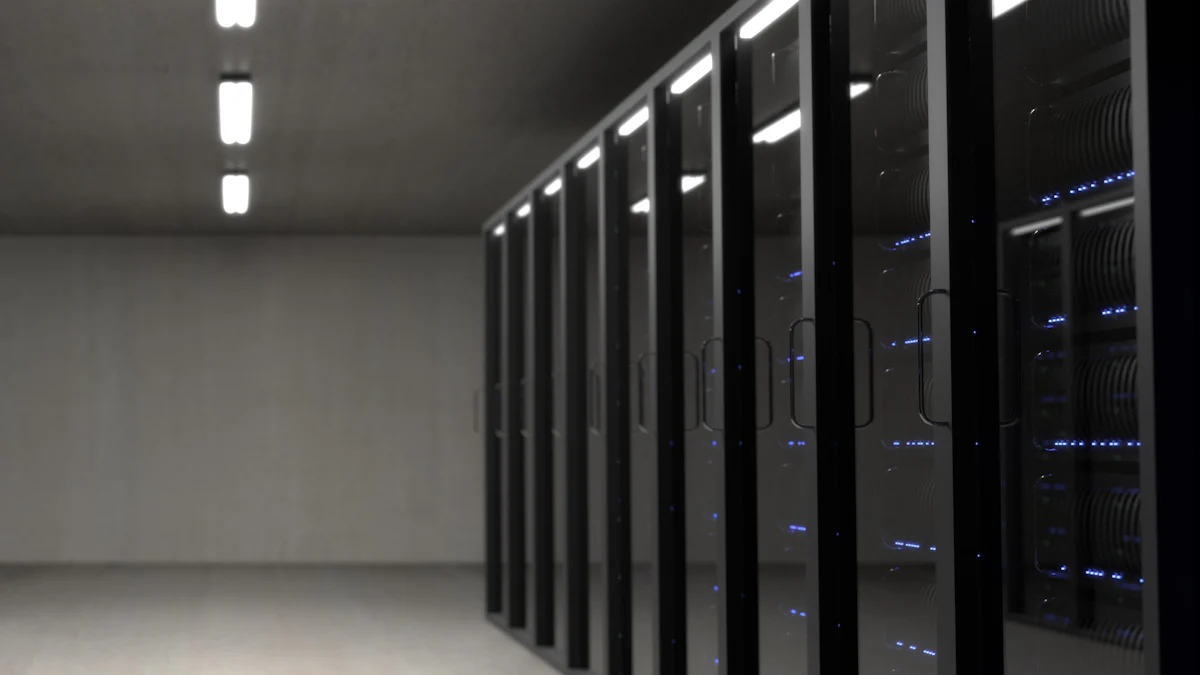
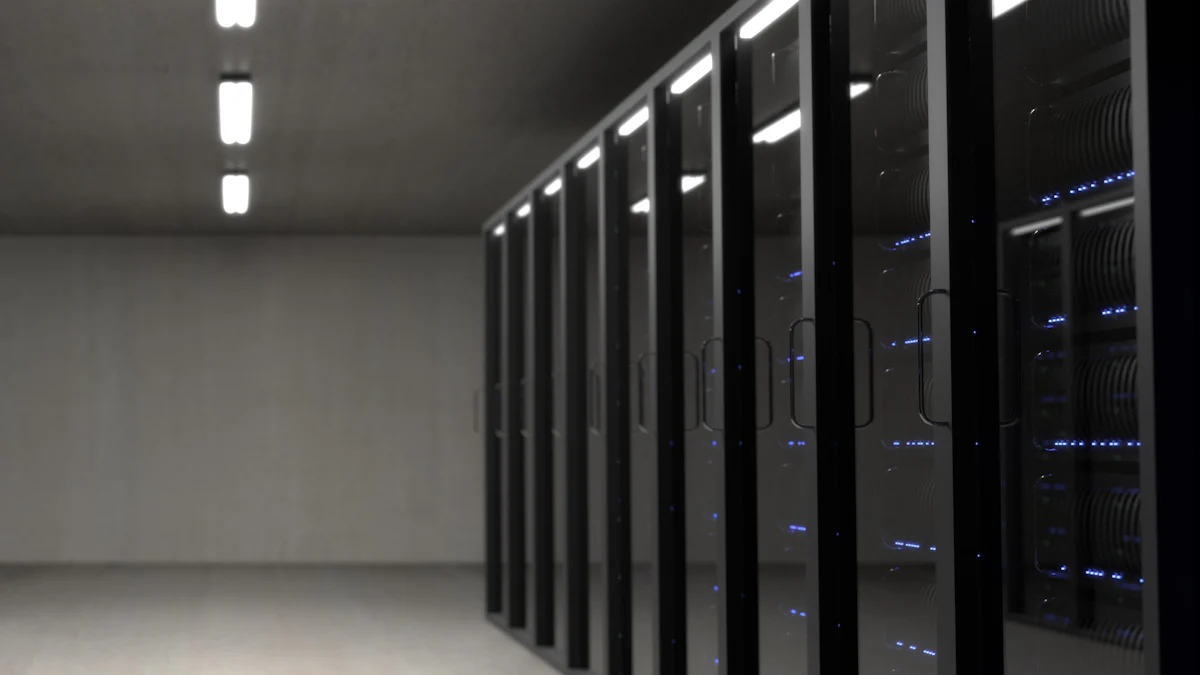
Data center power use is set to undergo significant changes in the coming years. The rise of artificial intelligence (AI) and cryptocurrency mining has led to a surge in energy consumption. These technologies demand substantial computational power, driving data centers to consume up to 8% of all electricity worldwide by 2030. This increase poses challenges for sustainability efforts, as data centers already account for 4% of global energy consumption and contribute to 1% of global greenhouse emissions. Addressing these challenges requires innovative solutions to balance technological growth with environmental responsibility.
Current Trends and Projections in Data Center Power Consumption
The Role of AI in Increasing Energy Demand
Artificial intelligence (AI) significantly impacts data center power use. As AI technologies advance, they require more computational power, leading to increased electricity consumption. AI’s Impact on Data Center Energy Consumption reveals that AI innovations will boost computing speed faster than they ramp up electricity use. However, the widespread adoption of AI still increases power consumption. By 2028, AI is expected to account for about 19% of data center power demand. This growth highlights the need for efficient energy management strategies to accommodate AI’s expanding role in data centers.
Cryptocurrency Mining and Its Energy Implications
Cryptocurrency mining also contributes to rising data center power use. The process of mining cryptocurrencies involves solving complex mathematical problems, which demands substantial computational resources. According to the IEA Report on Data Center Energy Consumption, data center energy consumption could double by 2026, reaching 1,000 TWh, largely driven by cryptocurrency mining. This surge in energy demand underscores the importance of developing sustainable practices to mitigate the environmental impact of cryptocurrency operations.
General Growth in Data Center Usage
The general increase in data center usage further amplifies energy consumption. As more businesses and individuals rely on digital services, data centers must expand their capacity to meet this demand. The Data Center Power Supply and Energy Consumption Trends study indicates that data centers rank among the largest consumers of electrical power. This trend accelerates alongside technological advancements, particularly AI workloads and increasing online activity. By 2030, the number of data centers is expected to exceed 24,000 sites, further straining energy resources.
Environmental Impact of Data Center Energy Use
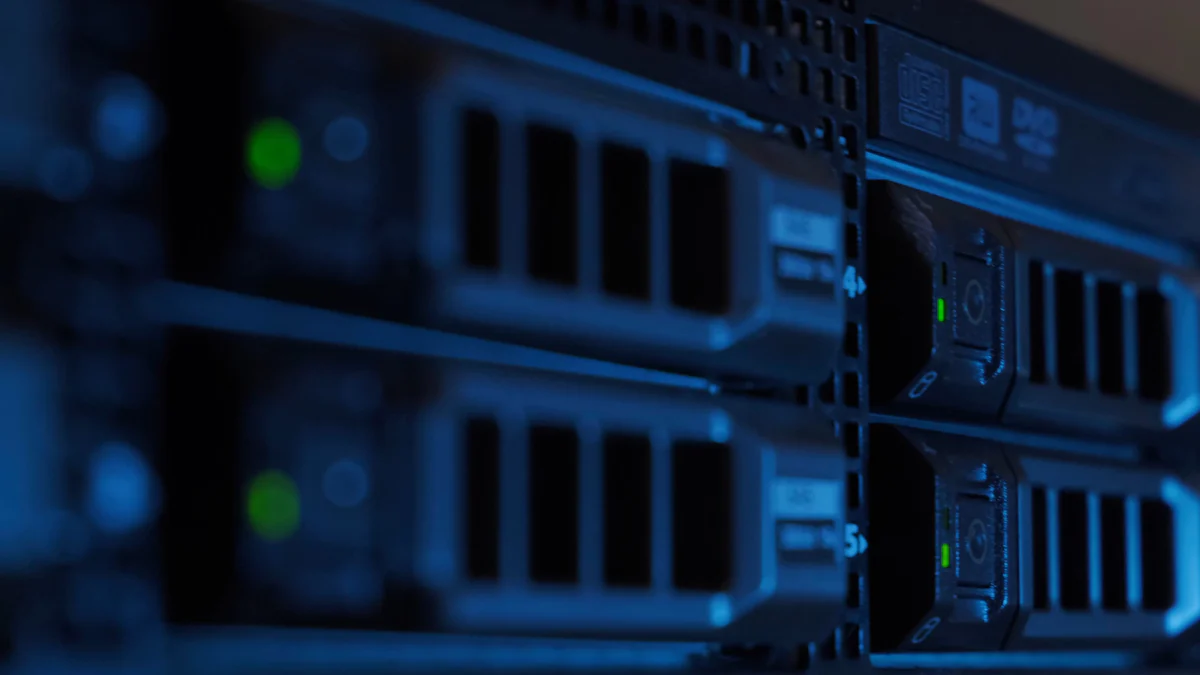
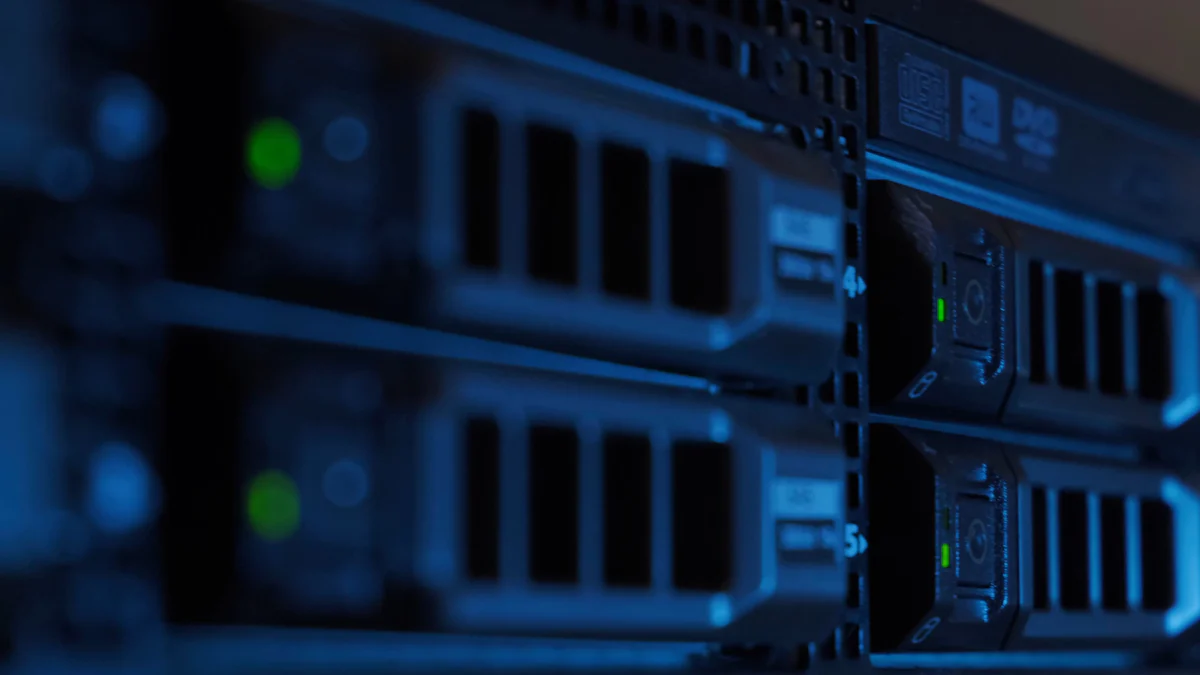
Carbon Emissions and Climate Change
Data centers significantly contribute to carbon emissions, impacting climate change. They consume around 2% of all electricity worldwide, which raises concerns about their environmental footprint. These facilities account for approximately 3% of global energy consumption and over 1% of greenhouse gas emissions. As data centers expand, their carbon footprint grows, posing challenges for climate change mitigation efforts. The energy-intensive nature of data centers, consuming 10 to 50 times the energy per floor space compared to typical commercial buildings, exacerbates this issue. Addressing these emissions requires innovative solutions and a commitment to reducing carbon output.
