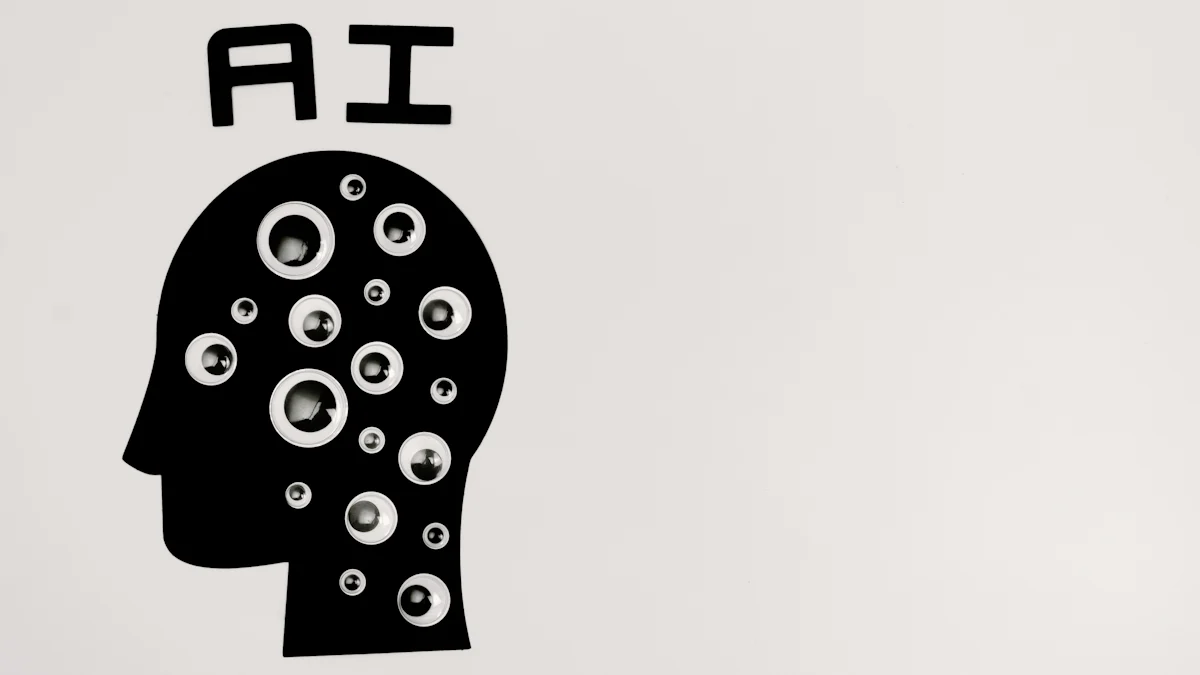
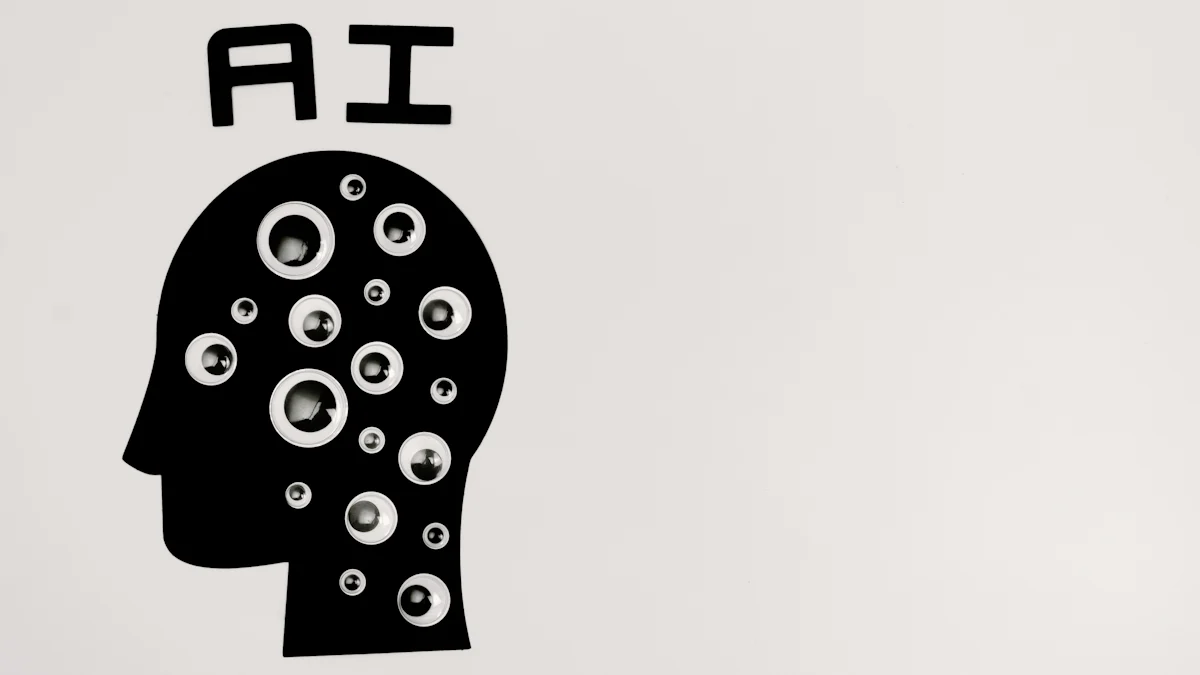
In the realm of artificial intelligence, understanding the financial implications of infrastructure investments has become indispensable. A well-executed TCO analysis empowers organizations to make informed decisions by evaluating the long-term costs and benefits of their AI systems. This approach ensures that businesses balance immediate expenses with sustainable returns. For instance, companies that optimize their AI cost analysis often achieve up to 3X higher ROI compared to those with fragmented strategies. By adopting a structured evaluation, organizations can unlock greater efficiency, reduce hidden costs, and maximize the value of their artificial intelligence initiatives.
