnerability assessments should also be conducted.
– Data encryption: Data should be encrypted both at rest and in transit to protect against unauthorized access. This can include using encryption algorithms and secure communication protocols.
Compliance regulations also play a significant role in the data center market. Many industries, such as healthcare and finance, have strict regulations regarding the storage and processing of data. Data center operators need to ensure that their facilities and processes comply with these regulations to avoid legal and financial consequences.
Emerging Technologies in the Data Center Market
The data center market is constantly evolving, driven by advancements in technology. Several emerging technologies are expected to have a significant impact on the data center market in the coming years.
One of these technologies is artificial intelligence (AI) and machine learning (ML). AI and ML can be used to optimize data center operations, improve energy efficiency, and enhance security. For example, AI algorithms can analyze data center performance metrics in real-time and make adjustments to optimize energy usage. ML algorithms can also be used to detect anomalies and potential security threats.
Another emerging technology in the data center market is edge computing. Edge computing brings computing power closer to the source of data generation, reducing latency and improving performance for applications that require real-time processing. This can include applications such as autonomous vehicles, smart cities, and industrial IoT.
Future Outlook for the Data Center Market
The future outlook for the data center market is promising, with continued growth expected in the coming years. The increasing demand for digital services, the proliferation of connected devices, and advancements in technology will continue to drive the growth of the data center market.
According to a report by Grand View Research, the global data center market is projected to reach $284.44 billion by 2028, growing at a CAGR of 7.7% from 2021 to 2028. This growth will be driven by factors such as the increasing adoption of cloud computing, the rise of big data analytics, and the need for scalable and secure data storage solutions.
However, data center operators will also face challenges in the future. Rising energy costs, increasing regulatory compliance requirements, and the need for continuous infrastructure upgrades will require data center operators to innovate and adapt to stay competitive.
In conclusion, the data center market plays a crucial role in supporting the growing demand for data storage and processing capabilities in the digital age. The market is currently experiencing significant growth, driven by trends such as cloud computing and big data analytics. While there are challenges in the market, there are also opportunities for growth and innovation. Data center operators need to stay ahead of emerging technologies and trends to capitalize on these opportunities and overcome the challenges they face.
If you’re interested in learning more about the future of computing, you should definitely check out this informative article on Understanding Edge Computing: The Future of Computing. It delves into the concept of edge computing and how it is revolutionizing the data center market. With the rise of IoT devices and the need for real-time processing, edge computing offers a decentralized approach that brings computation closer to the source of data generation. This article explores the benefits, challenges, and opportunities associated with this emerging trend.
FAQs
What is the data center market?
The data center market refers to the industry that provides physical space, power, and cooling for the storage and processing of digital data.
What are the current trends in the data center market?
Current trends in the data center market include the adoption of cloud computing, the use of artificial intelligence and machine learning, the growth of edge computing, and the increasing demand for renewable energy sources.
What are the challenges facing the data center market?
Challenges facing the data center market include the high cost of building and maintaining data centers, the need for skilled personnel to manage and operate them, and the increasing demand for energy-efficient and sustainable solutions.
What are the opportunities in the data center market?
Opportunities in the data center market include the potential for growth in emerging markets, the development of new technologies and services, and the increasing demand for data storage and processing capabilities.
What is the impact of cloud computing on the data center market?
Cloud computing has had a significant impact on the data center market, as it has led to the consolidation of data center infrastructure and the growth of hyperscale data centers. It has also increased the demand for data center services and created new opportunities for data center providers.
What is edge computing and how is it affecting the data center market?
Edge computing refers to the processing of data at or near the source of the data, rather than in a centralized data center. This trend is affecting the data center market by creating new opportunities for smaller, more distributed data centers that can support edge computing applications.
Auto data processing, or automatic data processing, is the use of computerized systems and software to handle large volumes of data efficiently. This process involves utilizing computers and electronic devices for data input, storage, manipulation, and output. It is crucial for organizations that regularly manage substantial amounts of information, such as financial institutions, healthcare providers, and retail businesses.
The auto data processing workflow begins with raw data input into a computer system from various sources, including customer transactions, inventory records, and employee information. The data is then processed using algorithms and software programs to organize, analyze, and interpret the information. Finally, the processed data is outputted in a format suitable for decision-making and reporting purposes.
This automated approach is essential for businesses to optimize their operations and make informed decisions based on accurate, current information. By automating data processing tasks, organizations can reduce time and resource expenditure, minimize human error risks, and enhance overall operational efficiency.
Key Takeaways
- Auto data processing involves the use of automated systems to collect, process, and analyze data for businesses.
- The benefits of auto data processing for businesses include increased efficiency, accuracy, and cost savings.
- When choosing the right auto data processing software, businesses should consider factors such as scalability, integration capabilities, and security features.
- Implementing auto data processing in your business requires careful planning, training, and ongoing maintenance to ensure success.
- Best practices for auto data processing include regular data backups, data validation, and compliance with data privacy regulations.
The Benefits of Auto Data Processing for Businesses
Increased Productivity and Efficiency
One of the primary benefits is the ability to process large volumes of data quickly and accurately. With automated systems in place, businesses can handle massive amounts of data without the need for manual intervention, which can significantly speed up the processing time and improve overall productivity.
Reduced Human Error
Another key benefit of auto data processing is the reduction of human error. Manual data processing is prone to errors, such as typos, miscalculations, and misinterpretations. By automating the data processing tasks, businesses can minimize the risk of errors and ensure that the processed data is accurate and reliable.
Real-Time Insights and Cost Savings
Furthermore, auto data processing allows businesses to generate real-time insights and reports based on the processed data. This enables decision-makers to have access to up-to-date information, which can be crucial for making informed decisions and responding quickly to changing market conditions. In addition, auto data processing can help businesses save costs by reducing the need for manual labor and streamlining their operations. By automating repetitive tasks, businesses can allocate their resources more efficiently and focus on value-added activities that contribute to their bottom line.
Choosing the Right Auto Data Processing Software
When it comes to choosing the right auto data processing software for your business, there are several factors to consider. First and foremost, it’s important to assess your business needs and objectives to determine the specific features and functionalities that are essential for your operations. For example, if your business deals with complex financial data, you may require software that offers advanced analytics and reporting capabilities.
It’s also crucial to consider the scalability of the software. As your business grows, you’ll need a data processing solution that can accommodate increasing volumes of data without compromising performance. Look for software that offers scalability options and can adapt to your evolving business needs.
Another important factor to consider is the integration capabilities of the software. Ideally, you’ll want a data processing solution that seamlessly integrates with your existing systems and workflows. This will ensure a smooth transition and minimize disruptions to your operations.
Additionally, consider the user-friendliness of the software. Look for a solution that is intuitive and easy to use, as this will facilitate adoption among your employees and minimize the need for extensive training. Lastly, consider the security features of the software.
Data security is paramount in today’s business environment, so it’s essential to choose a solution that offers robust security measures to protect your sensitive information from unauthorized access and cyber threats.
How to Implement Auto Data Processing in Your Business
Implementing auto data processing in your business requires careful planning and execution to ensure a smooth transition and maximize the benefits of automation. The first step is to assess your current data processing workflows and identify areas that can be automated for improved efficiency. This may involve conducting a thorough analysis of your existing processes and identifying bottlenecks or areas for improvement.
Once you have identified the areas for automation, it’s important to select the right auto data processing software that aligns with your business needs and objectives. Consider factors such as scalability, integration capabilities, user-friendliness, and security features when evaluating potential solutions. Next, develop a comprehensive implementation plan that outlines the steps involved in deploying the auto data processing software.
This plan should include a timeline for implementation, resource allocation, training requirements, and contingency measures to address any potential challenges or issues that may arise during the implementation process. It’s also crucial to involve key stakeholders in the implementation process to ensure buy-in and support from all levels of the organization. Communicate the benefits of auto data processing and provide training and support to employees to facilitate a smooth transition to the new system.
Finally, monitor the implementation process closely and gather feedback from users to identify any areas for improvement or optimization. Continuous evaluation and refinement of the auto data processing system will help ensure that it continues to meet the evolving needs of your business.
Best Practices for Auto Data Processing
To maximize the benefits of auto data processing in your business, it’s important to follow best practices that promote efficiency, accuracy, and security. One best practice is to establish clear data processing standards and protocols to ensure consistency and accuracy in the processed data. This may involve defining data entry guidelines, validation rules, and quality control measures to maintain high standards of data integrity.
Another best practice is to regularly monitor and audit the auto data processing system to identify any anomalies or discrepancies in the processed data. Implementing regular audits will help detect errors or inconsistencies early on and prevent them from impacting critical business decisions. It’s also important to establish robust security measures to protect your processed data from unauthorized access or cyber threats.
This may involve implementing encryption protocols, access controls, and regular security assessments to safeguard your sensitive information. Furthermore, it’s essential to stay updated with the latest advancements in auto data processing technology and continuously evaluate new software solutions that may offer improved features or capabilities for your business. Lastly, fostering a culture of continuous improvement and innovation within your organization will help drive ongoing optimization of your auto data processing workflows and ensure that you are leveraging the full potential of automation for your business operations.
Overcoming Common Challenges in Auto Data Processing
Data Accuracy and Integrity
One of the primary challenges is ensuring the accuracy and integrity of data throughout the processing workflow. To overcome this, businesses should implement robust validation checks and quality control measures to detect and correct errors in the processed data.