Balancing Growth with Sustainability
Balancing the growth of data centers with sustainability presents a complex challenge. As data centers could consume up to 9% of U.S. electricity generation by 2030, the need for sustainable practices becomes more pressing. The anticipated growth in global data center power requirements necessitates a focus on green technologies. Pioneering efforts in energy efficiency and renewable energy adoption can help mitigate the environmental impact. Data centers must prioritize sustainability to ensure that technological advancements do not come at the expense of the environment. By investing in energy-efficient infrastructure and exploring renewable energy sources, data centers can achieve a balance between growth and environmental responsibility.
Strategies for Improving Energy Efficiency
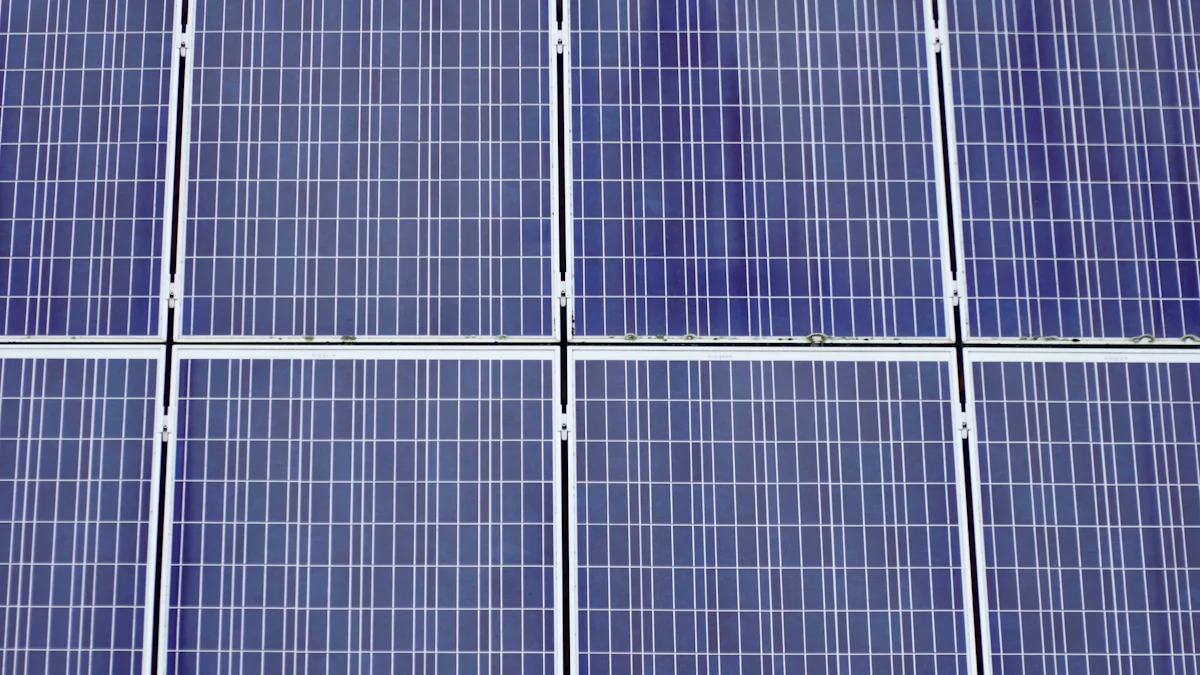
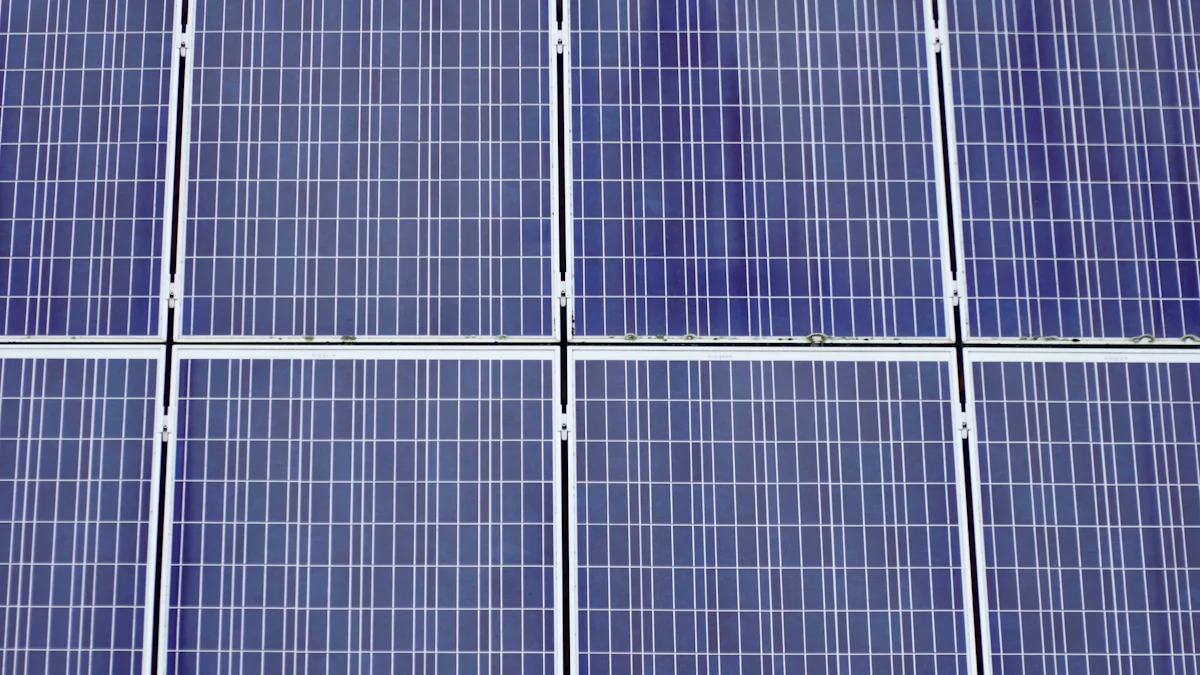
Innovations in Cooling Systems
Data centers constantly seek innovative cooling solutions to enhance energy efficiency. Liquid and Immersion Cooling methods have gained popularity. These techniques involve submerging components in a liquid medium, effectively dissipating heat. This approach not only saves energy but also improves cooling effectiveness. Additionally, Direct Liquid Cooling (DLC) circulates liquid directly in contact with electronic components, offering significant energy-saving advantages.
Efficient cooling systems, such as cold and hot aisles, play a crucial role in maximizing energy efficiency. By separating cold and hot airflows, these systems maintain optimal temperatures while minimizing energy usage. Free air cooling and evaporative cooling further contribute to energy savings by utilizing natural air and water evaporation processes. Implementing green cooling technologies, like water-side economization, significantly reduces energy consumption while optimizing cooling effectiveness.
Enhancing Resource Utilization
Enhancing resource utilization in data centers involves optimizing the use of available resources to achieve maximum efficiency. Data centers can implement virtualization technologies to consolidate workloads onto fewer servers, reducing energy consumption. By utilizing cloud computing, data centers can dynamically allocate resources based on demand, ensuring efficient use of energy.
Energy management systems play a vital role in monitoring and controlling energy usage. These systems provide real-time data on energy consumption, enabling data centers to identify areas for improvement. By analyzing this data, data centers can implement strategies to optimize resource utilization and reduce energy waste.
Adoption of Energy-Efficient Equipment
Adopting energy-efficient equipment is essential for reducing power consumption in data centers. Energy-efficient servers and storage devices consume less electricity while delivering high performance. These devices incorporate advanced technologies that optimize power usage, resulting in significant energy savings.
Precision air conditioning systems help maintain optimal temperatures in data centers while minimizing energy usage. These systems provide targeted cooling to specific areas, ensuring efficient energy utilization. Additionally, hot/cold aisle containment techniques further enhance cooling efficiency by preventing the mixing of hot and cold air.
Data centers can also benefit from advanced cooling systems that continually refine design and management practices. By implementing these systems, data centers can reduce energy needs and improve overall energy efficiency.
Data centers face a future of increasing energy demands driven by AI and cryptocurrency. This growth necessitates a focus on sustainable practices. Operators must integrate sustainability into their core strategies, balancing environmental, societal, and economic factors. By adopting energy-efficient technologies and innovative cooling systems, data centers can reduce their carbon footprint. This commitment not only enhances their reputation but also strengthens stakeholder trust. As organizations prioritize sustainability, they gain a competitive edge and prepare for future environmental challenges. Collaboration and innovation will be key in addressing these energy challenges effectively.
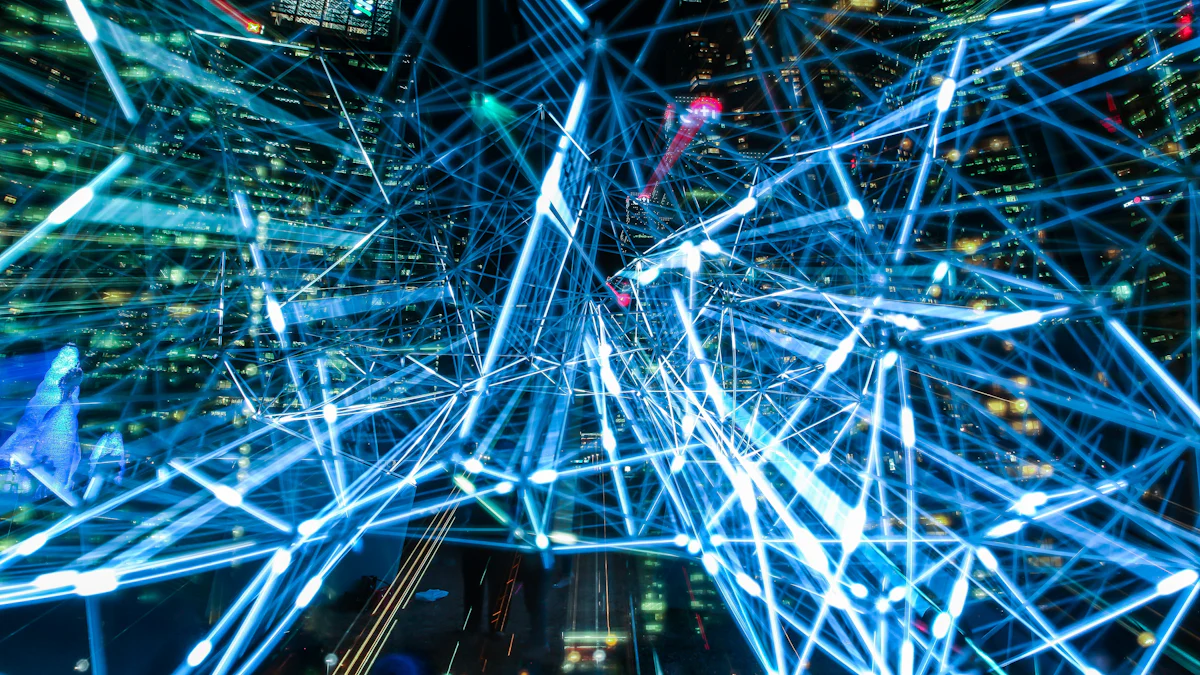
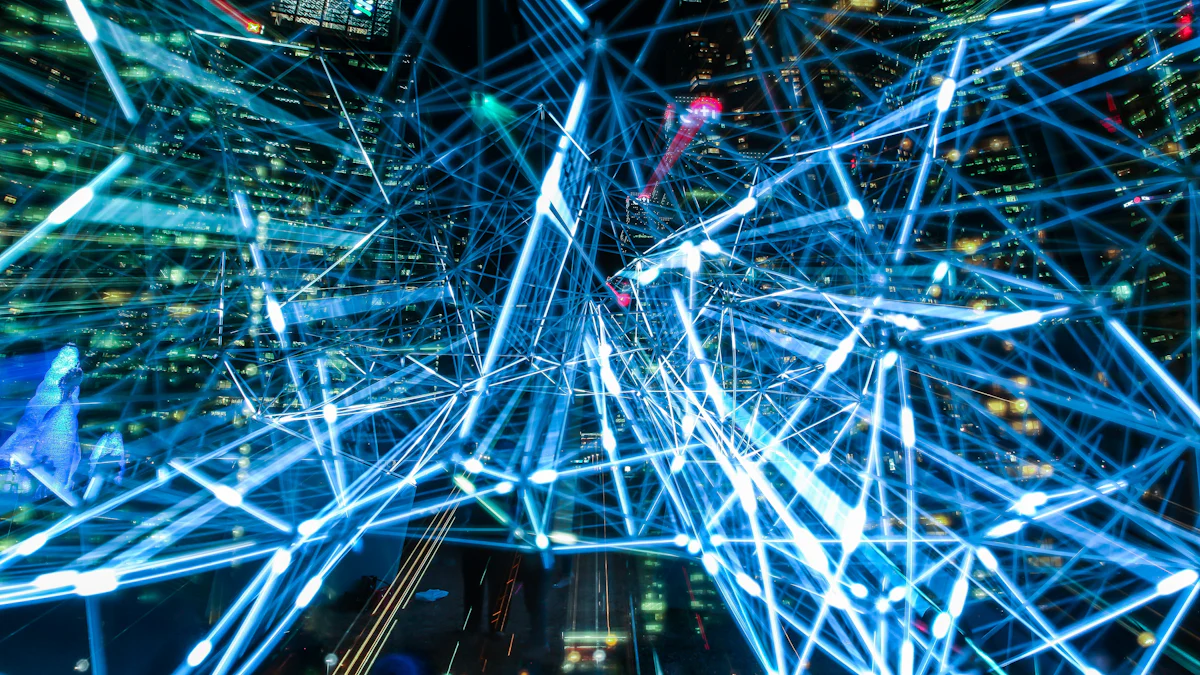
Cloud computing has transformed data center operations by providing on-demand access to computing resources. This shift enhances flexibility and scalability, allowing businesses to adapt quickly to changing demands. Companies increasingly rely on cloud solutions, with 98% of corporations storing some data in the cloud. This trend optimizes resource management, leading to improved efficiency and reduced operational costs. Notably, 82% of companies report cost savings and efficiency boosts from cloud adoption. Cloud data centers host remote servers that manage data, applications, and services, which are crucial for modern business operations.