Key Takeaways
Understanding Total Cost of Ownership (TCO) is essential for making informed financial decisions regarding AI infrastructure, as it encompasses all costs over the system’s lifecycle.
A structured TCO analysis can help organizations avoid hidden costs and optimize their AI investments, potentially achieving up to 3X higher ROI compared to fragmented strategies.
When evaluating deployment models, consider the unique cost structures of on-premises, cloud-based, and SaaS solutions to align with your organization’s budget and operational needs.
Regularly revisiting and updating TCO calculations ensures that businesses remain prepared for unforeseen financial demands and can adapt to changing project requirements.
Implementing cost optimization strategies, such as efficient resource allocation and leveraging hybrid models, can significantly reduce the total cost of ownership for AI systems.
Negotiating vendor contracts effectively is crucial for minimizing costs and ensuring long-term value, so businesses should focus on transparency and flexibility during negotiations.
Understanding TCO and Its Importance
What Is TCO?
Total Cost of Ownership (TCO) refers to the comprehensive assessment of all costs associated with acquiring, operating, and maintaining a system or infrastructure over its entire lifecycle. In the context of artificial intelligence, TCO encompasses expenses such as hardware procurement, software licensing, labor, data acquisition, and ongoing operational costs. This holistic approach ensures that organizations account for both visible and hidden costs when planning AI deployments.
Unlike simple upfront cost evaluations, TCO calculations provide a detailed view of long-term financial commitments. For example, deploying AI systems often involves recurring expenses like cloud service subscriptions or hardware upgrades. By calculating TCO, businesses can avoid underestimating these costs and ensure sustainable investments. Tools like TCO calculators, which evaluate deployment and running costs, help organizations estimate expenses such as cost per request and labor requirements. These tools also highlight potential areas for cost optimization.
“The Google Cloud article emphasizes the importance of modeling TCO for each AI use case to optimize costs and improve efficiency.” This insight underscores the need for businesses to tailor their TCO analysis to specific AI applications, ensuring accurate financial planning.
Why Is TCO Critical for AI Infrastructure?
TCO analysis plays a pivotal role in guiding organizations toward informed decision-making for AI infrastructure. Artificial intelligence systems, particularly those involving large-scale models, demand significant resources. Without a clear understanding of total cost of ownership, businesses risk overspending or underutilizing their investments. A structured TCO analysis helps mitigate these risks by providing a roadmap for cost management.
AI cost analysis becomes especially critical when comparing deployment models such as on-premises infrastructure, cloud-based solutions, or SaaS platforms. Each model presents unique cost structures, and TCO calculations enable businesses to weigh these options effectively. For instance, cloud-based AI services may reduce upfront costs but introduce variable operational expenses. On the other hand, on-premises setups require substantial initial investments but offer greater control over long-term costs.
Hidden costs, such as workforce re-engagement or hardware maintenance, often go unnoticed during initial budgeting. By incorporating these factors into TCO analysis, organizations can identify potential financial pitfalls. This proactive approach ensures that AI deployments remain financially viable over time.
In industries with strict data sovereignty requirements, TCO analysis becomes even more critical. Businesses must balance compliance needs with cost considerations, particularly when evaluating cloud-based AI solutions. A thorough understanding of TCO allows organizations to align their financial strategies with operational goals, ensuring both efficiency and compliance.