Managing Large Volumes of Data
Another challenge is managing large volumes of data efficiently without compromising performance. Businesses can address this by investing in scalable auto data processing solutions that can handle increasing volumes of data without sacrificing speed or reliability.
Data Security and Integration
Data security is another critical challenge in auto data processing. To mitigate this, businesses should prioritize implementing strong security measures, such as encryption, access controls, and regular security assessments to protect their processed data from unauthorized access or cyber threats. Additionally, ensuring seamless integration with existing systems and workflows can be a challenge when implementing auto data processing. Businesses should carefully evaluate potential solutions for their integration capabilities and work closely with their IT teams to ensure a smooth transition and minimal disruptions to their operations.
User Adoption and Training
Lastly, user adoption and training can be a challenge when introducing new auto data processing systems. To overcome this, businesses should provide comprehensive training and support to employees to facilitate a smooth transition to the new system and ensure that they are equipped with the necessary skills to leverage the automation effectively.
Tips for Maximizing the Potential of Auto Data Processing
To maximize the potential of auto data processing in your business, consider implementing the following tips: 1. Leverage advanced analytics: Explore advanced analytics capabilities offered by auto data processing software to gain deeper insights into your processed data and make informed decisions based on real-time analytics. 2. Embrace automation: Look for opportunities to automate repetitive tasks beyond just data processing, such as report generation, notifications, and alerts, to further streamline your operations. 3. Foster a culture of data-driven decision-making: Encourage decision-makers within your organization to rely on processed data for making strategic decisions rather than relying solely on intuition or past experiences. 4. Stay updated with industry trends: Keep abreast of industry trends in auto data processing technology and continuously evaluate new solutions that may offer improved features or capabilities for your business. 5. Collaborate with IT teams: Work closely with your IT teams to ensure that your auto data processing systems are aligned with your overall IT infrastructure and security protocols. By following these tips, businesses can unlock the full potential of auto data processing and drive greater efficiency, accuracy, and innovation within their operations.
If you’re interested in learning more about creating a secure and HIPAA-compliant data center, check out this article on tips for success. It provides valuable insights into the best practices for ensuring data security and compliance in a data center environment.
FAQs
What is auto data processing?
Auto data processing refers to the use of automated systems and software to process and analyze large volumes of data related to automobiles. This can include data from vehicle sensors, GPS systems, maintenance records, and more.
Why is auto data processing important?
Auto data processing is important because it allows for the efficient analysis of large volumes of data, which can provide valuable insights for vehicle manufacturers, fleet operators, and other stakeholders in the automotive industry. This can lead to improvements in vehicle performance, safety, and efficiency.
What are some common applications of auto data processing?
Some common applications of auto data processing include predictive maintenance, vehicle performance monitoring, fuel efficiency analysis, and driver behavior analysis. Auto data processing can also be used for telematics, fleet management, and connected car services.
What are the benefits of auto data processing?
The benefits of auto data processing include improved vehicle performance and safety, reduced maintenance costs, optimized fuel efficiency, and better decision-making for fleet management and logistics. Auto data processing can also enable the development of new automotive technologies and services.
What are some challenges associated with auto data processing?
Challenges associated with auto data processing include data security and privacy concerns, the need for advanced analytics and machine learning capabilities, and the integration of data from diverse sources such as vehicles, infrastructure, and external systems. Additionally, managing and processing large volumes of real-time data can be a technical challenge.
Auto data processing is the utilization of automated systems and software to gather, process, and analyze automotive industry-related data. This encompasses information on vehicle performance, maintenance, sales, customer preferences, and other relevant aspects. The primary objectives of auto data processing are to optimize operations, enhance decision-making processes, and improve overall efficiency within the automotive sector.
By employing advanced technologies such as artificial intelligence, machine learning, and big data analytics, auto data processing enables companies to extract valuable insights and make informed business decisions. The process involves various tools and techniques to manage large volumes of data generated by vehicles, customers, and other sources. This includes data collection through sensors and Internet of Things (IoT) devices, storage in cloud-based platforms, processing using algorithms and software applications, and analysis to identify meaningful patterns and trends.
As modern vehicles become increasingly complex and interconnected, auto data processing has emerged as a crucial component of the automotive industry, driving innovation and creating new opportunities for growth and development.
Key Takeaways
- Auto data processing involves the use of computer systems to process and analyze data related to automobiles and automotive operations.
- Auto data processing is important for improving efficiency, accuracy, and decision-making in the automotive industry.
- Auto data processing works by collecting, organizing, and analyzing data from various sources such as sensors, GPS, and vehicle diagnostics.
- Auto data processing plays a crucial role in optimizing production, supply chain management, and customer experience in the automotive industry.
- The benefits of auto data processing include improved safety, predictive maintenance, cost savings, and enhanced customer satisfaction.
The Importance of Auto Data Processing
Improving Vehicle Performance and Customer Experiences
By collecting and analyzing data from vehicles, manufacturers can gain valuable insights into usage patterns, maintenance needs, and potential issues. This enables them to design better products and provide more effective support services. Similarly, auto data processing allows dealerships and service centers to offer personalized solutions and proactive maintenance, leading to higher customer satisfaction and loyalty.
Understanding Market Trends and Competitive Dynamics
Auto data processing is essential for understanding market trends, consumer preferences, and competitive dynamics within the automotive sector. By analyzing sales data, customer feedback, and industry benchmarks, companies can identify opportunities for innovation, optimize their product offerings, and develop targeted marketing strategies. This not only helps businesses stay competitive in a rapidly evolving market but also fosters a culture of continuous improvement and customer-centricity.
Driving Efficiency, Innovation, and Sustainable Growth
Overall, auto data processing is a cornerstone of modern automotive operations, driving efficiency, innovation, and sustainable growth. By leveraging the power of data, companies can stay ahead of the curve, improve their bottom line, and build strong relationships with their customers.
How Auto Data Processing Works
Auto data processing involves a series of interconnected steps that enable the collection, storage, processing, and analysis of large volumes of data from various sources within the automotive industry. The process begins with the collection of data from vehicles, sensors, customer interactions, and other relevant touchpoints. This data is then transmitted to centralized databases or cloud-based platforms where it is stored securely and made accessible for further processing.
Once the data is stored, it undergoes various processing techniques such as cleaning, transformation, and enrichment to ensure its accuracy and relevance. After processing, the data is analyzed using advanced algorithms and software applications to identify patterns, trends, and insights that can inform decision-making and drive business outcomes. This analysis may involve techniques such as machine learning, predictive modeling, and statistical analysis to uncover hidden correlations and make accurate predictions.
The results of the analysis are then used to inform strategic decisions, optimize operations, and drive innovation within the automotive industry. Overall, auto data processing works by leveraging technology to turn raw data into actionable insights that can be used to improve products, services, and customer experiences.
The Role of Auto Data Processing in the Automotive Industry
Auto data processing plays a critical role in shaping the future of the automotive industry by enabling companies to harness the power of data for innovation, efficiency, and customer satisfaction. In vehicle manufacturing, auto data processing is used to monitor production processes, optimize supply chain management, and improve quality control. This ensures that vehicles are built to high standards and meet customer expectations for performance and reliability.
In addition, auto data processing is essential for developing connected and autonomous vehicles that rely on real-time data to operate safely and efficiently. Furthermore, in the aftermarket segment of the automotive industry, auto data processing is used to support vehicle maintenance, diagnostics, and repair services. By collecting and analyzing data from vehicles in real-time, service providers can proactively identify issues, recommend maintenance actions, and deliver personalized solutions to customers.
This not only improves the overall ownership experience but also helps extend the lifespan of vehicles and reduce operational costs for both customers and businesses. Overall, auto data processing is a driving force behind the transformation of the automotive industry towards a more connected, intelligent, and customer-centric ecosystem.
Benefits of Auto Data Processing
Auto data processing offers a wide range of benefits for companies operating within the automotive industry. One of the key advantages is the ability to gain valuable insights from large volumes of data that can inform strategic decision-making and drive business outcomes. By analyzing vehicle performance data, customer feedback, market trends, and other relevant information, companies can identify opportunities for innovation, optimize their operations, and develop targeted strategies that resonate with their target audience.
This leads to improved competitiveness, increased market share, and sustainable growth over time. Another benefit of auto data processing is its ability to enhance operational efficiency within the automotive sector. By automating processes such as inventory management, supply chain optimization, predictive maintenance, and customer relationship management, companies can reduce manual effort, minimize errors, and streamline their operations for better performance.
This not only leads to cost savings but also enables businesses to focus on value-added activities that drive innovation and customer satisfaction. Additionally, auto data processing enables companies to offer personalized products and services that cater to individual customer needs and preferences, leading to higher satisfaction and loyalty.
Challenges and Limitations of Auto Data Processing
Data Privacy and Security Concerns
As vehicles become increasingly connected and reliant on data-driven technologies, the risk of unauthorized access, data breaches, and misuse of personal information grows. To mitigate these risks, companies must invest in robust cybersecurity measures and compliance frameworks to protect sensitive data and maintain customer trust.
Managing Complex Data
The automotive ecosystem generates vast amounts of diverse data from multiple sources, making it challenging to manage. Companies need to invest in scalable infrastructure, advanced analytics capabilities, and skilled talent to handle the complexity of auto data processing effectively.
Compliance and Ethical Considerations
Companies must ensure compliance with regulations such as GDPR and CCPA when collecting and processing customer data to avoid legal repercussions. A strategic approach that prioritizes data governance, risk management, and ethical use of data is essential to address these challenges within the automotive industry.