The Evolution of Data Centers with Cloud Computing
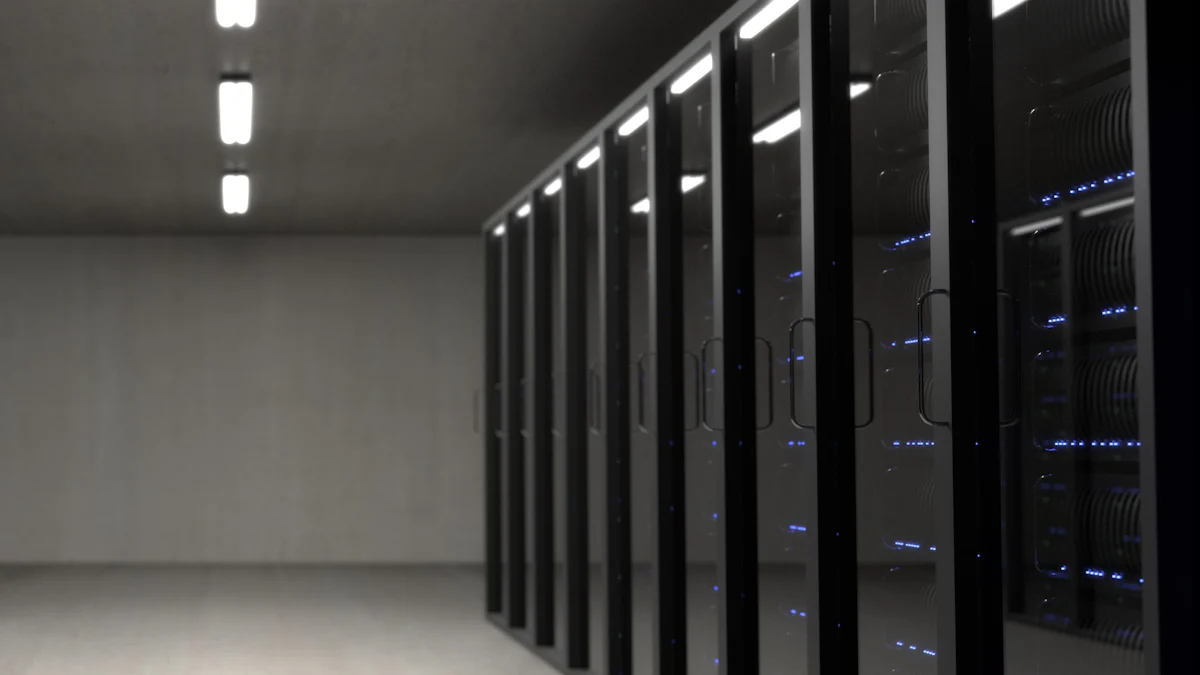
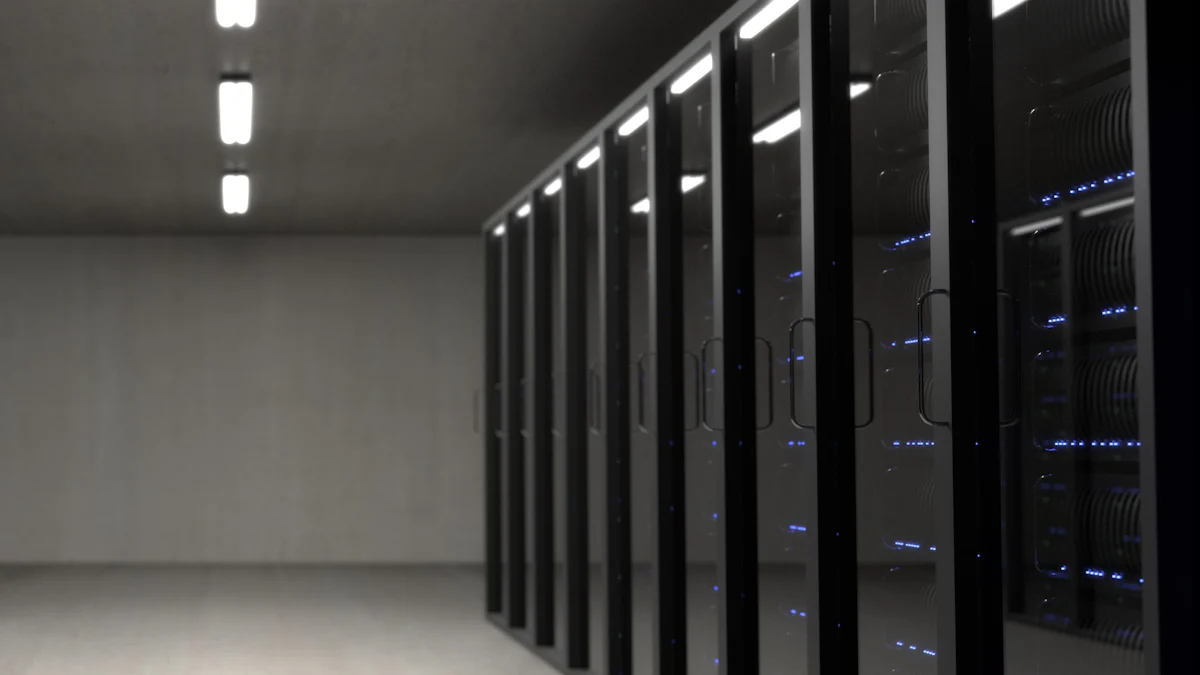
From Traditional to Cloud-Based Models
Data centers have undergone a remarkable transformation over the years. In the past, companies relied on traditional data centers, which housed physical infrastructure in a central location. This setup allowed businesses to access their resources from anywhere. However, the rise of the internet and advancements in computing technology during the 1990s marked a turning point. The dot-com boom spurred significant changes, leading to the development of more sophisticated data management systems.
Today, cloud computing has revolutionized the way organizations think about data centers. Many businesses have shifted their data and applications to the cloud, embracing a more distributed and hybrid approach. This shift has reached a level of maturity that benefits both large enterprises and small businesses. Cloud data centers primarily use a Software-as-a-Service (SaaS) model, enabling companies to provision computational resources without the need for extensive IT teams or costly hardware. As a result, about one-third of data now passes through the cloud daily, with this figure growing rapidly.
Virtualization and Automation
Virtualization and automation have played pivotal roles in the evolution of data centers. Virtualization allows multiple virtual machines to run on a single physical server, optimizing resource utilization and reducing costs. This technology has become a cornerstone of modern data center operations, enabling businesses to scale their resources efficiently.
Automation further enhances the capabilities of cloud-based data centers. By automating routine tasks, companies can streamline operations and improve efficiency. Automation tools manage everything from resource allocation to security updates, freeing up IT staff to focus on more strategic initiatives. This approach not only reduces operational costs but also minimizes the risk of human error.
Benefits of Cloud Computing for Data Centers
Cost Efficiency
Cloud computing offers significant cost advantages for data centers. Traditional data centers often require substantial investments in hardware, infrastructure, and maintenance. These costs can quickly escalate as businesses grow and demand increases. In contrast, cloud data centers provide a more cost-effective solution. Companies can access storage, network, and IT infrastructure without the need for large upfront expenditures. This model allows businesses to pay only for the resources they use, reducing unnecessary expenses.
Moreover, cloud services eliminate the need for constant hardware upgrades and maintenance. Third-party providers manage these tasks, freeing companies from the burden of ongoing upkeep. This approach not only lowers operational costs but also minimizes the risk of unexpected expenses. By leveraging cloud solutions, businesses can allocate their financial resources more strategically, investing in areas that drive growth and innovation.
Scalability and Flexibility
Scalability stands as a hallmark of cloud computing. Traditional data centers often struggle to adapt to fluctuating demands. Scaling up requires new planning, additional hardware, and sometimes even software purchases. This process can be time-consuming and costly. Cloud data centers, however, offer unparalleled scalability. Businesses can easily adjust their resource capacity based on current needs, ensuring optimal performance without overcommitting resources.
This agility empowers businesses to innovate and experiment without the constraints of rigid infrastructure. As a result, cloud solutions support a dynamic and responsive approach to data management, positioning organizations to thrive in an ever-evolving digital landscape.
Challenges and Considerations
Security and Compliance
Security remains a top concern for organizations adopting cloud solutions. Companies must ensure that their data stays protected from unauthorized access and breaches. Cloud providers implement robust security measures, including encryption and multi-factor authentication, to safeguard sensitive information. However, businesses must also take responsibility for securing their data. They should establish strong access controls and regularly update security protocols.
Compliance with industry regulations presents another challenge. Different sectors have specific requirements for data protection and privacy. Organizations must ensure that their cloud solutions align with these standards. Failure to comply can result in legal penalties and damage to reputation. Companies should work closely with cloud providers to understand compliance obligations and implement necessary measures.
Potential Drawbacks
Despite its advantages, cloud computing has potential drawbacks. One concern is the reliance on internet connectivity. Businesses need a stable and fast internet connection to access cloud services. Any disruption can impact operations and productivity. Companies should have contingency plans in place to mitigate this risk.
Another consideration is the potential for vendor lock-in. Organizations may find it challenging to switch providers once they commit to a specific cloud platform. This situation can limit flexibility and increase costs over time. Businesses should evaluate their options carefully and consider multi-cloud strategies to avoid dependency on a single provider.