Breaking Down the Key Cost Components
Infrastructure Costs
Infrastructure costs form the foundation of any AI system. These expenses include hardware, software, and networking components required to build and maintain AI infrastructure. High-performance computing resources, such as GPUs and TPUs, are essential for training and deploying AI models. The cost of these components can vary significantly based on the scale and complexity of the project. For instance, small-scale AI projects may require investments ranging from $50,000 to $500,000, while large-scale initiatives can exceed $5 million.
Cloud-based solutions often provide scalable infrastructure options, reducing the need for substantial upfront investments. However, they introduce recurring operational expenses, such as subscription fees and data transfer costs. On-premises setups, on the other hand, demand significant initial capital but offer greater control over long-term costs. Businesses must carefully evaluate these options through detailed TCO calculations to determine the most cost-effective approach for their specific needs.
Additionally, infrastructure maintenance plays a critical role in sustaining AI systems. Regular hardware upgrades, software updates, and system monitoring ensure optimal performance. Neglecting these aspects can lead to inefficiencies and increased operational costs over time.
Labor Costs
Labor costs represent a significant portion of the total cost of ownership for AI infrastructure. Developing, deploying, and maintaining AI systems require skilled professionals, including data scientists, machine learning engineers, and IT specialists. Salaries for these roles can vary widely depending on expertise and geographic location. For example, hiring a team of experienced AI professionals for a complex project may cost hundreds of thousands of dollars annually.
Beyond salaries, businesses must consider the cost of training and upskilling their workforce. AI technologies evolve rapidly, necessitating continuous learning to stay updated with the latest advancements. Investing in employee development ensures that teams remain proficient in managing AI systems, ultimately reducing the risk of errors and inefficiencies.
Outsourcing certain tasks, such as data annotation or model development, can help manage labor costs. However, organizations must weigh the benefits of outsourcing against potential challenges, such as quality control and data security concerns. Calculating TCO accurately requires factoring in all labor-related expenses, including hidden costs like recruitment and onboarding.
Data Costs
Data serves as the backbone of AI systems, making data-related costs a critical component of TCO analysis. These costs encompass data collection, storage, preprocessing, and management. For instance, acquiring high-quality datasets often involves expenses for data licensing, annotation, and cleaning. Complex AI projects may require vast amounts of data, driving up these costs significantly.
Storage and management of large datasets also contribute to data costs. Cloud storage solutions offer scalability but come with recurring fees based on usage. On-premises storage provides greater control but demands substantial initial investments in hardware and maintenance. Organizations must evaluate these options carefully to optimize data-related expenses.
Preprocessing and ensuring data quality are equally important. Poor-quality data can lead to inaccurate model predictions, resulting in wasted resources and additional costs for retraining. Businesses should prioritize robust data management practices to minimize these risks and enhance the efficiency of their AI systems.
“AI is not possible without massive amounts of data, leading to various data-related costs that should be factored into the potential cost of an AI system.” This insight highlights the importance of incorporating all data-related expenses into TCO calculations to ensure comprehensive financial planning.
Operational Costs
Operational costs represent a significant portion of the total cost of ownership for AI infrastructure. These expenses arise from the day-to-day functioning and upkeep of AI systems, ensuring their reliability and efficiency over time. Businesses must account for several key areas when evaluating operational costs to maintain financial sustainability.
One major contributor to operational costs is energy consumption. AI systems, particularly those involving high-performance computing, require substantial power to operate. Training large-scale models or running inference tasks can lead to high electricity bills, especially in on-premises setups. Organizations should monitor energy usage closely and explore energy-efficient hardware or cooling solutions to mitigate these expenses.
System maintenance also plays a critical role in operational costs. Regular updates to software, firmware, and security protocols ensure that AI systems remain functional and secure. Neglecting maintenance can result in system downtime, which disrupts operations and incurs additional costs. Businesses should allocate resources for routine inspections and timely upgrades to avoid such issues.
Another factor to consider is the cost of managing data pipelines. AI systems rely on continuous data ingestion, preprocessing, and storage. Operational costs increase as data volumes grow, particularly for projects requiring real-time data processing. Cloud-based solutions offer scalability but introduce recurring fees tied to data transfer and storage. On-premises systems demand investments in physical storage and IT personnel to manage these pipelines effectively.
Organizations must also address the costs associated with monitoring and troubleshooting AI systems. Deploying AI models in production environments often requires constant oversight to ensure optimal performance. Issues such as model drift or hardware failures can arise, necessitating immediate intervention. Businesses should invest in robust monitoring tools and skilled personnel to minimize disruptions and maintain system efficiency.