Future Trends in Auto Data Processing
The future of auto data processing in the automotive industry is poised for significant advancements driven by emerging technologies such as 5G connectivity, edge computing, and artificial intelligence. These technologies will enable faster data transmission, real-time analytics, and intelligent decision-making capabilities that can revolutionize how vehicles are designed, manufactured, operated, and serviced. For example, 5G connectivity will enable vehicles to exchange large volumes of data with each other and with infrastructure in real-time, paving the way for safer autonomous driving experiences and enhanced vehicle-to-everything (V2X) communication.
Furthermore, edge computing will enable vehicles to process data locally without relying on centralized servers or cloud platforms. This will reduce latency in decision-making processes and enable faster response times for critical applications such as collision avoidance systems or predictive maintenance alerts. Additionally, artificial intelligence will play a key role in auto data processing by enabling vehicles to learn from their environment, adapt to changing conditions, and personalize experiences for drivers and passengers.
Overall, these future trends in auto data processing will drive new opportunities for innovation within the automotive industry while also raising new challenges related to data governance, privacy protection, and ethical use of AI-powered technologies. In conclusion, auto data processing is a critical component of the modern automotive industry that enables companies to collect, process, analyze large volumes of data from vehicles customers other sources in order to drive innovation efficiency customer satisfaction over time. By leveraging advanced technologies such as artificial intelligence machine learning big data analytics auto data processing enables companies gain valuable insights make informed business decisions within the automotive sector.
Auto data processing involves series interconnected steps that enable collection storage processing analysis large volumes of data from various sources within the automotive industry. The process begins with collection of data from vehicles sensors customer interactions other relevant touchpoints. This data then transmitted centralized databases or cloud-based platforms where it stored securely made accessible further processing.
Once the data stored undergoes various processing techniques such as cleaning transformation enrichment ensure its accuracy relevance. After processing the data analyzed using advanced algorithms software applications identify patterns trends insights that can inform decision-making drive business outcomes. The results analysis then used inform strategic decisions optimize operations drive innovation within the automotive industry.
Auto data processing plays critical role shaping future automotive industry by enabling companies harness power data innovation efficiency customer satisfaction. In vehicle manufacturing auto data processing used monitor production processes optimize supply chain management improve quality control. This ensures that vehicles built high standards meet customer expectations performance reliability.
In addition auto data processing essential developing connected autonomous vehicles that rely real-time data operate safely efficiently. Furthermore aftermarket segment automotive industry auto data processing used support vehicle maintenance diagnostics repair services. By collecting analyzing data from vehicles real-time service providers can proactively identify issues recommend maintenance actions deliver personalized solutions customers.
This not only improves overall ownership experience but also helps extend lifespan vehicles reduce operational costs both customers businesses. Overall auto data processing driving force behind transformation automotive industry towards more connected intelligent customer-centric ecosystem. Auto data processing offers wide range benefits companies operating within automotive industry.
One key advantages ability gain valuable insights large volumes data can inform strategic decision-making drive business outcomes. By analyzing vehicle performance data customer feedback market trends other relevant information companies can identify opportunities innovation optimize their operations develop targeted strategies resonate with their target audience. This leads improved competitiveness increased market share sustainable growth over time.
Another benefit auto data processing ability enhance operational efficiency within automotive sector. By automating processes inventory management supply chain optimization predictive maintenance customer relationship management companies can reduce manual effort minimize errors streamline their operations better performance. This not only leads cost savings but also enables businesses focus on value-added activities drive innovation customer satisfaction.
Additionally auto data processing enables companies offer personalized products services cater individual customer needs preferences leading higher satisfaction loyalty. While auto data processing offers numerous benefits automotive industry also presents several challenges limitations companies need address order maximize its potential. One main challenges related data privacy security concerns.
As vehicles become more connected reliant on data-driven technologies there increased risk unauthorized access breaches misuse personal information. Companies need invest robust cybersecurity measures compliance frameworks protect sensitive data ensure trust among customers. Another challenge related complexity managing large volumes diverse data from multiple sources within automotive ecosystem.
This requires companies invest scalable infrastructure advanced analytics capabilities skilled talent handle complexity auto data processing effectively. Additionally companies need ensure they comply with relevant regulations such as GDPR CCPA when collecting processing customer data avoid legal repercussions. Overall addressing these challenges requires strategic approach prioritizes data governance risk management ethical use within automotive industry.
The future auto data processing automotive industry poised significant advancements driven emerging technologies such 5G connectivity edge computing artificial intelligence. These technologies will enable faster transmission real-time analytics intelligent decision-making capabilities can revolutionize how vehicles are designed manufactured operated serviced. For example 5G connectivity will enable vehicles exchange large volumes with each other with infrastructure real-time paving way safer autonomous driving experiences enhanced vehicle-to-everything (V2X) communication.
Furthermore edge computing will enable vehicles process locally without relying centralized servers cloud platforms. This will reduce latency decision-making processes enable faster response times critical applications collision avoidance systems predictive maintenance alerts. Additionally artificial intelligence will play key role auto data processing enabling vehicles learn from their environment adapt changing conditions personalize experiences drivers passengers.
Overall these future trends auto data processing will drive new opportunities innovation within automotive industry while also raising new challenges related governance privacy protection ethical use AI-powered technologies
If you’re interested in learning more about how data processing can be streamlined in the cloud, check out this article on Talend AWS. This article provides valuable insights into how Talend can help businesses get started with cloud computing in minutes, making data processing more efficient and effective.
FAQs
What is auto data processing?
Auto data processing refers to the use of automated systems and software to process and manage large volumes of data related to automobiles. This can include data related to vehicle performance, maintenance, sales, and customer information.
How does auto data processing work?
Auto data processing works by using computer systems and software to collect, store, and analyze data related to automobiles. This can include data from sensors within the vehicle, customer information from sales and service interactions, and historical data related to vehicle performance and maintenance.
Why does auto data processing matter?
Auto data processing is important because it allows for the efficient management and analysis of large volumes of data related to automobiles. This can lead to improved vehicle performance, better customer service, and more effective business operations for companies in the automotive industry.
In today’s digital age, data centers play a crucial role in storing, processing, and managing vast amounts of information. With the increasing reliance on technology and the growing demand for uninterrupted access to data, it is essential for data centers to prioritize redundancy in their infrastructure. Redundancy refers to the duplication of critical components and systems within a data center to ensure continuous operation in the event of a failure. This article will explore the importance of redundancy in data centers, the risks associated with single points of failure, different types of redundancy, the difference between redundancy and resilience, factors to consider when implementing redundancy, best practices for redundancy in data center architecture, the impact of redundancy on availability and uptime, the cost of redundancy, its role in disaster recovery and business continuity planning, future trends in redundancy and data center architecture, and conclude with final thoughts on the future of data center redundancy and architecture.
Key Takeaways
- Redundancy is crucial for ensuring high availability and uptime in data centers.
- Single points of failure in data center architecture can lead to costly downtime and data loss.
- Types of redundancy in data center design include power, cooling, network, and server redundancy.
- Resilience refers to a system’s ability to recover from failures, while redundancy focuses on preventing failures from occurring.
- Factors to consider when implementing redundancy include cost, complexity, and scalability.
- Best practices for redundancy in data center architecture include regular testing and maintenance, as well as monitoring and alerting systems.
- Redundancy can significantly improve data center availability and uptime, but it comes at a cost that must be balanced with risk mitigation.
- Redundancy plays a critical role in disaster recovery and business continuity planning.
- Future trends in redundancy and data center architecture include increased use of automation and artificial intelligence to improve efficiency and reduce downtime.
Understanding the Importance of Redundancy in Data Centers
Redundancy is a critical aspect of data center design and operation. It involves duplicating critical components and systems to ensure that if one fails, there is another ready to take its place without causing any disruption or downtime. Redundancy is important because it provides a safety net against failures that can occur due to various reasons such as hardware malfunctions, power outages, network failures, or natural disasters.
The consequences of not having redundancy in data centers can be severe. Without redundancy, a single point of failure can bring down an entire system or network, resulting in downtime and loss of productivity. This can have significant financial implications for businesses that rely on their data centers for their operations. Additionally, without redundancy, there is a higher risk of data loss or corruption if a failure occurs during critical operations such as data replication or backup.
The Risks of Single Points of Failure in Data Center Architecture
A single point of failure refers to a component or system within a data center that, if it fails, can cause the entire system to fail. Single points of failure pose significant risks to data center architecture as they can lead to downtime, loss of data, and disruption of services. Examples of single points of failure in data centers include a single power source, a single network connection, or a single cooling system.
The risks associated with single points of failure in data center architecture are numerous. If a data center relies on a single power source and that source fails, the entire system will go down. This can result in loss of data, interruption of services, and damage to the reputation of the organization. Similarly, if there is only one network connection and it fails, users will be unable to access the data center’s services. This can lead to customer dissatisfaction and loss of business.
Types of Redundancy in Data Center Design
There are several types of redundancy that can be implemented in data center design to ensure continuous operation and minimize the risks associated with single points of failure.
N+1 redundancy is a common approach where there is one additional backup component for every critical component in the system. For example, if a data center has four servers, it would have five power supplies – one for each server and an additional backup power supply.
2N redundancy takes redundancy a step further by having a complete duplicate of all critical components and systems. In this approach, there are two separate systems running in parallel, each capable of handling the full load. This provides an extra layer of protection against failures.