Future Trends in Cloud and Data Center Integration
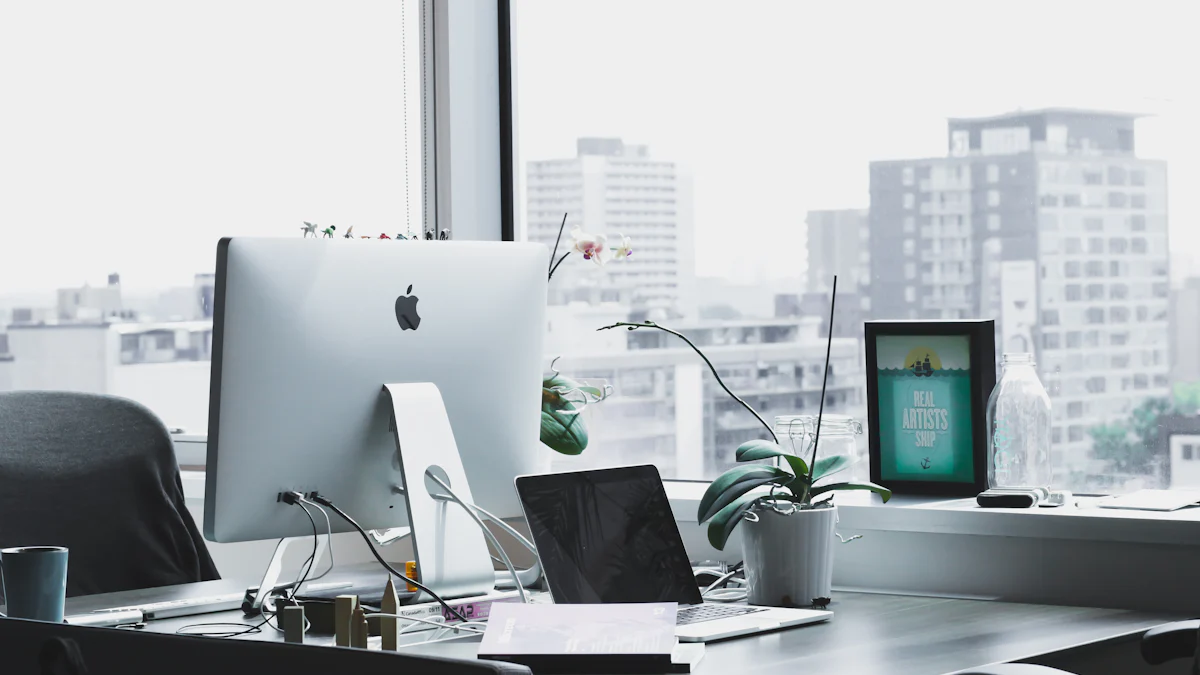
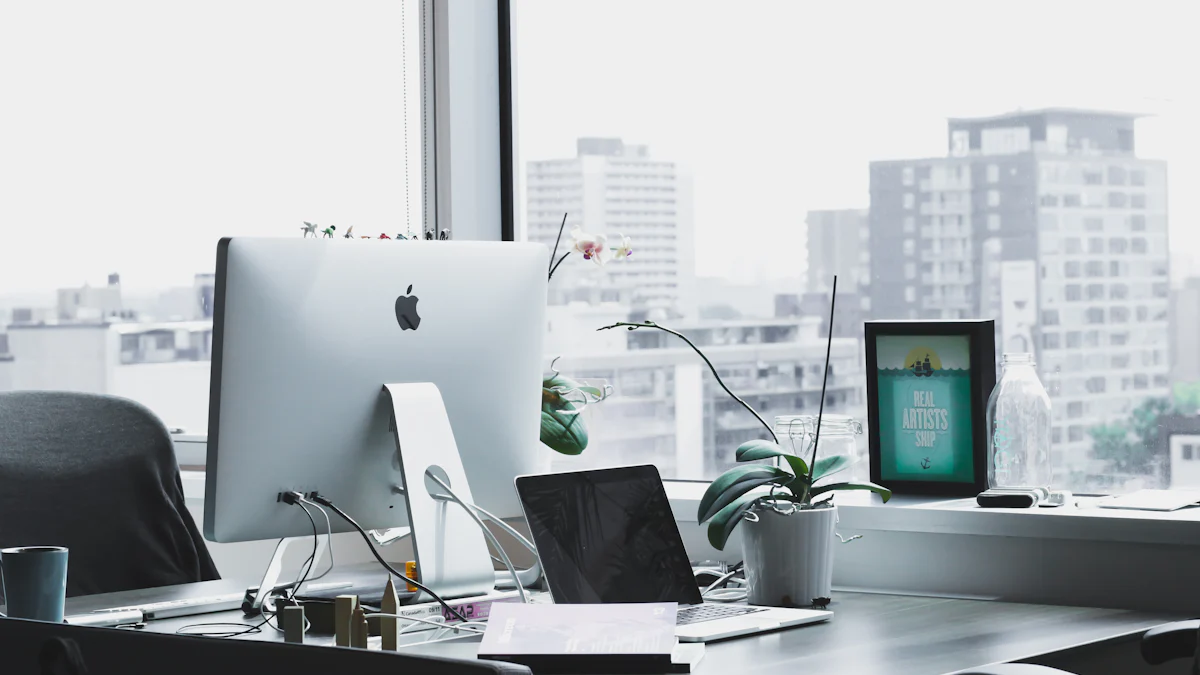
Emerging Technologies
Emerging technologies are reshaping the landscape of data centers. These innovations promise to enhance efficiency, performance, and sustainability. The Integration of Emerging Technologies in Data Centers highlights several key advancements. Artificial intelligence (AI) and machine learning (ML) are at the forefront. They optimize data processing and automate routine tasks. AI-driven analytics provide insights into system performance, enabling proactive maintenance and reducing downtime.
Edge computing represents another significant trend. It brings computation and data storage closer to the location where it is needed. This reduces latency and improves response times. Edge computing supports real-time applications, such as autonomous vehicles and smart cities. As a result, data centers must adapt to accommodate these decentralized models.
Blockchain technology also plays a role in future data center operations. It offers secure and transparent data management solutions. Blockchain enhances data integrity and reduces the risk of unauthorized access. This technology becomes increasingly important as data privacy concerns grow.
Strategic Approaches
Organizations must adopt strategic approaches to integrate cloud technologies effectively. Industry research from The Future of Data Centers: Transformation with Cloud Integration indicates a major shift toward cloud integration. Companies leverage cloud solutions to augment traditional IT infrastructure. This strategy improves efficiency and achieves cost savings.
A hybrid cloud model offers a balanced approach. It combines private and public cloud resources. This model provides flexibility and scalability while maintaining control over sensitive data. Businesses can choose the best environment for each workload, optimizing performance and cost.
Multi-cloud strategies also gain popularity. They involve using multiple cloud providers to avoid vendor lock-in. This approach increases resilience and allows organizations to select the best services from different providers. Companies can tailor their cloud environments to meet specific needs, enhancing agility and innovation.
Data Center Definition and Importance
Understanding Data Center Tiers
Data centers serve as the backbone of modern digital infrastructure. They house the critical systems and components that store, process, and disseminate data. The Data Center Definition encompasses a wide range of facilities, from small server rooms to large-scale operations that support global networks. Understanding the structure and classification of data centers is essential for businesses aiming to optimize their IT resources.
Data Center Tiers provide a standardized method to evaluate the performance and reliability of these facilities. The Uptime Institute developed this tier system, which categorizes data centers into four distinct levels:
Tier I: Basic capacity with limited protection against physical events. Suitable for small businesses with minimal IT requirements.
Tier II: Redundant capacity components offer improved protection. Ideal for companies needing more reliable infrastructure.
Tier III: Concurrently maintainable infrastructure ensures higher availability. This tier supports businesses with critical operations that cannot afford downtime.
Tier IV: Fault-tolerant systems provide the highest level of reliability. Designed for enterprises requiring continuous operation and zero downtime.
Each tier represents a different level of redundancy and fault tolerance, allowing organizations to choose the appropriate level based on their operational needs and budget constraints.
The Role of Data Center Virtualization
Data Center Virtualization plays a pivotal role in transforming traditional IT environments into more agile and efficient systems. By leveraging virtualization technologies, businesses can create multiple virtual versions of physical infrastructure within a data center. This approach optimizes resource utilization and reduces operational costs.
Virtualization enables the consolidation of computing resources, allowing multiple virtual machines (VMs) to run on a single physical server. This setup enhances resource efficiency and minimizes the need for additional hardware. Virtualization Servers utilize high-speed Ethernet connections to manage network traffic effectively, ensuring seamless communication within the data center.
The benefits of data center virtualization extend beyond cost savings. It improves scalability by allowing businesses to quickly adjust their IT resources in response to changing demands. Virtualization also enhances security through isolation, as each virtual environment operates independently. This separation reduces the risk of unauthorized access and data breaches.
Moreover, virtualization simplifies administrative tasks by providing centralized management tools. These tools offer visibility and control over the entire virtualized infrastructure, streamlining operations and improving overall efficiency. As a result, data center virtualization aligns IT infrastructure with business objectives, enabling companies to function more effectively in a competitive landscape.
Data Center Security and Related Concerns
Data center security remains a critical aspect of modern business operations. Organizations must protect their data from unauthorized access and potential breaches. As cloud computing becomes more prevalent, companies face new challenges in maintaining robust security measures. This section explores the essential security protocols and measures that ensure data center safety.
Cloud Security Measures
Cloud security measures play a vital role in safeguarding data stored in cloud environments. Providers implement various strategies to protect sensitive information. Encryption stands as a primary defense mechanism. It converts data into a coded format, making it unreadable to unauthorized users. Multi-factor authentication adds an extra layer of security by requiring users to verify their identity through multiple methods.
Providers also conduct regular security audits to identify vulnerabilities. These assessments help in updating security protocols and ensuring compliance with industry standards. Companies should collaborate with their cloud providers to understand the security measures in place. This partnership ensures that both parties work together to protect data effectively.
Data center security protocols encompass a range of practices designed to protect physical and digital assets. Data center security protocols include access control systems that restrict entry to authorized personnel only. These systems often include biometric scanners and keycard access to enhance security. Surveillance cameras monitor activities within the data center, providing real-time footage for security personnel.
Data center security protocols encompass a range of practices designed to protect physical and digital assets. Access control systems restrict entry to authorized personnel only. These systems often include biometric scanners and keycard access to enhance security. Surveillance cameras monitor activities within the data center, providing real-time footage for security personnel.
Firewalls and intrusion detection systems form the backbone of digital security. They prevent unauthorized access to the network and detect suspicious activities. Regular software updates and patches keep systems secure from emerging threats. Data centers also implement disaster recovery plans to ensure data availability during unforeseen events. These plans outline procedures for data backup and restoration, minimizing downtime and data loss.
Cloud computing has revolutionized data center operations by offering scalable, efficient, and flexible solutions. Businesses can now easily scale their operations without the burden of physical storage and server maintenance. This transformation enhances operational efficiency and cost-effectiveness. Despite challenges, the integration of cloud technologies will drive future innovations in data center management. Organizations must adapt to these changes to remain competitive and fully leverage cloud computing’s potential. The future of data centers lies in embracing cloud solutions to enhance sustainability and operational efficiency, ensuring they meet the demands of an ever-evolving digital landscape.