“Operational costs often escalate due to hidden factors like energy consumption and system downtime. Proactive management of these elements ensures long-term cost efficiency.” This insight highlights the importance of a comprehensive approach to operational cost analysis.
To optimize operational costs, businesses can adopt strategies such as automating routine tasks, leveraging predictive maintenance tools, and implementing hybrid infrastructure models. These measures reduce manual intervention, enhance system reliability, and balance cost efficiency with performance.
Comparing Deployment Models for AI Infrastructure
Selecting the right deployment model for AI infrastructure is a critical decision that impacts both cost and performance. Businesses must evaluate their unique requirements, including scalability, control, and budget, to determine the most suitable option. This section explores three primary deployment models: on-premises, cloud-based, and SaaS (Software as a Service), highlighting their distinct characteristics and implications for total cost of ownership (TCO).
On-Premises AI Infrastructure
On-premises AI infrastructure provides organizations with complete control over their hardware and software resources. This model involves deploying AI systems within a company’s physical data centers, utilizing specialized hardware such as GPUs, TPUs, or ASICs. While this approach offers enhanced security and compliance, it demands significant upfront investments in equipment and facilities.
The TCO for on-premises infrastructure includes not only the initial hardware costs but also ongoing expenses for maintenance, energy consumption, and system upgrades. For instance, high-performance computing resources require regular updates to remain efficient, which can increase operational costs over time. Additionally, businesses must allocate resources for IT personnel to manage and monitor these systems effectively.
Despite the higher initial costs, on-premises infrastructure can be advantageous for organizations with strict data sovereignty requirements or those handling sensitive information. By maintaining full control over their systems, businesses can ensure compliance with industry regulations while optimizing performance for specific AI workloads.
Cloud-Based AI Infrastructure
Cloud-based AI infrastructure has gained popularity due to its flexibility and scalability. This model allows businesses to access computing resources through cloud service providers, eliminating the need for substantial upfront investments. Companies can scale their resources up or down based on demand, making it an attractive option for projects with variable workloads.
The TCO for cloud-based infrastructure primarily consists of recurring operational expenses, such as subscription fees and data transfer costs. Cloud TCO models help organizations estimate these expenses by analyzing factors like usage patterns and resource allocation. For example, inference tasks often rely on expensive GPU-based compute resources, which can drive up costs if not managed efficiently.
Cloud-based solutions also simplify the AI deployment process by providing pre-configured environments and tools. However, businesses must consider potential challenges, such as data security and vendor lock-in. A thorough ai cost analysis ensures that organizations can balance the benefits of scalability with the risks associated with cloud-based systems.
“Comprehensive financial planning is essential for cloud-based AI projects to prevent unexpected expenses and ensure accurate budgeting.” This insight underscores the importance of evaluating all cost components when adopting cloud-based infrastructure.
SaaS (Software as a Service) for AI
SaaS for AI offers a streamlined approach to deploying AI applications. This model enables businesses to access AI tools and services through subscription-based platforms, eliminating the need for extensive infrastructure investments. SaaS solutions are particularly beneficial for organizations seeking to implement AI quickly without the complexities of managing hardware and software.
The TCO for SaaS models includes subscription fees, which often cover software updates, maintenance, and support. While these costs are predictable, businesses must assess the scalability and customization options offered by SaaS providers. Limited flexibility may pose challenges for organizations with unique requirements or large-scale AI projects.
SaaS platforms excel in reducing the time and effort required for AI deployment. They provide ready-to-use solutions that cater to specific use cases, such as natural language processing or image recognition. However, businesses should evaluate the long-term costs and potential limitations of relying on third-party providers for critical AI functions.
“SaaS solutions simplify AI adoption but require careful consideration of scalability and customization needs.” This perspective highlights the importance of aligning SaaS offerings with organizational goals to maximize value.
