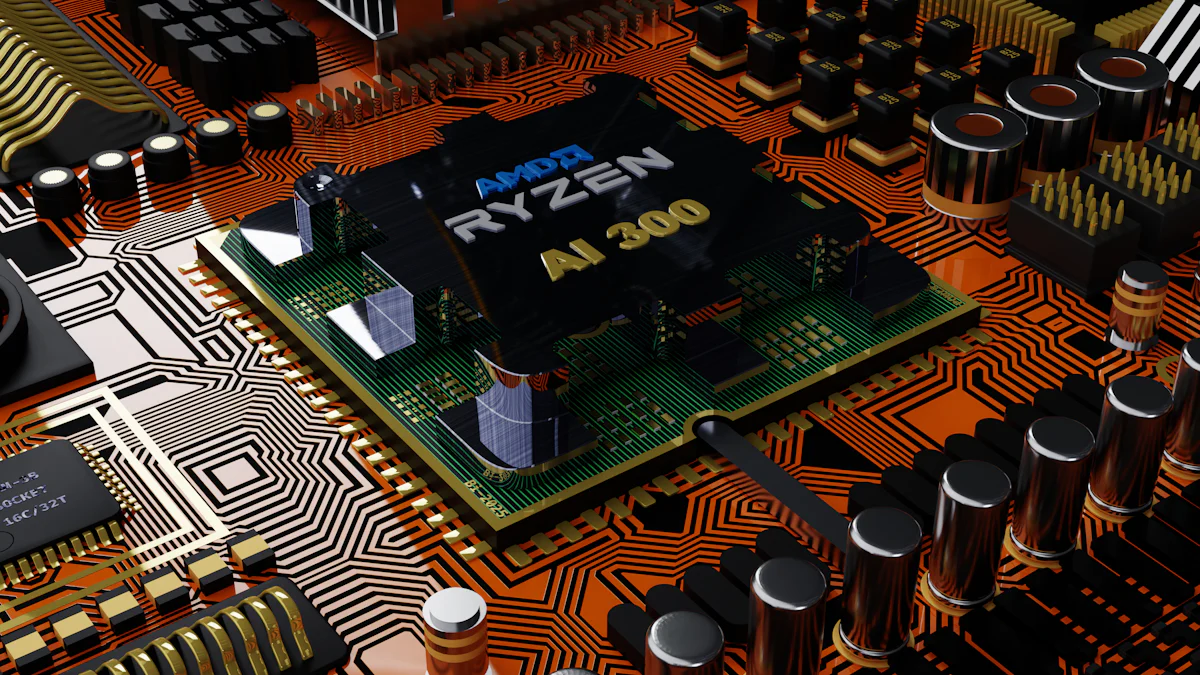
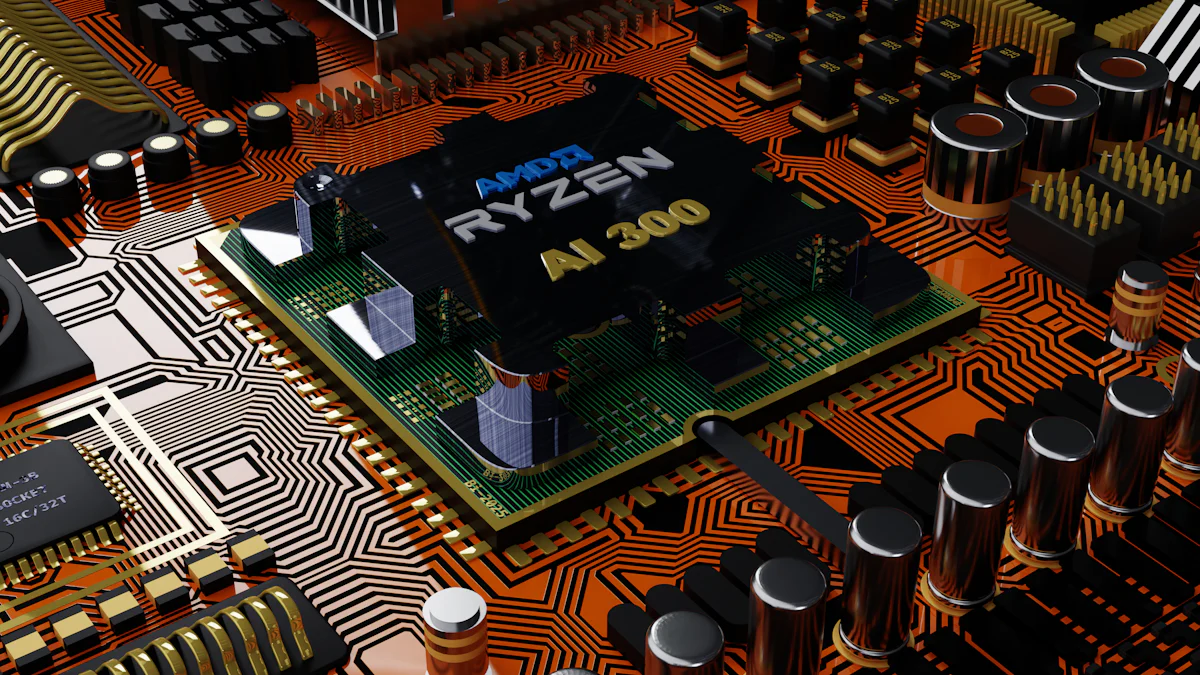
AI workloads demand immense computational power, making power management solutions essential. Training and deploying complex machine learning models consume significant electricity, often exceeding the energy needs of small countries like Denmark, as noted by Schneider Electric. This growing energy consumption impacts global carbon emissions and raises operational costs. You must prioritize energy efficiency to ensure scalability and sustainability. Innovative technologies, such as dynamic power management and energy-efficient hardware, play a crucial role in optimizing power usage. By addressing these challenges, you can balance performance with efficiency while reducing environmental impact.