N+N redundancy is similar to 2N redundancy but with a more scalable approach. It involves having multiple redundant components or systems that can handle the load individually but can also work together to handle increased demand.
Active-active redundancy involves distributing the workload across multiple active systems simultaneously. This approach ensures that even if one system fails, the others can continue to handle the load without interruption.
Redundancy vs. Resilience: What’s the Difference?
While redundancy and resilience are often used interchangeably, they are not the same thing. Redundancy refers to the duplication of critical components and systems to ensure continuous operation in the event of a failure. Resilience, on the other hand, refers to the ability of a system to recover quickly from failures and continue operating without interruption.
Both redundancy and resilience are important in data center design. Redundancy provides a safety net against failures, while resilience ensures that even if a failure occurs, the system can quickly recover and continue operating. It is important to strike a balance between redundancy and resilience to ensure maximum uptime and availability.
Factors to Consider When Implementing Redundancy in Data Center Infrastructure
When implementing redundancy in data center infrastructure, there are several factors that need to be considered:
1. Cost: Redundancy can be expensive to implement, especially if it involves duplicating critical components and systems. Organizations need to weigh the cost of redundancy against the potential cost of downtime and loss of productivity.
2. Scalability: Data centers need to be able to scale their redundancy as their operations grow. It is important to consider how easily redundancy can be expanded or upgraded as the demand for services increases.
3. Maintenance: Redundant systems require regular maintenance to ensure they are functioning properly. Organizations need to factor in the cost and effort required for maintaining redundant components and systems.
4. Complexity: Implementing redundancy can add complexity to data center architecture. It is important to consider how redundancy will impact the overall complexity of the system and whether it will introduce any additional risks or challenges.
5. Availability requirements: Different organizations have different availability requirements. Some may require 24/7 uptime, while others may have more flexibility. It is important to align redundancy efforts with the specific availability requirements of the organization.
Best Practices for Redundancy in Data Center Architecture
To ensure the effectiveness of redundancy in data center architecture, it is important to follow best practices:
1. Conducting a risk assessment: Before implementing redundancy, organizations should conduct a thorough risk assessment to identify potential single points of failure and assess the impact of failures on the system.
2. Implementing a redundancy plan: Once the risks have been identified, a redundancy plan should be developed and implemented. This plan should outline the specific redundancy measures to be taken and how they will be implemented.
3. Regularly testing and maintaining redundancy systems: Redundant systems should be regularly tested to ensure they are functioning properly. Additionally, regular maintenance should be performed to identify and address any issues before they become critical failures.
4. Documenting redundancy procedures: It is important to document all redundancy procedures and processes to ensure consistency and ease of maintenance. This documentation should include step-by-step instructions for implementing redundancy measures and recovering from failures.
The Impact of Redundancy on Data Center Availability and Uptime
Redundancy plays a crucial role in ensuring data center availability and uptime. By duplicating critical components and systems, redundancy provides a safety net against failures that can cause downtime or interruption of services. With redundant systems in place, if one component or system fails, another can seamlessly take over without any disruption to operations.
The importance of measuring and monitoring data center availability and uptime cannot be overstated. By regularly monitoring these metrics, organizations can identify any potential issues or bottlenecks that may impact availability and take proactive measures to address them. Redundancy is a key factor in achieving high availability and uptime, but it is not the only factor. Other factors such as network reliability, power supply stability, and cooling efficiency also play a role.
The Cost of Redundancy: Balancing Investment and Risk Mitigation
Implementing redundancy in data centers can be costly, especially if it involves duplicating critical components and systems. Organizations need to carefully balance the cost of redundancy with the potential cost of downtime and loss of productivity. While redundancy can be expensive, the cost of downtime can be even higher, especially for organizations that rely heavily on their data centers for their operations.
To strike a balance between investment and risk mitigation, organizations should conduct a cost-benefit analysis. This analysis should consider the potential impact of downtime on the organization’s operations, reputation, and bottom line. It should also take into account the likelihood of failures and the potential cost savings that can be achieved through redundancy.
The Role of Redundancy in Disaster Recovery and Business Continuity Planning
Redundancy plays a crucial role in disaster recovery and business continuity planning. In the event of a disaster or major failure, redundant systems can ensure that critical operations can continue without interruption. By duplicating critical components and systems, organizations can minimize the impact of disasters and quickly recover from failures.
Redundancy is particularly important in disaster recovery scenarios where data centers may be affected by natural disasters such as earthquakes, floods, or hurricanes. By having redundant systems in geographically diverse locations, organizations can ensure that even if one data center is affected, operations can continue from another location.
Future Trends in Redundancy and Data Center Architecture
The future of data center redundancy and architecture is likely to be shaped by emerging technologies such as artificial intelligence (AI) and machine learning (ML). These technologies have the potential to revolutionize data center operations by enabling predictive maintenance, automated failover, and intelligent load balancing.
AI and ML can help data centers become more proactive in identifying potential failures before they occur. By analyzing vast amounts of data in real-time, AI and ML algorithms can detect patterns and anomalies that may indicate an impending failure. This can enable data center operators to take proactive measures to prevent failures and minimize downtime.
The future of data center architecture is also likely to be influenced by the increasing demand for cloud computing and edge computing. As more organizations move their operations to the cloud and rely on edge computing for real-time processing, data centers will need to adapt their redundancy strategies to ensure continuous operation and high availability.
In conclusion, redundancy is a critical aspect of data center design and operation. It ensures continuous operation in the event of failures and minimizes the risks associated with single points of failure. Redundancy can be achieved through various approaches such as N+1 redundancy, 2N redundancy, N+N redundancy, and active-active redundancy.
While redundancy is important, it is not the only factor to consider in data center design. Resilience, which refers to the ability of a system to recover quickly from failures, is also crucial. Both redundancy and resilience need to be balanced to ensure maximum uptime and availability.
Implementing redundancy in data centers can be costly, but the potential cost of downtime and loss of productivity can be even higher. Organizations need to carefully balance the cost of redundancy with the potential risks and benefits. By following best practices for redundancy in data center architecture, regularly testing and maintaining redundancy systems, and documenting redundancy procedures, organizations can ensure the effectiveness of their redundancy measures.
The future of data center redundancy and architecture is likely to be shaped by emerging technologies such as AI and ML. These technologies have the potential to revolutionize data center operations by enabling predictive maintenance, automated failover, and intelligent load balancing. As more organizations move their operations to the cloud and rely on edge computing, data centers will need to adapt their redundancy strategies to ensure continuous operation and high availability.
If you’re interested in learning more about data center architecture and the importance of data storage, you might find this article on “Unlocking the Power of RPP Data Centers: Data Storage That’s Faster, Smarter, and More Secure” informative. It delves into the concept of redundant power paths (RPP) and how they enhance the performance, reliability, and security of data centers. Check it out here.
Data centers are the backbone of modern technology, housing the servers and equipment that power our digital world. With the increasing demand for data storage and processing, data centers are growing in size and complexity. One critical aspect of data center operations is temperature control. The heat generated by the servers and equipment can cause significant damage if not properly managed. This is where cooling solutions come into play.
There are several types of cooling solutions available for data centers, each with its own advantages and disadvantages. The most common types include air-cooled systems, liquid-cooled systems, precision air conditioning systems, and hot aisle/cold aisle containment systems. These solutions aim to maintain an optimal temperature range within the data center to ensure the reliable operation of the equipment.
Key Takeaways
- Data center cooling solutions are essential for maintaining optimal temperatures and preventing equipment failure.
- Temperature control is crucial in data centers to ensure efficient operation and prevent downtime.
- Common cooling architectures include perimeter cooling, in-row cooling, and overhead cooling.
- Liquid-cooled solutions offer higher efficiency and lower energy costs, but air-cooled solutions are more common and easier to install.
- Precision air conditioning systems provide precise temperature and humidity control for sensitive equipment.
The Importance of Temperature Control in Data Centers
Temperature control is crucial in data centers because it directly impacts the performance and reliability of the equipment. Servers and other IT equipment generate a significant amount of heat during operation. If this heat is not effectively removed, it can lead to overheating, which can cause system failures, downtime, and even permanent damage to the equipment.
Inadequate temperature control can have severe consequences for data center operations. High temperatures can lead to increased energy consumption as cooling systems work harder to maintain a stable environment. This not only increases operational costs but also puts a strain on the cooling infrastructure, potentially leading to system failures.
Furthermore, high temperatures can shorten the lifespan of IT equipment, leading to more frequent replacements and higher capital expenditures. It can also result in reduced performance and slower processing speeds, negatively impacting the overall efficiency of the data center.
Common Cooling Architectures for Data Centers
There are several cooling architectures commonly used in data centers, each with its own advantages and disadvantages. The most common architectures include raised floor cooling, overhead cooling, and in-row cooling.
Raised floor cooling is a traditional approach where cool air is supplied through the raised floor and distributed to the equipment via perforated tiles. The hot air is then expelled through the ceiling or returned to the cooling units for recooling. This architecture provides flexibility in terms of equipment placement and airflow management. However, it can be less efficient in terms of cooling capacity and airflow distribution.
Overhead cooling, on the other hand, involves supplying cool air from overhead ducts and returning hot air through a separate duct system. This architecture eliminates the need for a raised floor, allowing for more efficient airflow distribution. However, it may require additional structural modifications to accommodate the overhead ducts.