Strategies for Cost Optimization in TCO Analysis
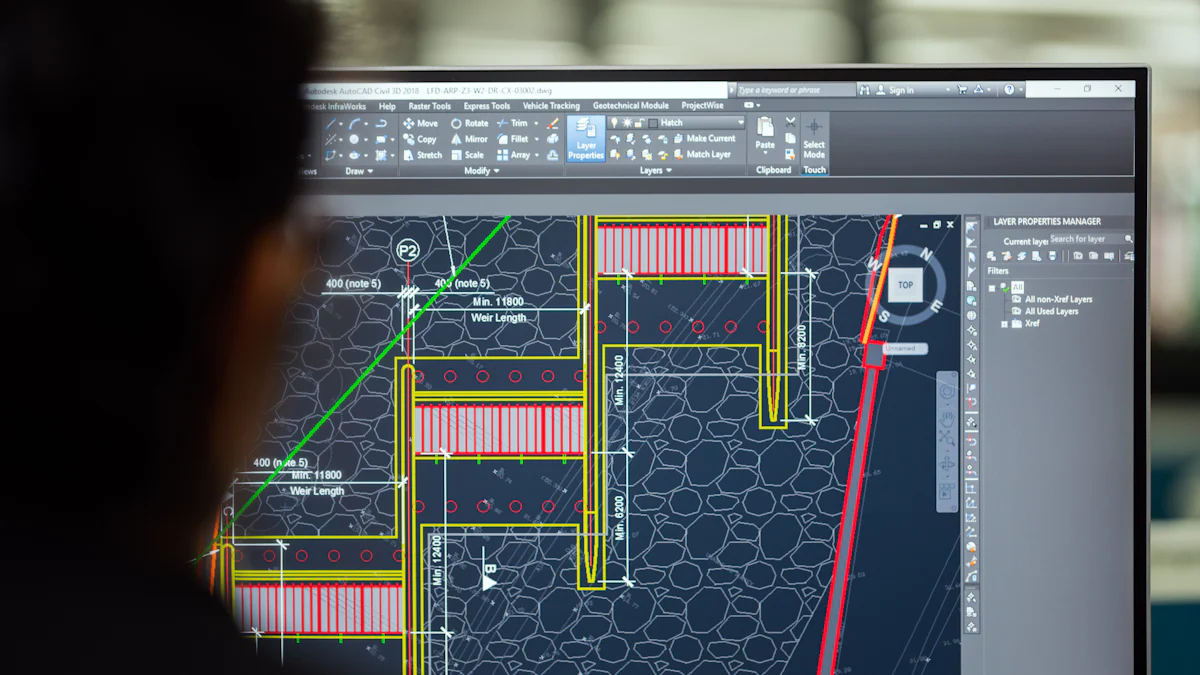
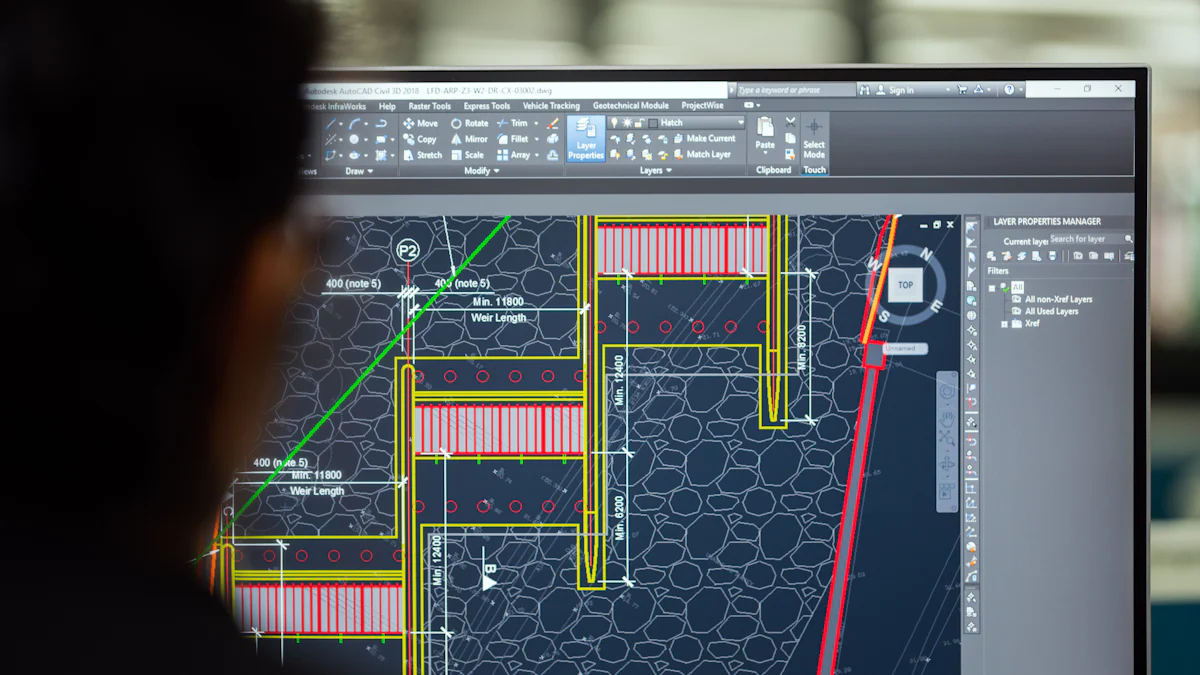
Optimizing Infrastructure Usage
Efficient use of infrastructure plays a pivotal role in reducing the total cost of ownership for AI systems. Organizations must evaluate their resource allocation to ensure optimal performance without unnecessary expenditure. For instance, businesses can adopt workload scheduling techniques to maximize the utilization of high-performance computing resources like GPUs and TPUs. By aligning computational tasks with periods of lower demand, companies can minimize idle time and reduce energy consumption.