Key Takeaways
AI workloads require significant energy; optimizing power management is crucial for sustainability and cost-effectiveness.
Adopt energy-efficient hardware like modern GPUs and TPUs to enhance performance while minimizing energy consumption.
Implement software solutions such as power-aware scheduling and dynamic voltage scaling to optimize energy usage in real-time.
Utilize AI-driven algorithms for predictive analytics to forecast energy demands and adjust power distribution proactively.
Integrate renewable energy sources, like solar and wind, to reduce reliance on fossil fuels and lower carbon emissions.
Real-time monitoring tools are essential for tracking energy usage and identifying inefficiencies in datacenter operations.
Collaboration between IT and sustainability teams can align operational goals with environmental objectives, promoting a balanced approach to energy efficiency.
Challenges in Power Management for AI Workloads
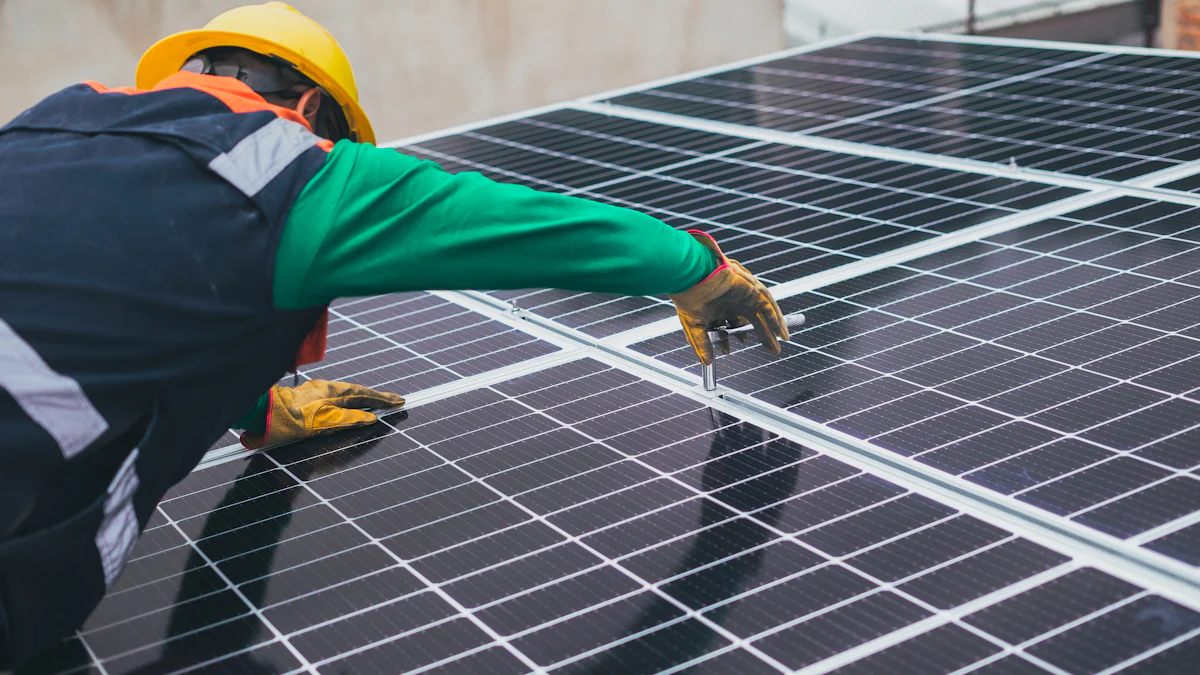
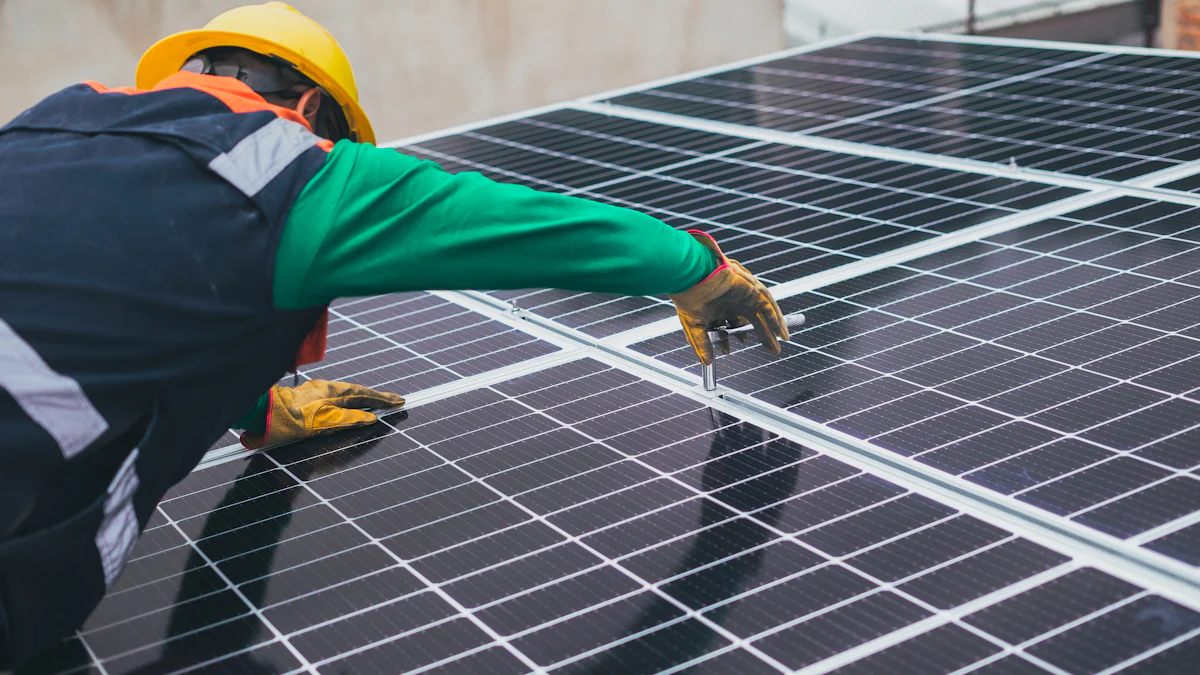
High Energy Demands of AI Models
Training and deploying large AI models require vast computational resources. These processes consume significant amounts of electricity, often surpassing the energy needs of traditional systems. For instance, the power consumption associated with AI workloads has been projected to reach levels comparable to the total energy usage of entire countries, such as Denmark. This highlights the immense energy demands of AI technologies.