In-row cooling is a more recent approach that involves placing cooling units directly next to the IT equipment in rows. This architecture provides precise cooling control and eliminates the need for extensive ductwork. However, it may require more floor space and can be more expensive to implement.
Air-Cooled vs. Liquid-Cooled Data Center Cooling Solutions
When it comes to data center cooling solutions, there are two main options: air-cooled and liquid-cooled systems. Air-cooled systems use fans and heat exchangers to remove heat from the data center environment, while liquid-cooled systems use water or other coolants to absorb and dissipate heat.
Air-cooled systems are the most common and cost-effective solution for data centers. They are relatively easy to install and maintain, requiring minimal infrastructure modifications. However, they may not be suitable for high-density data centers or environments with limited space.
Liquid-cooled systems, on the other hand, offer higher cooling capacities and greater energy efficiency. They can handle higher heat loads and provide more precise temperature control. However, they require additional infrastructure, such as piping and coolant distribution systems, which can increase installation and maintenance costs.
When choosing between air-cooled and liquid-cooled solutions, several factors need to be considered, including the heat load of the data center, available space, energy efficiency requirements, and budget constraints. It is essential to evaluate the specific needs and requirements of the data center to determine the most suitable cooling solution.
Precision Air Conditioning Systems for Temperature Control
Precision air conditioning systems are designed specifically for data centers and other critical environments that require precise temperature and humidity control. These systems provide a stable and controlled environment for IT equipment, ensuring optimal performance and reliability.
There are several types of precision air conditioning systems available, including direct expansion (DX) systems, chilled water systems, and hybrid systems. DX systems use refrigerant to cool the air directly, while chilled water systems use water as a cooling medium. Hybrid systems combine both DX and chilled water technologies to provide a more flexible and efficient cooling solution.
Precision air conditioning systems offer several benefits over traditional cooling solutions. They provide precise temperature control within a narrow range, ensuring that the equipment operates within optimal conditions. They also offer better humidity control, which is crucial for preventing equipment corrosion and static electricity buildup.
Furthermore, precision air conditioning systems are designed to be energy-efficient, with features such as variable speed fans and intelligent controls that optimize cooling capacity based on demand. This not only reduces energy consumption but also lowers operational costs.
Hot Aisle/Cold Aisle Containment for Energy Efficiency
Hot aisle/cold aisle containment is a popular approach to improve energy efficiency in data centers. This strategy involves separating the hot exhaust air from the cold supply air by using physical barriers or containment systems.
In a hot aisle/cold aisle layout, the server racks are arranged in alternating rows with cold air supplied from the front of the racks (cold aisle) and hot air expelled from the back of the racks (hot aisle). The containment system ensures that the hot air is contained within the hot aisle and does not mix with the cold supply air.
By separating the hot and cold air streams, hot aisle/cold aisle containment reduces the mixing of air, improving cooling efficiency and reducing energy consumption. It allows for more precise temperature control and eliminates hot spots within the data center.
There are several types of containment systems available, including aisle containment, rack-level containment, and room-level containment. Aisle containment involves enclosing the hot and cold aisles with physical barriers, such as doors or curtains. Rack-level containment involves enclosing individual server racks with doors or panels. Room-level containment involves enclosing the entire data center space with walls or partitions.
The choice of containment system depends on factors such as the size of the data center, the layout of the server racks, and the desired level of energy efficiency. It is important to carefully evaluate the specific requirements of the data center to determine the most suitable containment solution.
The Role of Data Center Infrastructure Management (DCIM) in Cooling Solutions
Data Center Infrastructure Management (DCIM) plays a crucial role in optimizing cooling solutions in data centers. DCIM software provides real-time monitoring and management of critical infrastructure components, including cooling systems, power distribution units, and environmental sensors.
DCIM software collects data from various sensors and devices throughout the data center, providing insights into temperature, humidity, airflow, and energy consumption. This data can be used to identify inefficiencies, optimize cooling capacity, and make informed decisions regarding cooling system upgrades or modifications.
There are several DCIM tools available for cooling management, including temperature monitoring systems, airflow management systems, and energy management systems. Temperature monitoring systems provide real-time temperature readings at various points within the data center, allowing for proactive temperature control.
Airflow management systems help optimize airflow distribution by identifying areas of high or low airflow and suggesting adjustments to cooling unit placement or airflow patterns. Energy management systems provide insights into energy consumption and efficiency, allowing for better resource allocation and cost optimization.
By leveraging DCIM tools for cooling management, data center operators can improve energy efficiency, reduce operational costs, and ensure the reliable operation of the equipment.
Cooling Solutions for High-Density Data Centers
High-density data centers, which house a large number of servers and equipment in a limited space, present unique cooling challenges. The high heat loads generated by the equipment require specialized cooling solutions to maintain optimal operating conditions.
One common cooling solution for high-density data centers is liquid cooling. Liquid-cooled systems, such as direct-to-chip cooling or immersion cooling, provide higher cooling capacities and more efficient heat dissipation compared to air-cooled systems. They can handle the high heat loads generated by densely packed servers and ensure reliable operation.
Another cooling solution for high-density data centers is rear-door heat exchangers. These devices are installed on the back of server racks and use chilled water or other coolants to remove heat directly from the exhaust air. Rear-door heat exchangers can significantly reduce the temperature of the exhaust air before it is released into the data center environment, improving overall cooling efficiency.
Additionally, high-density data centers can benefit from advanced airflow management techniques, such as hot aisle/cold aisle containment and in-row cooling. These strategies help optimize airflow distribution and prevent hot spots within the data center, ensuring that all equipment receives adequate cooling.
Future Trends in Data Center Cooling Architectures
The field of data center cooling is constantly evolving, driven by advancements in technology and increasing demands for energy efficiency. Several trends are shaping the future of data center cooling architectures.
One trend is the adoption of liquid cooling solutions. As data centers become more densely packed with high-performance servers, air-cooled systems may no longer be sufficient to handle the heat loads. Liquid-cooled systems offer higher cooling capacities and greater energy efficiency, making them an attractive option for future data centers.
Another trend is the integration of renewable energy sources into data center cooling systems. As sustainability becomes a top priority for many organizations, data centers are exploring ways to reduce their carbon footprint. This includes using renewable energy sources, such as solar or wind power, to generate electricity for cooling systems.
Furthermore, the use of artificial intelligence (AI) and machine learning (ML) in data center cooling is gaining traction. AI and ML algorithms can analyze vast amounts of data collected from sensors and devices within the data center, identifying patterns and optimizing cooling capacity in real-time. This can lead to more efficient cooling operations and reduced energy consumption.
Choosing the Right Cooling Solution for Your Data Center Needs
When it comes to choosing the right cooling solution for your data center needs, several factors need to be considered. These include the heat load of the data center, available space, energy efficiency requirements, budget constraints, and future scalability.
It is important to conduct a thorough assessment of the data center’s cooling requirements, taking into account factors such as server density, power consumption, and airflow patterns. This will help determine the cooling capacity needed and identify any potential hot spots or airflow issues.
Additionally, it is essential to evaluate the available space and infrastructure constraints. Some cooling solutions may require extensive modifications to the data center layout or infrastructure, which can be costly and time-consuming. It is important to choose a solution that can be easily integrated into the existing infrastructure without major disruptions.
Energy efficiency is another crucial factor to consider. Cooling systems can account for a significant portion of a data center’s energy consumption. Choosing an energy-efficient solution can help reduce operational costs and minimize environmental impact.
Finally, it is important to consider future scalability and flexibility. Data centers are constantly evolving, with new equipment being added or replaced regularly. The chosen cooling solution should be able to accommodate future growth and changes in equipment requirements.
In conclusion, cooling solutions play a critical role in data centers by ensuring optimal operating conditions for servers and equipment. The choice of cooling solution depends on factors such as heat load, available space, energy efficiency requirements, and budget constraints. There are several types of cooling solutions available, including air-cooled and liquid-cooled systems, precision air conditioning systems, and hot aisle/cold aisle containment systems.
Precision air conditioning systems provide precise temperature and humidity control, while hot aisle/cold aisle containment improves energy efficiency by separating hot and cold air streams. Data Center Infrastructure Management (DCIM) tools can help optimize cooling solutions by providing real-time monitoring and management of critical infrastructure components.
High-density data centers present unique cooling challenges, which can be addressed through liquid cooling solutions, rear-door heat exchangers, and advanced airflow management techniques. Future trends in data center cooling include the adoption of liquid cooling, integration of renewable energy sources, and the use of AI and ML algorithms for optimization.
When choosing a cooling solution for your data center needs, it is important to consider factors such as heat load, available space, energy efficiency requirements, budget constraints, and future scalability. By carefully evaluating these factors and selecting the most suitable cooling solution, data center operators can ensure the reliable operation of their equipment while minimizing operational costs and environmental impact.
For more information on data center cooling solutions and how they contribute to the overall architecture for temperature control, check out this related article: “Choosing the Right Data Center Cooling Systems” (https://datacenterinfo.com/choosing-the-right-data-center-cooling-systems/). This article provides valuable insights into the different types of cooling systems available for data centers and offers tips for selecting the most suitable solution based on specific requirements. It covers various aspects such as energy efficiency, scalability, and cost-effectiveness, helping businesses make informed decisions to optimize their data center cooling strategies.
Power distribution in data centers refers to the process of delivering electrical power from the utility grid to the various IT equipment and infrastructure within the facility. It involves the design, installation, and management of electrical systems that ensure a reliable and efficient supply of power to support the operation of critical IT equipment.