Another effective approach involves leveraging automation tools to monitor and manage infrastructure usage. These tools provide real-time insights into resource consumption, enabling businesses to identify inefficiencies and implement corrective measures. For example, scaling down unused virtual machines or reallocating underutilized resources can significantly lower operational costs.
“Continuous monitoring of infrastructure usage ensures that organizations avoid overspending while maintaining system efficiency.” This proactive strategy helps businesses achieve sustainable cost management.
Additionally, organizations should consider adopting containerization and orchestration platforms, such as Kubernetes, to streamline resource management. These platforms enable efficient deployment and scaling of AI workloads, ensuring that infrastructure resources are used effectively. Through detailed TCO calculations, businesses can identify areas for improvement and implement strategies to optimize infrastructure usage.
Reducing Data Costs
Data-related expenses often constitute a significant portion of AI cost analysis. To reduce these costs, organizations must prioritize efficient data management practices. For instance, businesses can implement data deduplication techniques to eliminate redundant information, thereby reducing storage requirements. Compressing datasets before storage also minimizes costs associated with cloud-based solutions.
Another strategy involves selecting high-quality datasets that align with specific AI objectives. Instead of acquiring vast amounts of generic data, organizations should focus on targeted datasets that directly contribute to model accuracy and performance. This approach not only reduces acquisition costs but also minimizes preprocessing efforts.
“AI is not possible without massive amounts of data, leading to various data-related costs that should be factored into the potential cost of an AI system.” By addressing these costs strategically, businesses can enhance the financial viability of their AI initiatives.
Cloud TCO models offer valuable insights into data storage and transfer expenses. These models help organizations evaluate the cost implications of different storage options, such as on-premises systems versus cloud-based solutions. For example, businesses handling sensitive data may opt for hybrid storage models to balance cost efficiency with compliance requirements. Calculating TCO accurately ensures that all data-related expenses are accounted for, enabling informed decision-making.
Leveraging Hybrid Models
Hybrid models combine the benefits of on-premises and cloud-based AI infrastructure, offering a flexible and cost-effective solution. This approach allows organizations to allocate workloads based on specific requirements, optimizing both performance and expenses. For instance, businesses can use on-premises systems for sensitive data processing while leveraging cloud resources for scalable tasks like model training.
The hybrid model also provides a safeguard against vendor lock-in, enabling organizations to diversify their infrastructure investments. By distributing workloads across multiple platforms, businesses can mitigate risks associated with dependency on a single provider. This strategy enhances operational resilience while maintaining cost efficiency.
“To optimize AI costs, we recommend these strategies: It is essential to select AI projects that directly address specific, measurable business objectives and contribute to the overall strategic vision.” Hybrid models align with this recommendation by enabling tailored resource allocation for diverse AI projects.
Organizations adopting hybrid models should invest in robust integration tools to ensure seamless communication between on-premises and cloud systems. These tools facilitate efficient data transfer and workload distribution, minimizing operational disruptions. Through comprehensive TCO calculations, businesses can evaluate the financial impact of hybrid models and identify opportunities for further optimization.
Negotiating Vendor Contracts
Negotiating vendor contracts plays a crucial role in optimizing the total cost of ownership (TCO) for AI infrastructure. Businesses must approach this process strategically to secure favorable terms that align with their financial and operational goals. Effective negotiation not only reduces costs but also ensures long-term value from vendor partnerships.
Key Considerations for Vendor Negotiations
Organizations should evaluate several factors when negotiating contracts with vendors. These considerations help businesses identify opportunities for cost savings and mitigate potential risks:
Pricing Models: Vendors often offer various pricing structures, such as pay-as-you-go, subscription-based, or tiered pricing. Businesses must analyze these models to determine which aligns best with their usage patterns and budget constraints.
Service Level Agreements (SLAs): SLAs define the performance standards and support levels vendors must meet. Clear and enforceable SLAs ensure accountability and minimize disruptions caused by service failures.
Hidden Costs: Contracts may include hidden fees, such as data transfer charges, maintenance costs, or penalties for exceeding usage limits. Identifying and addressing these costs during negotiations prevents unexpected expenses.