The complexity and size of AI models directly influence their energy consumption. As models grow more advanced, their computational requirements increase exponentially. This creates a challenge for maintaining energy efficiency while meeting the performance expectations of modern AI systems. Without proper workload management, these energy demands can lead to unsustainable operational costs and environmental impacts.
Scalability and Sustainability Concerns
Scaling AI deployments introduces additional challenges in balancing performance with energy efficiency. Large-scale AI systems, especially those operating in datacenters, require robust power management strategies to optimize energy usage. Data center energy consumption continues to rise as AI adoption grows, making it essential to address these concerns proactively.
High energy usage in datacenters not only increases operational costs but also contributes to environmental degradation. The carbon emissions resulting from powering these facilities can have long-term ecological consequences. To ensure sustainability, you must adopt innovative solutions that reduce energy waste while maintaining system performance. By prioritizing energy efficiency, you can achieve scalable AI operations without compromising environmental health.
Key Power Management Solutions
Hardware-Based Solutions
Energy-efficient GPUs, TPUs, and custom accelerators for AI workloads.
Selecting the right hardware is critical for improving efficiency in datacenters handling AI workloads. Modern GPUs, TPUs, and custom accelerators are designed to deliver high performance while minimizing energy consumption. For instance, NVIDIA A100, built on the Ampere architecture, offers up to 20 times the speed of its predecessors. Its multi-instance GPU (MIG) feature allows you to allocate resources efficiently across multiple tasks, reducing energy waste. Similarly, NVIDIA T4 provides excellent performance-per-watt, making it ideal for edge AI and cloud computing environments. These advancements ensure that your systems achieve optimal energy efficiency without compromising performance.
Adoption of higher voltage distribution and medium voltage solutions.
Higher voltage distribution systems play a vital role in maintaining power reliability in datacenters. By adopting medium voltage solutions, you can reduce energy losses during transmission and distribution. This approach not only enhances efficiency but also supports the growing power demands of AI workloads. Implementing these systems ensures that your infrastructure remains scalable and sustainable, even as computational requirements increase.
Software-Based Solutions
Power-aware scheduling and dynamic voltage and frequency scaling (DVFS).
Software-based strategies like power-aware scheduling and DVFS are essential for effective workload management. Power-aware scheduling helps you allocate resources based on real-time demand, ensuring that energy is not wasted on idle systems. DVFS adjusts the voltage and frequency of processors dynamically, optimizing power usage during peak and low-demand periods. These techniques enable you to balance performance and energy consumption, making your datacenters more efficient.
AI model optimization techniques to reduce computational overhead.
Optimizing AI models can significantly lower their energy requirements. Techniques such as pruning, quantization, and knowledge distillation reduce the computational complexity of models without sacrificing accuracy. By implementing these methods, you can decrease the energy demands of training and inference processes. This not only improves efficiency but also reduces operational costs, making your AI systems more sustainable.
Hybrid Approaches Combining Hardware and Software
Integration of hardware and software for real-time dynamic power management.
Combining hardware and software solutions offers a comprehensive approach to power management. Real-time dynamic power management systems integrate advanced hardware with intelligent software to monitor and adjust energy usage continuously. For example, NVIDIA GB200 Grace Blackwell Superchip demonstrates exceptional energy efficiency, achieving 25 times the efficiency of previous generations. Such integration ensures that your systems operate at peak performance while minimizing energy consumption.
Use of AI-driven algorithms to optimize energy consumption in datacenters.
AI-driven algorithms are transforming how datacenters manage energy. These algorithms analyze patterns in energy usage and predict future demands, enabling proactive adjustments to power distribution. By leveraging machine learning, you can optimize energy consumption, enhance power reliability, and reduce environmental impact. This innovative approach ensures that your datacenters remain efficient and sustainable in the face of increasing workloads.
Emerging Trends and Innovations
AI-Driven Power Management Systems
Predictive analytics and machine learning for energy optimization.
AI-driven power management systems are revolutionizing how you handle energy demands in datacenters. Predictive analytics, powered by machine learning, enables you to forecast energy usage with remarkable accuracy. These systems analyze historical data and identify patterns to predict future energy needs. By doing so, you can allocate resources more effectively and prevent energy waste.