In today’s digital age, data centers play a crucial role in supporting the growing demand for computing resources. They house servers, storage devices, networking equipment, and other critical infrastructure that enable businesses to store, process, and transmit vast amounts of data. As data centers continue to grow in size and complexity, the importance of effective power distribution becomes even more significant.
Key Takeaways
- Power distribution is a critical component of data center architecture.
- Challenges in power distribution include high energy consumption, heat dissipation, and potential downtime.
- Optimizing power distribution can improve efficiency, reduce costs, and increase reliability.
- Key components of power distribution include UPS systems, PDUs, and power cords.
- Best practices for optimizing power distribution include load balancing, monitoring, and regular maintenance.
Understanding the Challenges in Power Distribution in Data Centers
Power distribution in data centers presents several challenges that need to be addressed for optimal performance and reliability. These challenges include high power density, heat dissipation, power quality issues, and scalability.
High power density is a common challenge in modern data centers. As more powerful servers and storage devices are deployed, the demand for electricity increases. This leads to higher power densities within the facility, which can strain the electrical infrastructure and increase the risk of power outages.
Heat dissipation is another critical challenge in power distribution. IT equipment generates a significant amount of heat during operation, and it must be effectively removed to prevent overheating and equipment failure. Proper cooling systems and airflow management are essential to maintain optimal operating temperatures.
Power quality issues can also impact data center operations. Voltage sags, surges, harmonics, and other electrical disturbances can cause equipment malfunctions or even complete system failures. Implementing power conditioning devices such as voltage regulators and surge protectors can help mitigate these issues.
Scalability is a challenge that arises as data centers expand or upgrade their infrastructure. Power distribution systems must be designed with future growth in mind to accommodate additional IT equipment without compromising performance or reliability.
Importance of Optimizing Power Distribution in Data Center Architecture
Optimizing power distribution in data center architecture offers several benefits, including cost savings, improved efficiency, increased reliability, and reduced downtime.
Cost savings can be achieved through efficient power distribution. By implementing energy-efficient equipment and practices, data centers can reduce their electricity consumption and lower their operational expenses. Additionally, optimizing power distribution can help avoid costly downtime and equipment failures caused by power-related issues.
Improved efficiency is another advantage of optimizing power distribution. By reducing energy waste and improving power utilization, data centers can maximize the performance of their IT equipment. This leads to better resource allocation and increased productivity.
Increased reliability is crucial for data centers that need to provide uninterrupted services to their customers. By implementing redundant power distribution systems and backup power sources, data centers can ensure continuous operation even in the event of a power outage or equipment failure.
Reduced downtime is a significant benefit of optimizing power distribution. Power-related issues are one of the leading causes of data center downtime. By implementing best practices in power distribution design and maintenance, data centers can minimize the risk of downtime and its associated costs.
Key Components of Power Distribution in Data Centers
Several key components are involved in power distribution in data centers. These components work together to ensure a reliable and efficient supply of power to the IT equipment.
Power distribution units (PDUs) are devices that distribute electrical power from the main electrical panel to the IT equipment racks. They provide multiple outlets for connecting servers, switches, and other devices. PDUs come in various types, including basic PDUs, metered PDUs, switched PDUs, and intelligent PDUs.
Uninterruptible power supply (UPS) systems are critical for providing backup power during a utility outage or voltage fluctuation. UPS systems use batteries or flywheels to store energy that can be used to keep the IT equipment running until the main power source is restored or a backup generator kicks in.
Generators are backup power sources that can provide electricity for an extended period during a utility outage. They are typically fueled by diesel, natural gas, or propane and are designed to start automatically when the main power source fails.
Transfer switches are devices that automatically switch the power source from the utility grid to the backup generator during a power outage. They ensure a seamless transition and prevent any interruption in power supply to the IT equipment.
Circuit breakers are essential for protecting the electrical infrastructure from overloads and short circuits. They automatically trip and disconnect the power supply when an abnormal condition is detected, preventing damage to the equipment and minimizing the risk of fire.
Best Practices for Optimizing Power Distribution in Data Center Architecture
To optimize power distribution in data center architecture, several best practices should be followed. These practices include proper planning and design, regular maintenance and testing, monitoring and management, and capacity planning.
Proper planning and design involve assessing the power requirements of the IT equipment and designing a power distribution system that can meet those requirements. This includes determining the appropriate capacity of PDUs, UPS systems, generators, and other components. It also involves considering factors such as redundancy, scalability, and future growth.
Regular maintenance and testing are crucial for ensuring the reliability of the power distribution system. This includes inspecting and cleaning electrical connections, testing UPS batteries, calibrating monitoring devices, and conducting load testing. Regular maintenance helps identify potential issues before they cause downtime or equipment failures.
Monitoring and management involve continuously monitoring the performance of the power distribution system and taking proactive measures to address any issues. This includes monitoring power consumption, temperature levels, voltage fluctuations, and other parameters. It also involves implementing remote management capabilities to allow for real-time monitoring and control.
Capacity planning is essential for ensuring that the power distribution system can accommodate future growth. By regularly assessing the power requirements of the IT equipment and projecting future needs, data centers can avoid overloading the electrical infrastructure and ensure scalability.
Importance of Redundancy and Resilience in Power Distribution
Redundancy and resilience are crucial aspects of power distribution in data centers. They help ensure continuous operation and minimize the risk of downtime.
N+1 redundancy is a common approach to achieve resilience in power distribution. It involves having one extra backup component for every N components in the system. For example, if a data center has N UPS systems, it would have N+1 UPS systems to provide redundancy. This ensures that even if one component fails, there is still a backup available to maintain power supply.
2N redundancy takes redundancy a step further by providing a complete duplicate of the power distribution system. This means that every component, including PDUs, UPS systems, generators, and transfer switches, has a redundant counterpart. This approach offers the highest level of resilience but comes at a higher cost.
Fault tolerance is another aspect of resilience in power distribution. It involves designing the system in such a way that it can continue to operate even if one or more components fail. This is achieved through redundant paths and automatic failover mechanisms.
Disaster recovery is an essential part of resilience in power distribution. It involves having a comprehensive plan in place to recover from major disruptions such as natural disasters or equipment failures. This includes backup power sources, off-site data replication, and procedures for restoring operations in a different location if necessary.
Strategies for Reducing Power Consumption in Data Centers
Reducing power consumption is a critical goal for data centers as it helps lower operational costs and minimize environmental impact. Several strategies can be employed to achieve this goal.
Virtualization is a technique that allows multiple virtual servers to run on a single physical server. By consolidating workloads and reducing the number of physical servers required, virtualization can significantly reduce power consumption.
Consolidation involves consolidating multiple servers or storage devices onto a smaller number of more powerful and energy-efficient systems. This reduces the overall power consumption and improves resource utilization.
Using energy-efficient hardware is another effective strategy for reducing power consumption. This includes selecting servers, storage devices, and networking equipment that are designed to operate efficiently and consume less power.
Cooling optimization is crucial for reducing power consumption in data centers. By implementing efficient cooling systems, such as hot aisle/cold aisle containment, economizers, and variable speed fans, data centers can reduce the energy required for cooling.
Impact of Renewable Energy Sources on Power Distribution in Data Centers
The use of renewable energy sources in data centers has gained significant attention in recent years. Renewable energy sources offer several benefits, including reduced carbon emissions and lower operational costs.
Solar power is one of the most popular renewable energy sources for data centers. Solar panels can be installed on the roof or in open areas to generate electricity from sunlight. This electricity can be used to power the data center directly or fed back into the grid.
Wind power is another viable option for data centers. Wind turbines can be installed on-site or in nearby locations to generate electricity from wind energy. This electricity can be used to supplement the power supply from the utility grid or provide backup power during outages.
Hydro power is a reliable and sustainable source of energy for data centers located near water bodies. Water turbines can be used to generate electricity from flowing water, such as rivers or streams. This electricity can be used to power the data center or stored in batteries for backup purposes.
Geothermal power utilizes the heat from the Earth’s core to generate electricity. Data centers located in geothermal-rich areas can tap into this renewable energy source to power their operations. Geothermal power offers a constant and reliable source of electricity, making it an attractive option for data centers.
Role of Automation in Power Distribution in Data Centers
Automation plays a crucial role in power distribution in data centers. It helps improve efficiency, reduce human error, and enable proactive management of the power infrastructure.
Intelligent PDUs are equipped with advanced features such as remote monitoring, outlet-level power metering, and environmental sensors. They provide real-time data on power consumption, temperature, humidity, and other parameters. This allows data center operators to monitor and manage the power distribution system more effectively.
Automated transfer switches are devices that can automatically switch the power source from the utility grid to a backup generator during a power outage. They eliminate the need for manual intervention and ensure a seamless transition.
Predictive maintenance uses advanced analytics and machine learning algorithms to predict equipment failures before they occur. By analyzing data from sensors and monitoring devices, predictive maintenance can identify patterns and trends that indicate potential issues. This allows data center operators to take proactive measures to prevent downtime and equipment failures.
Energy management systems integrate various components of the power distribution system, including PDUs, UPS systems, generators, and cooling systems. They provide centralized control and monitoring capabilities, allowing data center operators to optimize energy usage and reduce waste.
Future Trends in Power Distribution in Data Center Architecture
The future of power distribution in data center architecture is shaped by emerging trends such as edge computing, modular data centers, artificial intelligence (AI), and blockchain technology.