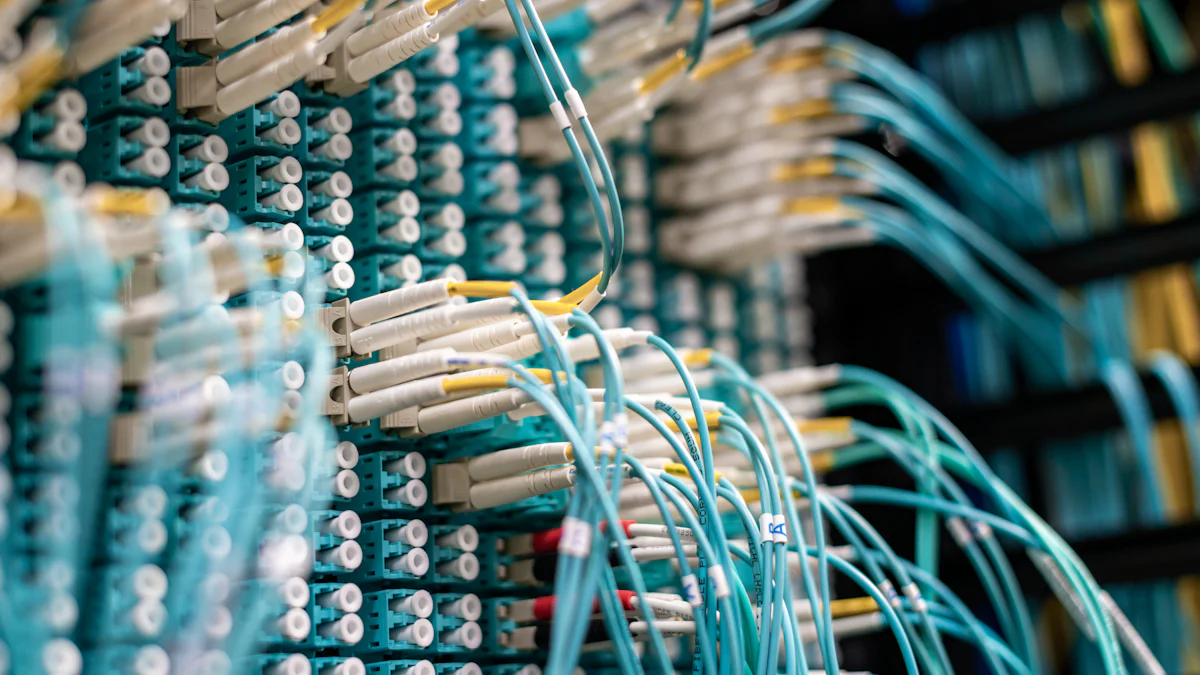
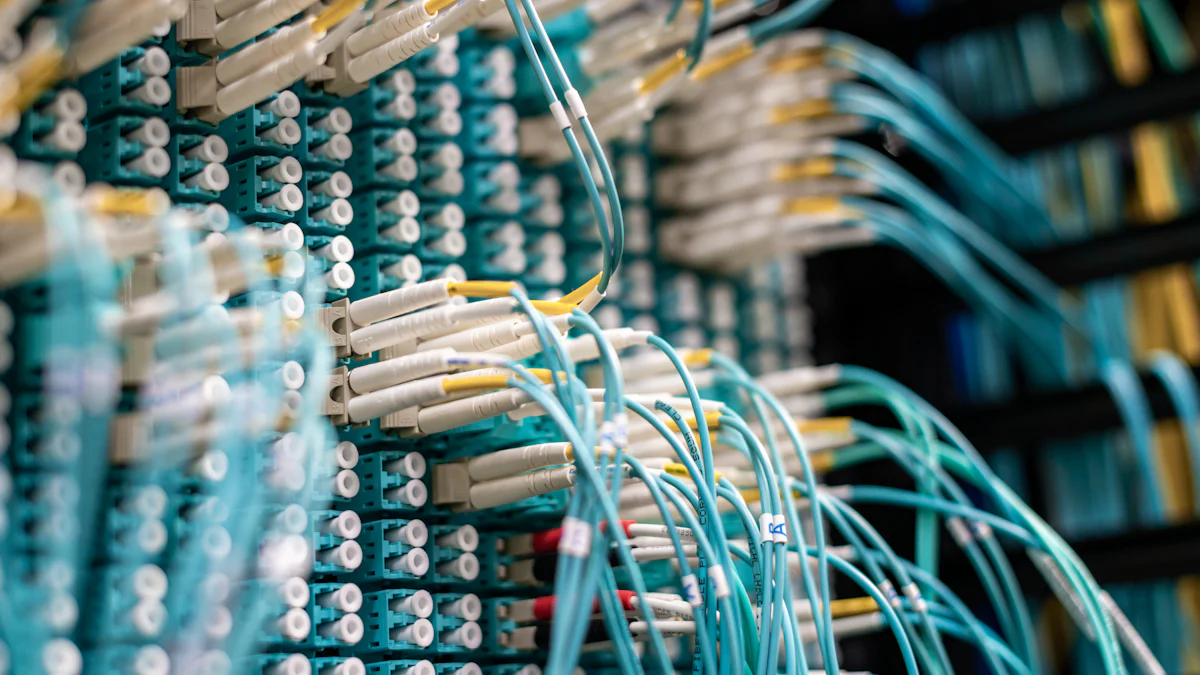
You can achieve optimal performance by effectively using a data server manager. These tools play a crucial role in enhancing your server’s performance, security, and cost management. By monitoring server performance metrics like CPU utilization and memory usage, you can identify bottlenecks and areas for improvement. This proactive approach minimizes risks and prevents failures, ensuring business productivity. Additionally, server management systems provide real-time operational data on a management dashboard, making tasks easier and reducing the probability of failures. Embrace server management to save time and money while maintaining a finely tuned, energy-efficient data center.
Understanding Data Server Managers
Definition and Importance
What are Data Server Managers?
Data server managers are essential tools that help you oversee and optimize the performance of your servers. They provide a centralized platform to monitor, manage, and maintain your server infrastructure. By using a data server manager, you can ensure that your servers operate efficiently and reliably. These tools track key metrics such as CPU usage, memory consumption, and network activity, allowing you to identify potential issues before they become critical.
Why are They Important for Businesses?
For businesses, data server managers are invaluable. They enhance operational efficiency by automating routine tasks and providing real-time insights into server performance. This proactive management reduces downtime and improves productivity. Moreover, data server managers bolster security by implementing best practices and conducting regular audits. They also contribute to cost savings by optimizing resource allocation and minimizing energy consumption. In today’s competitive landscape, leveraging a data server manager can give your business a significant edge.
Types of Data Servers
On-Premises Servers
On-premises servers are physical machines located within your organization’s premises. They offer robust performance and complete control over your data. However, they require significant investment in terms of space, power, and cooling resources. You must also manage their maintenance and security. Despite these challenges, on-premises servers remain a popular choice for businesses that prioritize data privacy and control.
Cloud Servers
Cloud servers provide a flexible and scalable alternative to on-premises servers. They allow you to access computing resources over the internet, eliminating the need for physical hardware. With cloud servers, you can easily scale your resources up or down based on demand. This flexibility makes them ideal for businesses with fluctuating workloads. Additionally, cloud servers offer enhanced disaster recovery capabilities, ensuring business continuity in case of data loss.
Hybrid Servers
Hybrid servers combine the best of both on-premises and cloud servers. They enable you to maintain critical data on-site while leveraging the scalability and flexibility of the cloud for other workloads. This approach provides a balanced solution that meets diverse business needs. By using hybrid servers, you can optimize performance, enhance security, and reduce costs. They offer a seamless integration of resources, allowing you to adapt to changing business requirements.
Key Server Management Activities
Setup and Configuration
Initial Setup Procedures
When you begin using a data server manager, the initial setup is crucial. Start by installing the software on your server. Follow the installation guide provided by the vendor. Ensure that your server meets all the necessary hardware and software requirements. After installation, configure the basic settings. This includes setting up user accounts, defining access permissions, and establishing network connections. Proper initial setup lays the foundation for effective server management.
Configuration Best Practices
Once the initial setup is complete, focus on configuration best practices. Customize your data server manager to suit your specific needs. Adjust settings to optimize performance. For example, allocate resources like CPU and memory based on your workload requirements. Regularly update your server manager software to benefit from the latest features and security patches. Implementing these best practices ensures that your data server manager operates efficiently and securely.
Monitoring and Performance Tuning
Tools for Monitoring
Monitoring is a key activity in server management. Use tools like Microsoft System Center, VMware vSphere, or Red Hat Ansible to keep an eye on your server’s performance. These tools offer various forms, including GUIs, CLIs, and web-based dashboards. They provide real-time insights into metrics such as CPU usage, memory consumption, and network activity. By using these tools, you can quickly identify and address potential issues, ensuring optimal server performance.
Techniques for Performance Tuning
Performance tuning involves adjusting your server settings to enhance efficiency. Start by analyzing the data collected from monitoring tools. Identify bottlenecks and areas for improvement. Techniques like load balancing and resource allocation can help optimize performance. Regularly review and adjust your server configurations to adapt to changing workloads. Performance tuning ensures that your data server manager delivers the best possible results.
Security Management
Implementing Security Protocols
Security is a top priority in server management. Implement robust security protocols to protect your data. Use firewalls, encryption, and access controls to safeguard your server. Regularly update your security settings to defend against emerging threats. A data server manager can help automate these tasks, ensuring that your server remains secure at all times.
Regular Security Audits
Conduct regular security audits to assess the effectiveness of your security measures. These audits help identify vulnerabilities and areas for improvement. Use the insights gained from audits to enhance your security protocols. Regular audits ensure that your data server manager maintains a high level of security, protecting your valuable data from potential threats.
Backup and Recovery
Backup Strategies
You must implement effective backup strategies to safeguard your data. Regular backups ensure that you can recover critical information in case of data loss. Consider using automated backup tools to streamline the process. These tools can schedule backups at regular intervals, reducing the risk of human error. Choose a backup method that suits your needs, such as full, incremental, or differential backups. Full backups copy all data, while incremental and differential backups save only changes since the last backup. This approach saves time and storage space.
“Regular data backups are essential to safeguard critical information and ensure business continuity in case of data loss.”
Additionally, consider the location of your backups. Storing backups off-site or in the cloud provides an extra layer of protection against physical disasters. Cloud-based solutions offer scalability and accessibility, allowing you to retrieve data from anywhere. Evaluate your backup strategy regularly to ensure it meets your evolving needs.
Recovery Planning
Recovery planning is crucial for minimizing downtime and ensuring business continuity. Develop a comprehensive recovery plan that outlines the steps to restore data after a loss. Identify critical data and prioritize its recovery. Test your recovery plan regularly to ensure its effectiveness. Simulate different scenarios to identify potential weaknesses and make necessary adjustments.
“Creating regular data backups not only protects against hardware failures but also mitigates risks associated with cyberattacks.”
Incorporate server management tools into your recovery plan. These tools offer features like automatic anomaly detection and built-in service checks, which enhance your recovery efforts. Choose tools that align with your needs and budget. Consider factors like ease of use, scalability, and compatibility with your existing systems. A well-executed recovery plan ensures that you can quickly resume operations after a data loss event, minimizing the impact on your business.
Benefits of Effective Server Management
Enhanced Performance
Effective server management significantly boosts your server’s performance. By regularly monitoring and tuning your server, you can identify and resolve bottlenecks swiftly. This proactive approach ensures that your server operates at peak efficiency, minimizing downtime and maximizing productivity. Utilizing server management tools allows you to automate routine tasks, freeing up resources for more critical operations. As a result, your server remains responsive and reliable, supporting your business needs seamlessly.
Improved Security
Security is paramount in server management. Implementing robust security protocols protects your data from unauthorized access and cyber threats. Regular security audits help you identify vulnerabilities and strengthen your defenses. By using server management tools, you can automate security updates and monitor for suspicious activities in real-time. This vigilance ensures that your server remains secure, safeguarding your valuable data and maintaining your organization’s reputation.
Cost Efficiency
Cost efficiency is a significant advantage of effective server management. By optimizing resource allocation, you can reduce energy consumption and lower operational costs. Server management tools provide insights into resource usage, allowing you to make informed decisions about scaling and resource distribution. Additionally, automating routine tasks reduces the need for manual intervention, saving time and labor costs. This strategic approach to server management ensures that you achieve maximum value from your IT investments.
Seamless Integration with Existing Systems
Integrating a data server manager with your existing systems can significantly enhance your IT infrastructure’s efficiency. This integration allows you to streamline operations and improve overall performance. Here’s how you can achieve seamless integration:
Assess Compatibility: Before integrating a data server manager, evaluate its compatibility with your current systems. Ensure that it supports the operating systems, applications, and hardware you already use. This step prevents potential conflicts and ensures smooth operation.
Utilize APIs and Connectors: Many data server managers offer APIs and connectors that facilitate integration with other software. These tools allow different systems to communicate effectively, enabling data sharing and synchronization. By leveraging these features, you can create a cohesive IT environment.
Implement Gradual Integration: Start with a phased approach to integration. Begin by connecting the data server manager to non-critical systems. Monitor the performance and address any issues that arise. Once you confirm stability, proceed to integrate with more critical systems. This method minimizes disruptions and ensures a smooth transition.
Conduct Regular Testing: After integration, regularly test the systems to ensure they function correctly. Testing helps identify any integration-related issues early, allowing you to address them promptly. It also ensures that the data server manager continues to operate efficiently within your IT ecosystem.
Train Your Team: Provide training for your IT staff on using the data server manager. Familiarize them with its features and integration capabilities. A well-trained team can effectively manage the integrated systems, maximizing the benefits of the data server manager.
“Utilizing server management tools with disaster recovery capabilities enhances business continuity and resilience against disruptions.”
By following these steps, you can ensure that your data server manager integrates seamlessly with your existing systems. This integration not only enhances performance but also supports business continuity by providing a robust and resilient IT infrastructure.