Machine learning algorithms also allow real-time adjustments to energy consumption. For example, they can detect inefficiencies in your datacenters and recommend immediate corrective actions. This dynamic approach ensures that your operations remain efficient, even during peak workloads. Studies have shown that AI techniques significantly improve energy efficiency while reducing operational costs. By adopting these systems, you can optimize energy usage and enhance the sustainability of your AI workloads.
Adaptive systems that adjust power consumption based on workload demands.
Adaptive power management systems take energy optimization a step further. These systems continuously monitor workload demands and adjust power consumption accordingly. When workloads increase, the system allocates additional energy to maintain performance. Conversely, during low-demand periods, it reduces energy usage to conserve resources.
This adaptability is particularly beneficial for datacenters handling fluctuating AI workloads. It ensures that you achieve maximum efficiency without compromising performance. Research highlights the role of adaptive systems in promoting sustainability and reducing environmental impact. By integrating these technologies, you can create a more resilient and energy-efficient infrastructure.
Renewable Energy Integration for AI Workloads
Leveraging solar, wind, and other renewable sources to power AI operations.
Renewable energy sources are becoming a cornerstone of sustainable AI operations. Solar panels, wind turbines, and other renewable technologies provide clean and reliable energy for datacenters. By incorporating these sources, you can reduce your reliance on fossil fuels and lower carbon emissions.
For instance, many organizations are now using solar farms to power their AI workloads. Wind energy is another popular choice, especially in regions with consistent wind patterns. These renewable solutions not only support environmental goals but also help you achieve long-term cost savings. By leveraging renewable energy, you can align your operations with global sustainability initiatives while maintaining efficiency.
Energy storage solutions for consistent and sustainable power supply.
Energy storage systems play a critical role in ensuring a steady power supply for AI workloads. Technologies like lithium-ion batteries and advanced energy storage solutions store excess energy generated by renewable sources. This stored energy can be used during periods of high demand or when renewable generation is low.
For example, a datacenter powered by solar energy can use battery storage to maintain operations during cloudy days or at night. This approach ensures that your AI workloads run smoothly without interruptions. Energy storage solutions also enhance grid stability and improve overall efficiency. By adopting these systems, you can create a more sustainable and reliable energy infrastructure for your datacenters.
Best Practices for Implementing Power Management Solutions
Monitoring and Analytics Tools
Real-time monitoring tools for tracking energy usage and system health.
Real-time monitoring tools are essential for maintaining the efficiency of your datacenter operations. These tools provide continuous insights into energy usage, helping you track power consumption across various systems. By using these tools, you can identify patterns in energy demand and adjust resources accordingly. For example, advanced monitoring systems can alert you to spikes in power usage, allowing you to take immediate action to prevent waste.
The integration of artificial intelligence (AI) into monitoring tools has further enhanced their capabilities. AI-driven algorithms analyze data in real time, enabling dynamic adjustments to power usage. A recent survey highlighted the importance of predictive analytics in optimizing energy consumption. These systems not only improve efficiency but also ensure the sustainability of your operations. By adopting real-time monitoring, you can maintain system health and reduce unnecessary energy expenditure.
Identifying inefficiencies and optimizing resource utilization.
Identifying inefficiencies is a critical step in improving power management solutions. Inefficient systems often consume more energy than necessary, leading to higher costs and environmental impact. Monitoring tools help you pinpoint these inefficiencies by providing detailed reports on energy usage. For instance, they can reveal underutilized servers or outdated hardware that drains power without contributing to performance.
Once inefficiencies are identified, you can optimize resource utilization to enhance efficiency. AI-powered tools play a significant role in this process. They recommend specific actions, such as redistributing workloads or upgrading hardware, to maximize energy savings. By addressing inefficiencies, you can create a more sustainable and cost-effective infrastructure for your datacenters.
Collaboration Between IT and Sustainability Teams
Aligning IT operations with sustainability goals for energy efficiency.
Collaboration between IT and sustainability teams is vital for achieving energy efficiency in datacenters. IT teams focus on maintaining performance, while sustainability teams prioritize reducing environmental impact. By working together, these teams can align their goals to create a balanced approach to power management.