Edge computing involves moving computing resources closer to the source of data generation. This reduces latency and improves performance for applications that require real-time processing. Power distribution in edge data centers needs to be optimized for smaller footprints and lower power requirements.
Modular data centers are pre-fabricated units that can be quickly deployed and scaled as needed. They offer flexibility and cost savings compared to traditional brick-and-mortar data centers. Power distribution in modular data centers needs to be designed for easy installation and scalability.
Artificial intelligence (AI) has the potential to revolutionize power distribution in data centers. AI algorithms can analyze vast amounts of data from sensors and monitoring devices to optimize power usage, predict failures, and automate maintenance tasks. AI-powered energy management systems can help data centers achieve higher levels of efficiency and reliability.
Blockchain technology is being explored for its potential to improve the transparency and security of power distribution in data centers. Blockchain-based smart contracts can enable peer-to-peer energy trading and ensure fair and efficient allocation of power resources. This can lead to more sustainable and cost-effective power distribution.
Power distribution is a critical aspect of data center architecture that requires careful planning, design, and management. Optimizing power distribution offers several benefits, including cost savings, improved efficiency, increased reliability, and reduced downtime. Key components such as PDUs, UPS systems, generators, transfer switches, and circuit breakers play a crucial role in ensuring a reliable and efficient supply of power. Best practices such as proper planning, regular maintenance, monitoring, and capacity planning should be followed to optimize power distribution. Redundancy and resilience are essential for minimizing the risk of downtime, while strategies such as virtualization, consolidation, energy-efficient hardware, and cooling optimization can help reduce power consumption. The use of renewable energy sources, automation, and emerging trends such as edge computing, modular data centers, AI, and blockchain technology are shaping the future of power distribution in data center architecture. Data center operators must implement best practices and stay up-to-date with these trends to ensure optimal performance and reliability.
For more information on optimizing power distribution in data center architecture, check out this related article on “Unlocking Efficient Performance: Why Maximizing Datacenter Bandwidth is Key.” This article explores the importance of maximizing datacenter bandwidth for improved performance and cost savings. Learn about the strategies and best practices for optimizing bandwidth in your data center. Read more
In the digital era, cyber attacks pose an escalating threat to organizations worldwide. The increasing dependence on technology and interconnected data systems has heightened the vulnerability to such attacks. Cybercriminals continually refine their strategies to exploit weaknesses in data center operations, necessitating a proactive approach to cybersecurity.
The impact of cyber attacks can be severe, encompassing financial losses, reputational damage, and legal consequences. Common forms of attacks include ransomware, data breaches, phishing scams, and malware infections. As the frequency and complexity of these threats grow, businesses must prioritize cybersecurity to protect their data center operations.
The cyber threat landscape is dynamic, with attackers employing increasingly sophisticated methods. Successful breaches can result in data loss, operational disruptions, and significant financial repercussions. To mitigate these risks, organizations must invest in robust cybersecurity measures, stay informed about emerging threats and vulnerabilities, and implement industry best practices.
Proactive steps to enhance data center security include:
1. Regular security audits and vulnerability assessments
2. Implementation of multi-factor authentication
3.
Encryption of sensitive data
4. Continuous employee training on cybersecurity awareness
5. Deployment of advanced threat detection and prevention systems
6.
Development and testing of incident response plans
By recognizing the growing cyber threat and taking decisive action, businesses can better protect their data center operations and maintain the integrity of their digital assets.
Key Takeaways
- Cyber attacks are a growing threat, with data centers being a prime target for hackers.
- Vulnerabilities in data center operations can lead to devastating cybersecurity breaches.
- Cybersecurity breaches can have a significant impact on data centers, including financial losses and damage to reputation.
- Implementing robust cybersecurity measures is crucial for protecting data center operations from cyber attacks.
- Best practices for securing data center operations include regular security audits, employee training, and implementing multi-layered security measures.
Vulnerabilities in Data Center Operations
Physical Security Risks
Physical security vulnerabilities such as unauthorized access to data centers or inadequate security protocols can compromise the integrity of data storage and processing.
Network Vulnerabilities and Insider Threats
Similarly, network vulnerabilities such as unsecured connections or outdated software can provide entry points for cyber attackers to infiltrate data center operations. Additionally, human error and insider threats can also pose significant risks to the security of data center operations.
Complexity of IT Infrastructure and the Need for Regular Assessments
One of the key vulnerabilities in data center operations is the increasing complexity of IT infrastructure. As organizations adopt new technologies and expand their digital footprint, the complexity of their IT systems also grows, creating more potential points of vulnerability. This complexity can make it challenging for organizations to effectively monitor and secure their data center operations, leaving them susceptible to cyber attacks. As such, it is essential for businesses to conduct regular assessments of their data center operations to identify and address vulnerabilities before they can be exploited by cyber attackers.
Impact of Cybersecurity Breaches on Data Centers
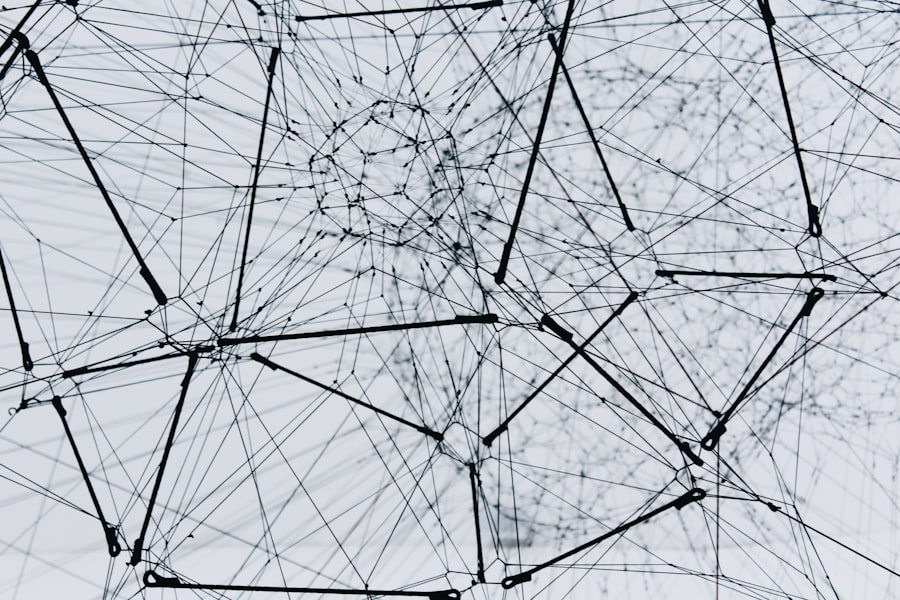
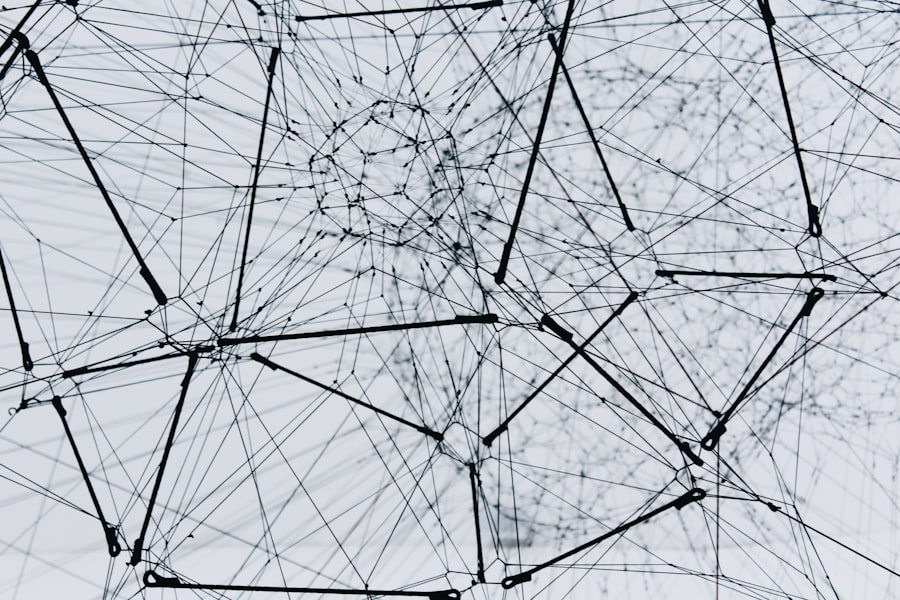
The impact of cybersecurity breaches on data centers can be far-reaching and severe. In addition to financial losses and operational disruptions, organizations that experience a cybersecurity breach may also suffer reputational damage and legal implications. For example, a data breach can result in the loss of sensitive customer information, leading to a loss of trust and confidence in the organization.
This can have long-term implications for the business, affecting customer retention and brand reputation. Additionally, organizations may also face legal consequences if they fail to adequately protect customer data, leading to regulatory fines and legal action. Furthermore, the operational impact of a cybersecurity breach can be significant, leading to downtime, loss of productivity, and damage to critical infrastructure.
For example, a ransomware attack can encrypt critical data and systems, rendering them inaccessible until a ransom is paid. This can result in significant downtime and financial losses for the organization. Additionally, the reputational damage caused by a cybersecurity breach can have long-term implications for the business, affecting customer trust and loyalty.
As such, it is essential for organizations to recognize the potential impact of cybersecurity breaches on their data center operations and take proactive measures to mitigate these risks.