Scalability Options: AI workloads often fluctuate, requiring flexible infrastructure solutions. Vendors offering scalable resources enable businesses to adapt to changing demands without incurring excessive costs.
Strategies for Successful Negotiations
To achieve favorable outcomes, businesses should adopt a structured approach to vendor negotiations. The following strategies can enhance the effectiveness of this process:
Conduct Thorough Research
Organizations must gather detailed information about vendors, including their pricing structures, service offerings, and market reputation. Comparing multiple vendors provides leverage during negotiations and helps identify the most competitive options.Define Clear Objectives
Businesses should establish specific goals for the negotiation process, such as reducing costs, securing flexible terms, or obtaining additional support services. Clear objectives guide discussions and ensure alignment with organizational priorities.Leverage Competitive Bidding
Inviting multiple vendors to submit proposals fosters competition and encourages them to offer better terms. This approach enables businesses to evaluate various options and select the most cost-effective solution.Negotiate Custom Terms
Standard contracts may not always meet the unique needs of an organization. Businesses should request customized terms that address their specific requirements, such as tailored pricing models or enhanced support services.Focus on Long-Term Value
While upfront cost reductions are important, businesses must also consider the long-term value of vendor partnerships. Factors such as reliability, scalability, and support quality significantly impact the overall TCO.
Common Pitfalls to Avoid
During vendor negotiations, businesses should remain vigilant to avoid common pitfalls that could undermine their efforts:
Overlooking Contract Details: Failing to review contract terms thoroughly may result in unfavorable conditions or hidden costs. Organizations must scrutinize all clauses to ensure transparency and fairness.
Relying on a Single Vendor: Dependence on one vendor increases the risk of vendor lock-in and limits bargaining power. Diversifying vendor relationships enhances flexibility and reduces risks.
Neglecting Exit Clauses: Contracts should include clear exit clauses that allow businesses to terminate agreements without excessive penalties. This ensures flexibility in case the vendor fails to meet expectations.
“Negotiating vendor contracts effectively requires a balance between cost reduction and value creation. Businesses must prioritize transparency, flexibility, and long-term benefits to achieve sustainable success.”
By adopting these strategies and avoiding common pitfalls, organizations can optimize their vendor contracts and reduce the TCO of their AI infrastructure. A well-negotiated contract not only minimizes expenses but also strengthens vendor relationships, ensuring reliable support for AI initiatives.
Limitations and Considerations in TCO Analysis
Challenges in Accurate Cost Estimation
Accurately estimating the total cost of ownership (TCO) for AI infrastructure presents significant challenges. AI systems involve numerous cost components, many of which are dynamic and difficult to predict. For instance, hardware expenses, such as GPUs or TPUs, can fluctuate based on market demand and technological advancements. These variations make it challenging for businesses to forecast long-term costs with precision.
Operational costs further complicate cost estimation. Energy consumption, system maintenance, and data storage requirements often increase as AI systems scale. Businesses may underestimate these expenses during initial planning, leading to budget overruns. Additionally, hidden costs, such as those associated with workforce training or unexpected system downtime, frequently go unnoticed. These overlooked factors can significantly impact the overall financial viability of AI projects.
Another layer of complexity arises from the integration of AI into existing systems. Customizing AI solutions to align with organizational workflows often incurs additional development and deployment costs. These expenses vary widely depending on the complexity of the AI model and the compatibility of existing infrastructure. For example, integrating a simple AI model may cost $5,000, while deploying a complex solution could exceed $500,000.
“Achieving a high return on investment (ROI) poses a considerable challenge for businesses that lack effective AI cost optimization strategies.” This highlights the importance of thorough cost estimation to ensure that AI investments deliver measurable value.
To address these challenges, businesses must adopt a structured approach to ai cost analysis. Leveraging tools like TCO calculators can help organizations identify potential cost drivers and estimate expenses more accurately. Regularly revisiting and updating cost projections ensures that businesses remain prepared for unforeseen financial demands.
Balancing Cost and Performance
Balancing cost and performance is a critical consideration in TCO analysis for AI infrastructure. Organizations often face trade-offs between minimizing expenses and achieving optimal system performance. For instance, investing in high-performance hardware may increase upfront costs but significantly enhance the speed and accuracy of AI models. Conversely, opting for lower-cost alternatives could result in slower processing times and reduced model efficiency.
The choice of deployment model also influences this balance. On-premises infrastructure offers greater control over performance but demands substantial capital investment. Cloud-based solutions provide scalability and flexibility, yet they introduce recurring operational expenses. Businesses must carefully evaluate these options to align their financial strategies with performance goals.