For example, IT teams can implement energy-efficient hardware and software solutions, while sustainability teams monitor their impact on carbon emissions. This partnership ensures that your datacenter operations remain efficient without compromising environmental objectives. A unified strategy helps you meet both performance and sustainability targets, making your operations more resilient and future-ready.
Developing strategies to reduce carbon footprint while maintaining performance.
Reducing your carbon footprint requires a strategic approach that balances energy efficiency with performance. Start by evaluating the energy sources powering your datacenters. Transitioning to renewable energy, such as solar or wind, can significantly lower emissions. Additionally, energy storage solutions ensure a consistent power supply, even during periods of low renewable generation.
AI-driven systems also contribute to carbon reduction by optimizing energy usage. These systems analyze workload demands and adjust power consumption in real time, minimizing waste. A survey on AI integration revealed its potential to enhance efficiency across sectors, including datacenters. By leveraging these technologies, you can maintain high performance while reducing your environmental impact.
Addressing power management challenges in AI workloads is essential for ensuring efficiency and sustainability. By adopting hardware, software, and hybrid solutions, you can optimize energy usage, reduce costs, and enhance the performance of your systems. Modern GPUs and AI-driven algorithms enable datacenters to operate with greater precision and lower energy waste. Emerging trends, such as renewable energy integration and real-time monitoring tools, further support sustainable operations. Implementing these best practices empowers you to scale AI workloads effectively while minimizing environmental impact and achieving long-term operational success.
FAQ
How much energy does AI use?
AI workloads consume significant amounts of energy due to their computational intensity. Training and deploying advanced AI models often require substantial power. However, the efficiency these models bring to various processes can result in net energy savings over time.
What is the challenge related to AI energy consumption?
The primary challenge lies in the increasing energy demands needed to sustain AI operations. As AI systems grow more complex, their energy requirements rise, impacting resource utilization and operational costs. Understanding these demands is crucial for managing their consequences effectively.
What are the benefits of selecting an energy-efficient GPU for AI?
Energy-efficient GPUs play a vital role in maintaining performance while reducing power usage. They help prevent overheating, minimize strain on power systems, and extend hardware lifespan by addressing thermal issues. Choosing efficient GPUs ensures optimal energy utilization without compromising computational capabilities.
Why is power optimization important in the context of AI and energy consumption?
Power optimization ensures that energy resources are used effectively, reducing waste and improving efficiency. AI-driven algorithms and predictive analytics enable real-time monitoring and dynamic adjustments to meet energy demands. This approach supports sustainable operations and lowers energy costs.
What is the common usage of AI in energy management?
AI is widely used to optimize energy production, distribution, and consumption. Machine learning and other technologies enhance efficiency, reduce waste, and promote the use of renewable energy. These advancements also help lower costs and improve overall energy management systems.
What metric is used to evaluate overall energy efficiency in data centers?
Data centers commonly use the Power Usage Effectiveness (PUE) metric to measure energy efficiency. A lower PUE indicates better efficiency, as it reflects a higher proportion of energy being used directly for computing rather than for supporting infrastructure like cooling systems.
What are the main reasons for power optimization in AI workloads?
Power optimization reduces environmental impact, lowers operational costs, and ensures a stable energy supply for future needs. By prioritizing energy efficiency, you contribute to sustainability while maintaining the performance of AI systems.
How can renewable energy support AI workloads?
Renewable energy sources like solar and wind provide clean and sustainable power for AI operations. Integrating these sources reduces reliance on fossil fuels and lowers carbon emissions. Energy storage solutions, such as batteries, ensure consistent power supply even during periods of low renewable generation.
What role does AI play in improving energy efficiency?
AI enhances energy efficiency by analyzing usage patterns and predicting future demands. Machine learning algorithms enable real-time adjustments to power consumption, optimizing energy distribution and reducing waste. This technology ensures that energy resources are utilized effectively.
How can you monitor energy usage in data centers?
Real-time monitoring tools track energy consumption and system health across data centers. These tools identify inefficiencies and provide actionable insights to optimize resource utilization. AI-powered monitoring systems further enhance this process by enabling dynamic adjustments and predictive analytics.