Importance of Implementing Robust Cybersecurity Measures
The importance of implementing robust cybersecurity measures cannot be overstated in today’s digital landscape. With the growing threat of cyber attacks and the potential impact on data center operations, organizations must prioritize cybersecurity as a fundamental aspect of their business strategy. Robust cybersecurity measures not only protect sensitive data and critical infrastructure but also safeguard the organization’s reputation and financial stability.
By investing in cybersecurity, organizations can mitigate the risk of cyber attacks and minimize the potential impact on their data center operations. Implementing robust cybersecurity measures is essential for protecting sensitive data from unauthorized access or theft. This includes implementing encryption protocols, access controls, and monitoring systems to ensure that only authorized personnel have access to sensitive information.
Additionally, robust cybersecurity measures can also help organizations detect and respond to potential threats in a timely manner, minimizing the impact on data center operations. By investing in cybersecurity, organizations can demonstrate their commitment to protecting customer data and maintaining a secure operating environment. This can help build trust with customers and stakeholders, enhancing the organization’s reputation and competitive advantage.
Best Practices for Securing Data Center Operations
Securing data center operations requires a multi-faceted approach that addresses physical security, network security, and personnel training. One of the best practices for securing data center operations is implementing robust access controls and monitoring systems to prevent unauthorized access to sensitive information. This includes using encryption protocols, multi-factor authentication, and role-based access controls to ensure that only authorized personnel have access to critical data and systems.
Additionally, organizations should regularly review and update their access controls to adapt to evolving threats and vulnerabilities. Another best practice for securing data center operations is implementing regular security assessments and audits to identify potential vulnerabilities and weaknesses. This includes conducting penetration testing, vulnerability scanning, and security audits to proactively identify and address potential points of weakness in data center operations.
By regularly assessing the security posture of their data center operations, organizations can stay ahead of potential threats and minimize the risk of cyber attacks. Furthermore, organizations should prioritize employee training and awareness programs to educate personnel about best practices for cybersecurity. This includes training employees on how to recognize potential threats such as phishing scams or social engineering tactics.
By empowering employees with the knowledge and skills to identify and respond to potential threats, organizations can strengthen their overall security posture and minimize the risk of human error leading to a cybersecurity breach.
The Role of Compliance and Regulations in Cybersecurity
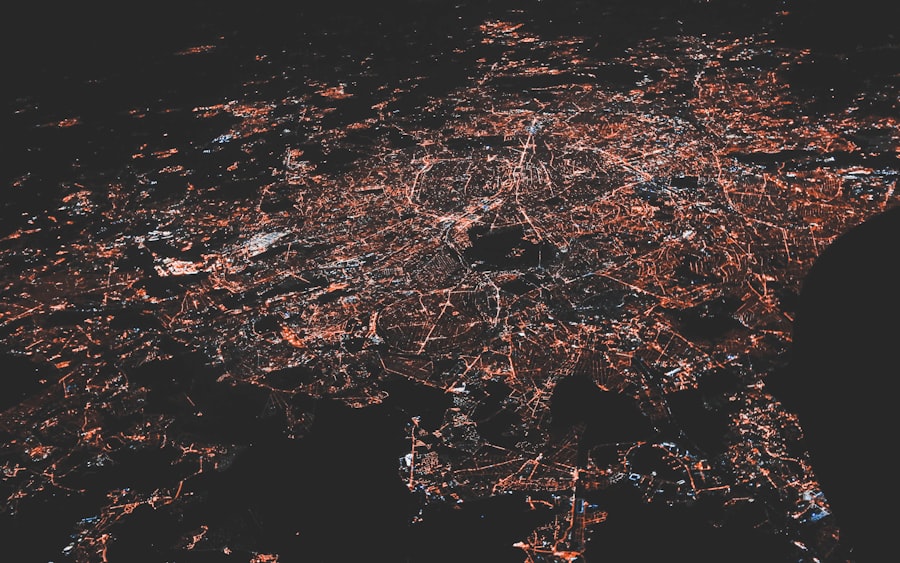
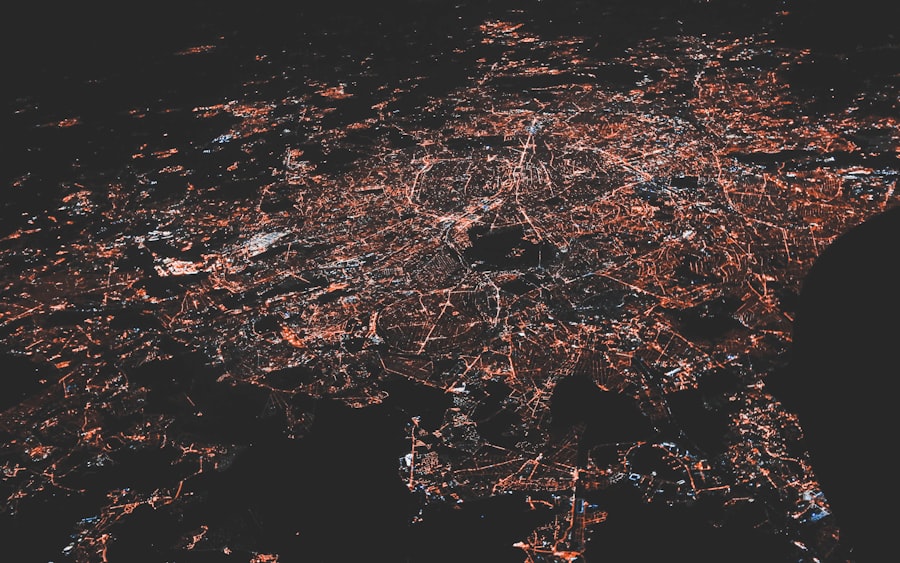
Industry-Specific Regulations and Compliance Standards
Certain industries, such as healthcare and finance, are subject to strict regulatory requirements like HIPAA and PCI DSS. These regulations dictate how sensitive information should be protected, and failure to comply can result in significant fines and legal consequences.
Benefits of Compliance Standards
Compliance standards provide a framework for organizations to follow best practices for cybersecurity. By adhering to industry-specific regulations and compliance standards, organizations can ensure they are implementing robust cybersecurity measures that align with industry best practices. This helps demonstrate their commitment to protecting sensitive data and maintaining a secure operating environment, enhancing trust with customers and stakeholders.
Measuring Cybersecurity Posture
Compliance standards also provide a benchmark for organizations to measure their cybersecurity posture against industry best practices. By aligning with compliance standards, organizations can ensure they are meeting minimum requirements for cybersecurity while striving for continuous improvement in their security posture. This helps organizations stay ahead of potential threats and vulnerabilities while demonstrating their commitment to maintaining a secure operating environment.
Investing in Cybersecurity for Long-Term Protection
Investing in cybersecurity is essential for long-term protection of data center operations. By prioritizing cybersecurity as a fundamental aspect of their business strategy, organizations can mitigate the risk of cyber attacks and minimize the potential impact on their data center operations. This includes investing in robust cybersecurity measures such as encryption protocols, access controls, monitoring systems, and employee training programs to protect sensitive data from unauthorized access or theft.
Furthermore, investing in cybersecurity can also help organizations build trust with customers and stakeholders by demonstrating their commitment to protecting sensitive information. This can enhance the organization’s reputation and competitive advantage while also minimizing the potential impact of reputational damage caused by a cybersecurity breach. Additionally, investing in cybersecurity can also help organizations stay ahead of potential threats and vulnerabilities by proactively identifying and addressing points of weakness in their data center operations.
By regularly assessing their security posture through security assessments and audits, organizations can minimize the risk of cyber attacks while also demonstrating their commitment to maintaining a secure operating environment. In conclusion, the growing threat of cyber attacks poses significant risks to data center operations. Organizations must recognize the vulnerabilities in their data center operations and take proactive measures to implement robust cybersecurity measures.
By prioritizing cybersecurity as a fundamental aspect of their business strategy, organizations can mitigate the risk of cyber attacks while also building trust with customers and stakeholders. Compliance standards provide a framework for organizations to follow best practices for cybersecurity while also providing a benchmark for continuous improvement in their security posture. Investing in cybersecurity is essential for long-term protection of data center operations, helping organizations stay ahead of potential threats while also demonstrating their commitment to maintaining a secure operating environment.
The Critical Need for Cybersecurity in Data Center Operations is crucial for protecting sensitive information and maintaining the integrity of digital infrastructure. For more information on best practices for physical and digital security measures in data centers, check out this article. It provides valuable insights into the importance of securing data centers from both physical and digital threats.
FAQs
What is cybersecurity in data center operations?
Cybersecurity in data center operations refers to the practices and measures put in place to protect the data, systems, and networks within a data center from cyber threats and attacks.
Why is cybersecurity important in data center operations?
Cybersecurity is important in data center operations because data centers store and process sensitive and critical information for organizations. Without proper cybersecurity measures, this data is at risk of being compromised, leading to financial loss, reputational damage, and legal implications.
What are the common cyber threats to data center operations?
Common cyber threats to data center operations include malware, ransomware, phishing attacks, DDoS attacks, insider threats, and unauthorized access.
What are the potential consequences of a cyber attack on a data center?
The potential consequences of a cyber attack on a data center include data breaches, financial loss, operational disruption, reputational damage, legal and regulatory penalties, and loss of customer trust.
What are some best practices for cybersecurity in data center operations?
Best practices for cybersecurity in data center operations include implementing strong access controls, regular security assessments and audits, employee training and awareness programs, encryption of data, and the use of advanced security technologies such as firewalls and intrusion detection systems.