Role of Server Management Tools
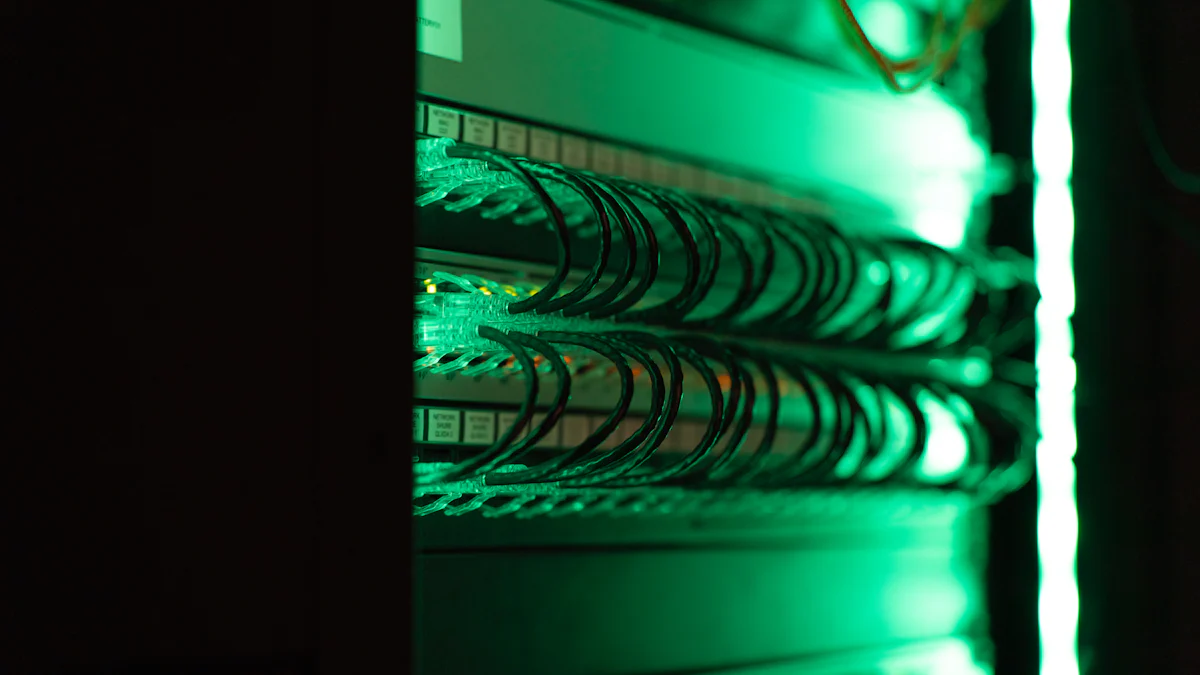
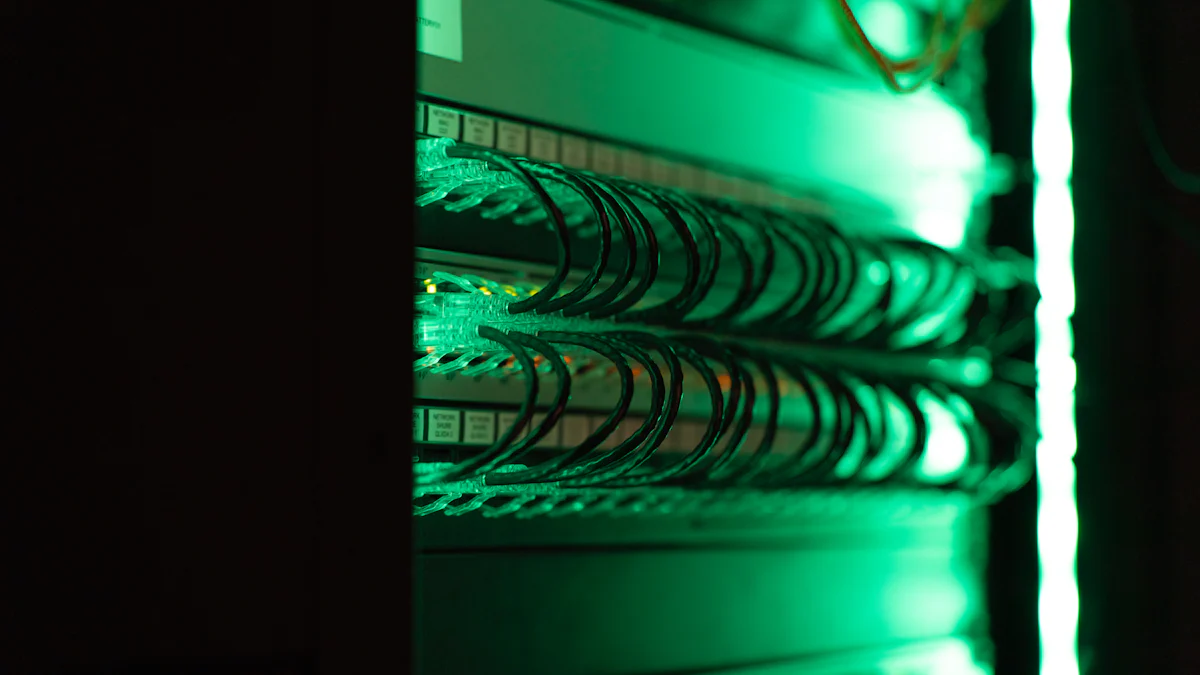
Server management tools play a vital role in maintaining the efficiency and reliability of your IT infrastructure. These tools help you monitor, manage, and optimize your servers, ensuring they perform at their best. By using server management tools, you can automate routine tasks, enhance security, and improve overall system performance.
Overview of Popular Tools
Several popular server management tools are available to help you manage your data server manager effectively. Each tool offers unique features and capabilities, catering to different needs and preferences.
Microsoft System Center: This tool provides comprehensive management solutions for your IT infrastructure. It offers features like monitoring, automation, and configuration management, making it a popular choice for businesses of all sizes.
VMware vSphere: Known for its virtualization capabilities, VMware vSphere allows you to manage virtualized environments efficiently. It offers features like resource allocation, performance monitoring, and security management.
Red Hat Ansible: This open-source tool focuses on automation and configuration management. It simplifies complex tasks and ensures consistency across your server environment.
“Given the importance of reliable servers, many organizations are turning to server management services for support.” – SupportPro, Server Management Services Expert
Features to Look for in a Tool
When choosing a server management tool, consider the features that will best support your data server manager. Look for tools that offer:
Automation: Automating routine tasks saves time and reduces the risk of human error. Choose a tool that can automate processes like backups, updates, and performance monitoring.
Scalability: As your business grows, your server management needs may change. Select a tool that can scale with your organization, accommodating increased workloads and additional servers.
Security: Protecting your data is crucial. Opt for a tool that offers robust security features, such as encryption, access controls, and regular security updates.
User-Friendly Interface: A tool with an intuitive interface makes it easier for your team to manage and monitor your servers. Look for tools that offer dashboards and visualizations to simplify complex data.
How to Choose the Right Tool for Your Needs
Selecting the right server management tool involves evaluating your specific requirements and comparing available options. Follow these steps to make an informed decision:
Assess Your Needs: Identify the key features and capabilities you require in a server management tool. Consider factors like automation, scalability, and security.
Research Available Options: Explore the various tools on the market, focusing on those that align with your needs. Read reviews and seek recommendations from industry experts.
Test the Tools: Many server management tools offer free trials or demos. Take advantage of these opportunities to test the tools and assess their suitability for your data server manager.
Evaluate Cost and Support: Consider the cost of the tool and the level of support provided by the vendor. Ensure that the tool fits within your budget and offers adequate support for your team.
Make an Informed Decision: Based on your research and testing, choose the tool that best meets your needs and supports your data server manager effectively.
By carefully selecting the right server management tool, you can enhance your server’s performance, security, and efficiency, ultimately supporting your business’s success.
Strategies for Efficient Data Management
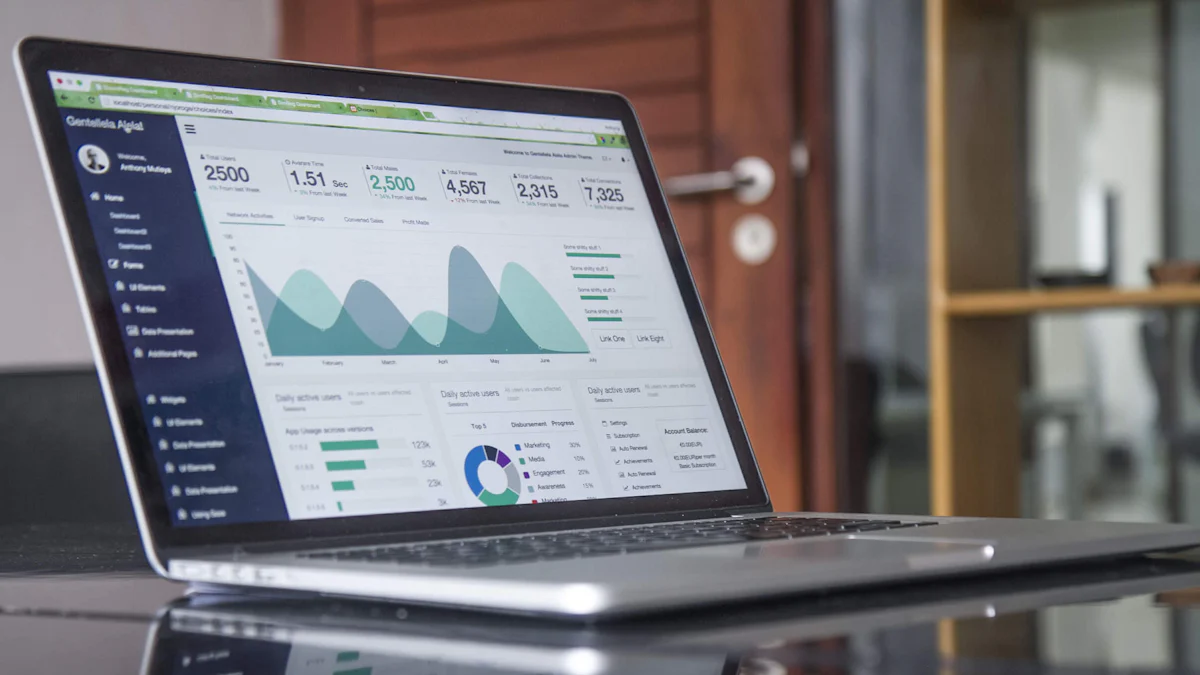
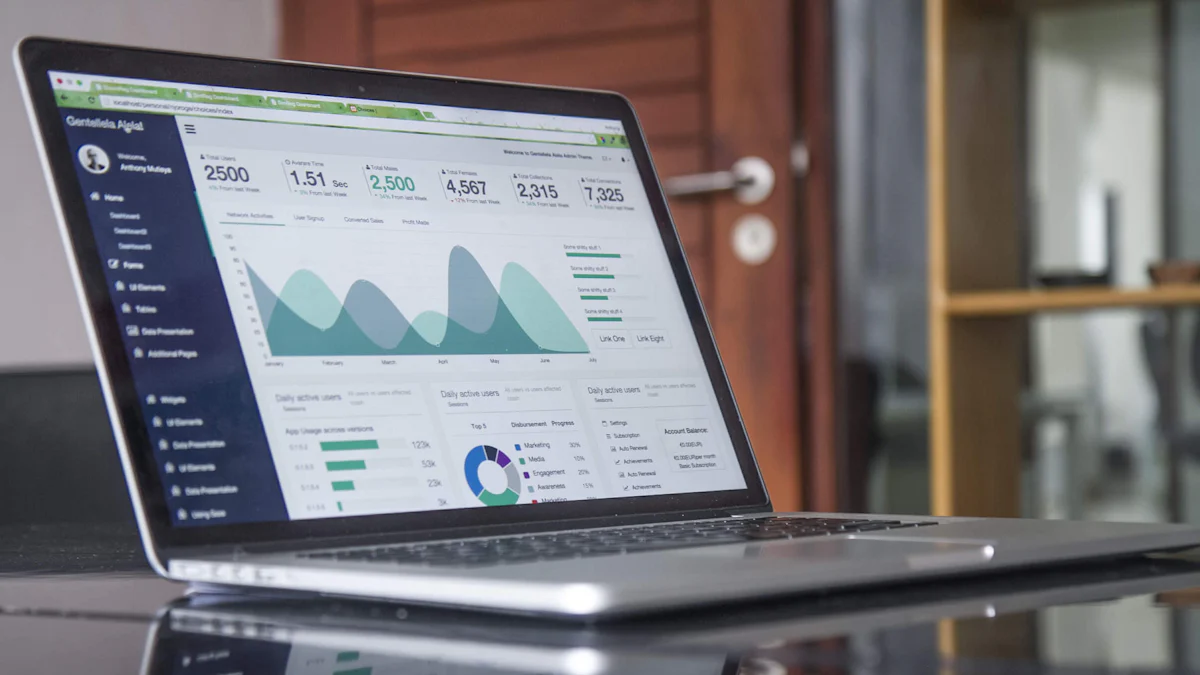
Data Organization Techniques
Efficient data management begins with organizing your data effectively. You should categorize your data based on its type and relevance. This approach simplifies data retrieval and enhances operational efficiency. Consider using a structured format, such as databases or spreadsheets, to store your data. These tools allow you to sort, filter, and analyze information quickly.
Labeling is another crucial technique. Assign clear and descriptive labels to your data files. This practice helps you identify the contents at a glance, reducing the time spent searching for specific information. Consistency in labeling ensures that everyone in your organization understands the data structure.
Regularly review and update your data organization methods. As your business evolves, your data needs may change. Adapt your organization techniques to accommodate new data types and volumes. This proactive approach keeps your data management system efficient and relevant.