Data quality plays a pivotal role in this equation. High-quality datasets improve model accuracy but often come at a premium. Acquiring and preprocessing such data can drive up costs, particularly for projects requiring extensive data annotation or cleaning. However, poor-quality data can lead to inaccurate predictions, necessitating costly retraining efforts. Striking the right balance between data quality and cost is essential for maintaining both financial and operational efficiency.
“AI requires computational resources, and the overall costs for implementing and maintaining AI can vary widely depending on various factors.” This underscores the need for businesses to weigh cost considerations against performance requirements when planning AI deployments.
To achieve this balance, organizations should prioritize cost-effective strategies that do not compromise performance. For example, leveraging hybrid models allows businesses to allocate workloads based on specific needs, optimizing both cost and efficiency. Additionally, adopting energy-efficient hardware and automating routine tasks can reduce operational expenses while maintaining system reliability.
Ultimately, balancing cost and performance requires a holistic approach to TCO analysis. Businesses must consider all cost components, from hardware and labor to data and operations, while aligning these factors with their performance objectives. This ensures that AI investments deliver sustainable value without exceeding budgetary constraints.
TCO analysis remains a cornerstone for effective decision-making in AI infrastructure. By understanding the key cost components—such as infrastructure, labor, data, and operations—businesses can allocate resources wisely and avoid financial pitfalls. Comparing deployment models, including on-premises, cloud-based, and SaaS, further empowers organizations to align their strategies with operational goals. Cost optimization strategies, like leveraging cloud TCO models or refining ai cost analysis, enhance both financial and operational efficiency. Calculating TCO should not be a one-time task but a continuous process, ensuring sustainable AI deployment and long-term success.
FAQ
What is TCO in the context of AI infrastructure?
Total Cost of Ownership (TCO) in AI refers to the comprehensive evaluation of all expenses involved in AI projects. These include costs for model serving, training and tuning, cloud hosting, training data storage, application layers, and operational support. TCO provides a holistic view of financial commitments, ensuring businesses account for both visible and hidden costs.
Why is understanding TCO important for AI and cloud investments?
Understanding TCO enables businesses to anticipate all associated costs, preventing unexpected financial burdens. This insight ensures accurate budgeting, promotes sustainable growth, and enhances operational efficiency. For AI and cloud investments, TCO analysis serves as a critical tool for aligning financial strategies with long-term objectives.
What are the key cost elements to consider in building AI systems?
Building AI systems involves several cost elements, including expenses for data scientists, AI engineers, software developers, project managers, and business analysts. Hardware and specialized expertise also contribute significantly. Recognizing these components helps organizations allocate resources effectively and plan budgets with precision.
Why is measuring the costs and returns of AI implementation crucial for healthcare decision-makers?
Healthcare decision-makers must measure the costs and returns of AI implementation to ensure regulatory compliance and achieve a positive ROI. Comprehensive TCO analyses and healthcare-specific ROI models guide informed decisions, leading to improved patient outcomes and enhanced operational efficiency.
“In healthcare, understanding TCO ensures that AI investments align with both financial goals and patient care priorities.”
What are the main expenses included in the total AI TCO?
The total AI TCO primarily includes infrastructure costs, such as hardware and software setups, and labor costs for engineers and specialists. Tools like AI TCO calculators focus on deployment and operational expenses, excluding additional costs like hardware maintenance or workforce re-engagement.
What does the AI Cloud Total Cost of Ownership model analyze?
The AI Cloud TCO model evaluates various factors, including historical and future GPU rental prices, install base projections, inference and training costs, and GPU throughput optimizations. It provides a detailed analysis of financial implications, helping businesses optimize their cloud-based AI investments.
Why is calculating TCO important for organizations considering the adoption of language models?
Calculating TCO for language models offers organizations valuable insights into the financial impact of implementing these technologies. A reliable TCO calculator aids in budgeting, comparing solutions, and ensuring long-term sustainability. This analysis is essential for organizations aiming to adopt large-scale language models effectively.
What are the various costs associated with implementing AI features in a business?
The costs of implementing AI features vary based on specific use cases and requirements. Key factors driving these expenses include data acquisition, model development, infrastructure, and operational support. Understanding these variables ensures businesses can budget accurately and achieve successful AI integration.
What is the range of costs for AI applications?
AI application costs range widely, from $5,000 for basic models to over $500,000 for advanced, cutting-edge solutions. Effective budgeting requires a thorough understanding of cost components, from initial development to ongoing maintenance, ensuring informed decision-making and resource allocation.
“Investing in AI demands careful financial planning to balance innovation with affordability.”