Automating Routine Tasks
Automation plays a vital role in efficient data management. By automating routine tasks, you can save time and reduce the risk of human error. Identify repetitive tasks in your data management process, such as data entry or report generation. Use automation tools to handle these tasks, freeing up your resources for more critical activities.
Consider implementing scripts or software solutions that automate data processing. These tools can perform tasks like data validation, transformation, and integration. Automation ensures consistency and accuracy in your data management efforts.
Regularly evaluate your automation processes. Technology evolves rapidly, and new tools may offer better solutions for your needs. Stay informed about the latest advancements in automation technology. By doing so, you can continuously improve your data management efficiency.
Regular Performance Reviews
Conducting regular performance reviews is essential for maintaining efficient data management. Schedule periodic assessments of your data management processes. These reviews help you identify areas for improvement and ensure that your strategies remain effective.
During a performance review, analyze key metrics such as data retrieval times, error rates, and resource utilization. Use these insights to make informed decisions about optimizing your data management system. Address any identified issues promptly to prevent them from affecting your operations.
Involve your team in the review process. Encourage open communication and collaboration among stakeholders. Their feedback can provide valuable perspectives on the effectiveness of your data management strategies. By fostering a culture of continuous improvement, you can enhance your organization’s overall data management capabilities.
You have explored the essential aspects of using data server managers for optimal performance. By implementing strategies like effective setup, monitoring, and security management, you can enhance your server’s efficiency and reliability. Tools such as Microsoft System Center and VMware vSphere offer automation capabilities that reduce human error and improve compliance. Take action by applying these insights to your server management practices. This proactive approach will ensure your IT infrastructure remains robust and efficient, supporting your business’s success.
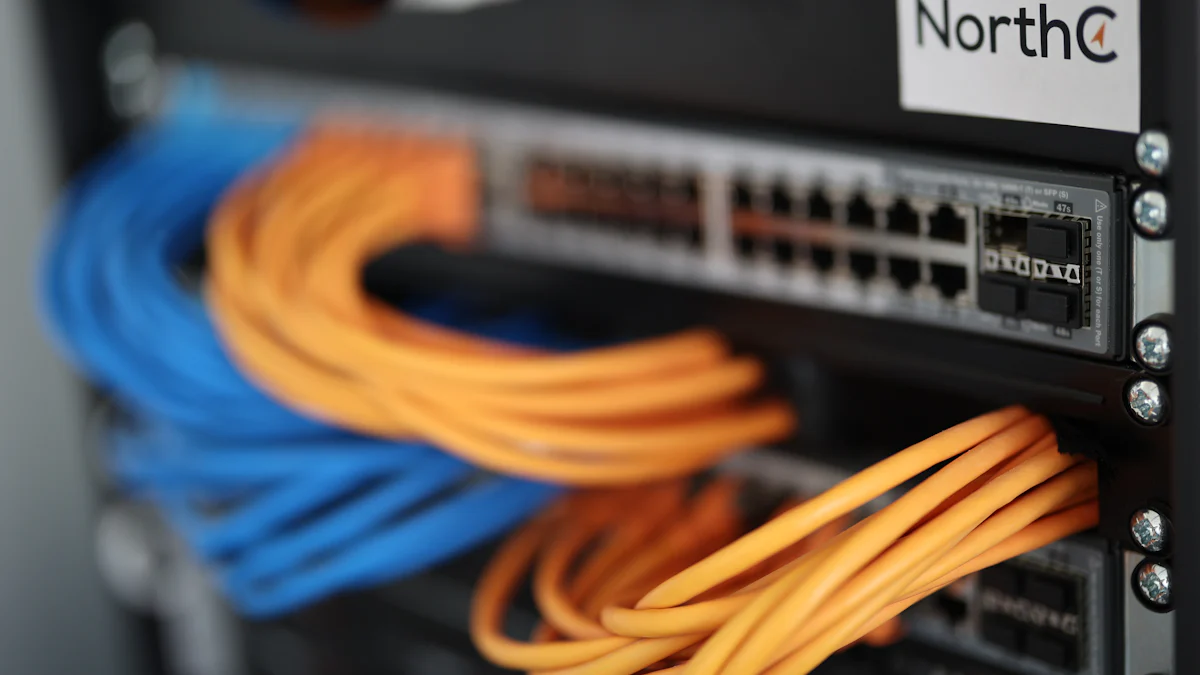
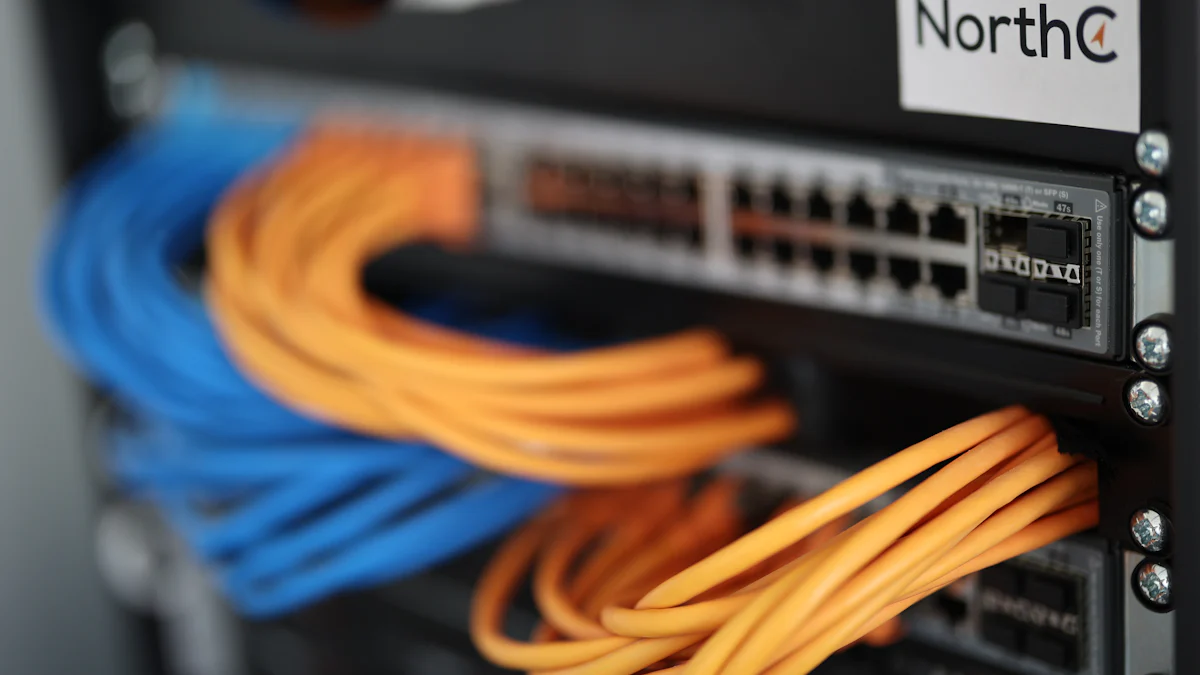
Sysracks offers a compelling value proposition for those seeking a reliable Server Rack solution. The product stands out due to its exceptional quality, cost-effectiveness, and robust features. Users appreciate its durability, which ensures long-term use without frequent replacements. Additionally, Sysracks provides excellent customer support, enhancing the overall user experience. These attributes make Sysracks a worthy investment for anyone needing efficient data management and organization.
Key Features of Sysracks
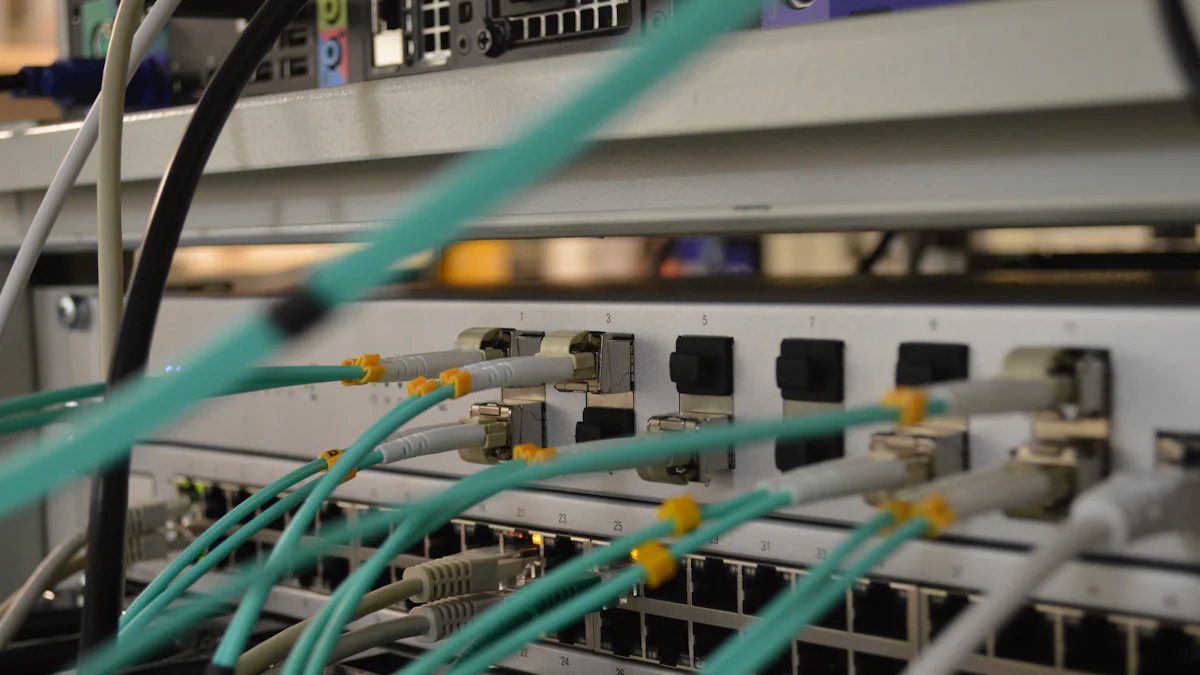
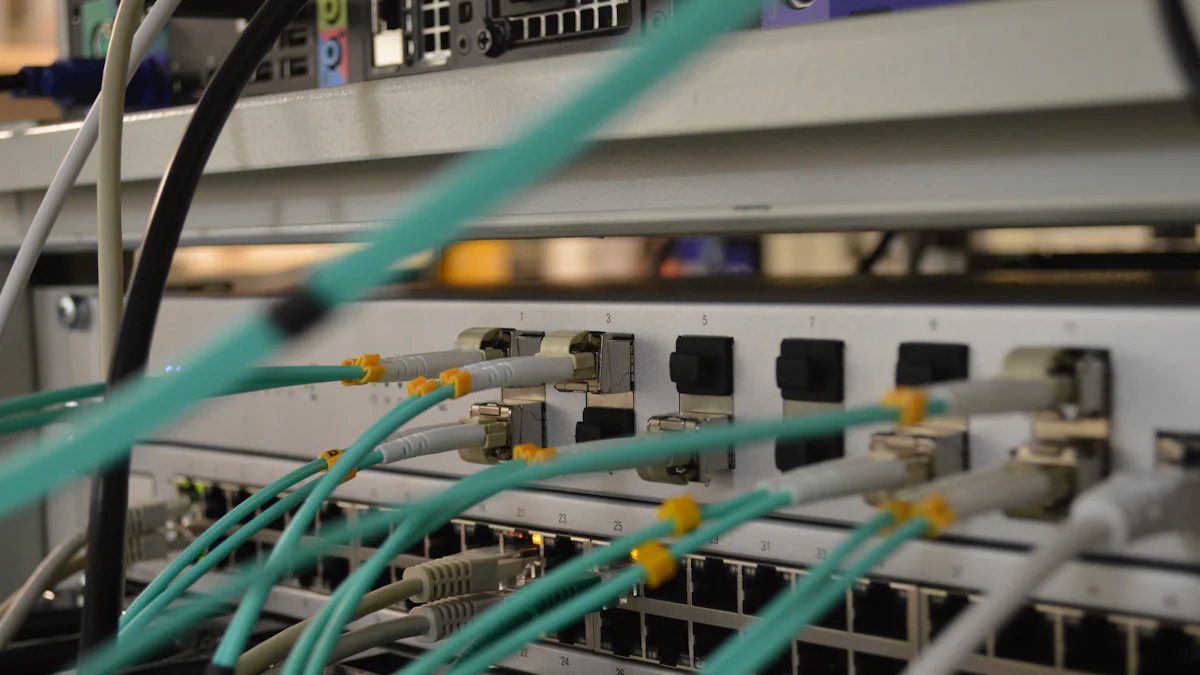
Build Quality
Materials Used
Sysracks constructs its Server Rack products using high-quality materials, ensuring durability and reliability. The Sysracks 42U Free Standing Server Rack Cabinet exemplifies this with its robust alloy steel frame. This material choice provides a sturdy structure capable of supporting up to 1600 lbs of equipment. The use of powder-coated finishes not only enhances the aesthetic appeal but also offers protection against corrosion, extending the lifespan of the rack.
Design and Aesthetics
The design of Sysracks Server Racks combines functionality with visual appeal. For instance, the Sysracks 6U 24 inch Depth Wall Mounted Server Rack Cabinet features a modern design with a light gray finish that fits seamlessly into various environments. The lockable glass front door in a perforated metal frame adds a touch of elegance while ensuring security. Additionally, the compact and sleek design of the Sysracks 18U 35 Depth Portable Under Desk 19 Enclosure makes it an ideal choice for both home and office settings, providing a professional look without occupying excessive space.
Compatibility
Support for Various Server Types
Sysracks Server Racks offer versatile compatibility, accommodating a wide range of server types and IT equipment. The Sysracks 42U Free Standing Server Rack Cabinet supports 19-inch standard IT and telecom equipment, making it suitable for data centers and advanced network configurations. Similarly, the Sysracks 12U Data Racks cater to small businesses, educational institutions, and large corporations, highlighting their adaptability across different sectors.
Customization Options
Customization plays a crucial role in meeting specific user needs, and Sysracks excels in this area. Users can tailor their Server Rack setups with various accessories and configurations. For example, the Sysracks Standing Network Rack includes features like built-in cooling fans and adjustable shelves, allowing users to optimize their setup for performance and efficiency. The inclusion of brush strip panels and vented shelves in many models further enhances cable management and airflow, ensuring organized and efficient operation.
Cost-Effectiveness
Pricing Analysis
Sysracks stands out in the market due to its competitive pricing strategy. They offer high-quality server racks at manufacturer prices, making them an attractive option for budget-conscious buyers. This approach allows Sysracks to provide excellent value without compromising on quality.
Comparison with Competitors
When comparing Sysracks to its competitors, several key differences emerge:
Price Point: Sysracks offers products at a lower price point compared to many competitors. This affordability does not come at the expense of quality, as Sysracks maintains high standards in its product offerings.
Quality Assurance: While some competitors may offer similar products, Sysracks ensures that their server racks meet rigorous quality checks, providing peace of mind to users.
Range of Options: Sysracks caters to a wide range of needs, offering both premium and budget-friendly options. This flexibility allows customers to choose products that best fit their financial and operational requirements.
Unique Value or Cost-Saving Aspects
Sysracks provides several unique value propositions that contribute to cost savings:
Direct Manufacturer Pricing: By selling directly from the manufacturer, Sysracks eliminates middleman costs, passing the savings onto the customer.
Durability and Longevity: The robust construction of Sysracks products means fewer replacements over time, reducing long-term expenses.
Included Accessories: Many Sysracks models come with essential accessories, such as cooling fans and power distribution units, which would otherwise incur additional costs if purchased separately.
These factors collectively make Sysracks a cost-effective choice for those seeking reliable and affordable server rack solutions.
User Experience
Customer Reviews
Customer reviews provide valuable insights into the Sysracks Server Rack’s performance and user satisfaction. Many users have shared their experiences, highlighting key aspects of the product.
Ease of Installation
Users frequently praise the Sysracks Server Rack for its straightforward installation process. The product comes with a comprehensive video guide that simplifies assembly. This feature proves beneficial for individuals with varying levels of technical expertise. Customers appreciate the welded frame design, which eliminates the need for special tools. The inclusion of heavy-duty casters further enhances the setup experience by allowing easy mobility and positioning. Overall, users find the installation process quick and hassle-free.
Usability and Performance
Sysracks Server Racks receive high marks for usability and performance. Customers commend the product’s robust construction, which supports a wide range of equipment securely. The locking cabinet design adds an extra layer of security, protecting valuable devices from unauthorized access. Users also highlight the efficient cable management system, which keeps setups organized and clutter-free. The built-in cooling fan maintains optimal airflow, ensuring equipment operates at peak performance. Many reviews mention the stylish black finish, which complements various environments, adding to the rack’s appeal. Overall, users express satisfaction with the Sysracks Server Rack’s functionality and reliability.
Durability and Reliability
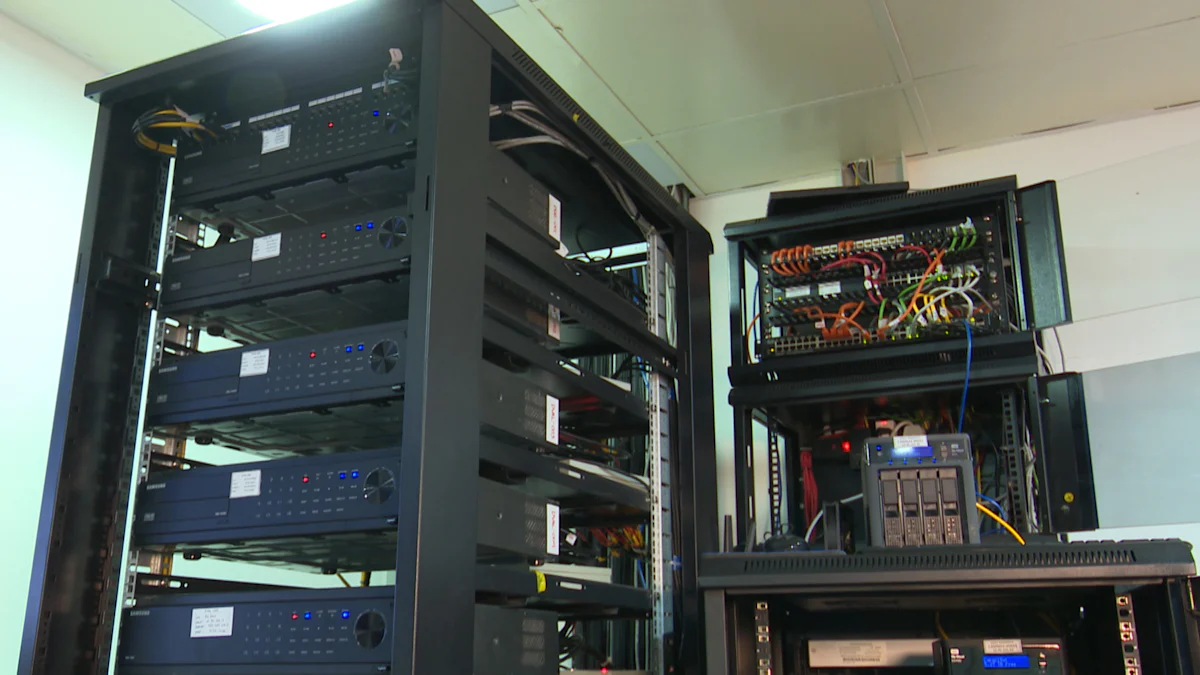
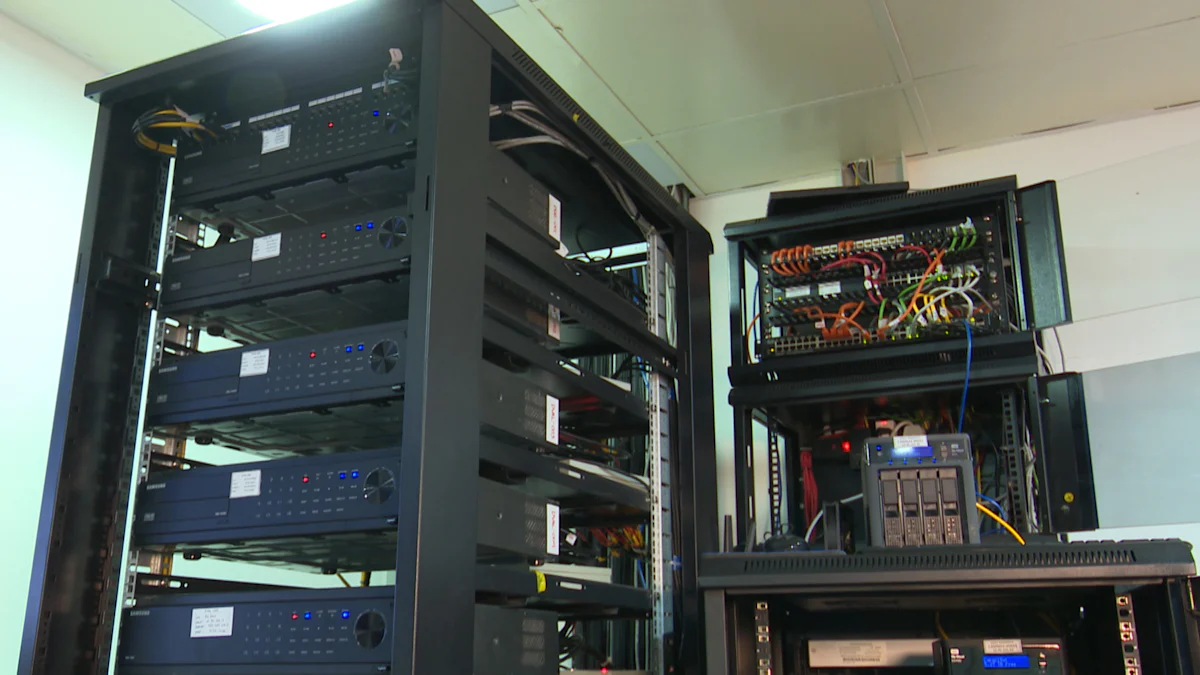
Longevity
Sysracks Server Rack products excel in durability and reliability, making them a preferred choice for long-term use. The longevity of these racks stems from their superior materials and construction techniques.
Materials and Construction
Sysracks employs high-quality alloy steel in the construction of their Server Rack products. This choice of material ensures a robust and stable structure, capable of withstanding significant weight and pressure. The alloy steel frame provides excellent resistance to wear and tear, which is crucial for maintaining the integrity of the rack over time. Additionally, the powder-coated finish protects against corrosion, further extending the lifespan of the Server Rack. This meticulous attention to materials and construction results in a product that users can rely on for years without the need for frequent replacements.
Warranty Offerings
Sysracks stands behind the durability of their Server Rack products by offering comprehensive warranty options. Customers receive a two-year satisfaction guarantee, which underscores the company’s commitment to quality and customer satisfaction. This warranty provides peace of mind, assuring users that they are investing in a reliable product. In the rare event of any issues, Sysracks’ responsive customer support team is readily available to address concerns and provide solutions. The combination of robust materials, thoughtful construction, and strong warranty offerings makes Sysracks Server Rack products a dependable choice for anyone seeking long-lasting equipment solutions.
Customer Support
Sysracks excels in providing outstanding customer support, which significantly enhances the overall user experience. Their commitment to customer satisfaction is evident in their proactive approach to addressing inquiries and resolving issues.
Quality of Service
Sysracks prioritizes quality in their customer service. They ensure that every interaction with their support team leaves a positive impression on the customer. The staff at Sysracks demonstrate a deep understanding of their products, which allows them to offer precise and helpful guidance. Customers often feel valued, regardless of the size of their purchase. This dedication to service quality fosters trust and loyalty among users.
Availability
Sysracks makes their customer support readily accessible to all users. They provide multiple channels for communication, including phone, email, and an online support page. This ensures that customers can reach out with questions about their Server Rack products, services, shipping, or cancellations at any time. The availability of comprehensive product help materials, such as manuals and certifications, further supports users in making informed decisions and troubleshooting independently.
Responsiveness
The responsiveness of Sysracks’ customer support team sets them apart from competitors. Customers frequently praise the promptness with which their inquiries are addressed. For instance, one user reported receiving immediate assistance when they encountered shipping damage to their Server Rack. Sysracks quickly dispatched replacement parts, demonstrating their commitment to resolving issues efficiently. This level of responsiveness not only resolves problems swiftly but also reinforces the company’s reputation for reliability and customer care.
Sysracks’ dedication to providing exceptional customer support ensures that users have a seamless experience with their Server Rack products. Their focus on availability and responsiveness makes them a trusted partner for anyone seeking reliable server solutions.
Sysracks emerges as a top choice for those seeking reliable server rack solutions. The analysis highlights its exceptional build quality, cost-effectiveness, and user-friendly features. Customers consistently praise Sysracks for its high-quality products and excellent customer service. For instance, one customer noted the prompt response and resolution when they encountered an issue with their 12U cabinet. Another customer appreciated the ease of assembly and completeness of the 6U data rack kit. These experiences underscore the overall satisfaction and value for money that Sysracks provides, making it a worthy investment.
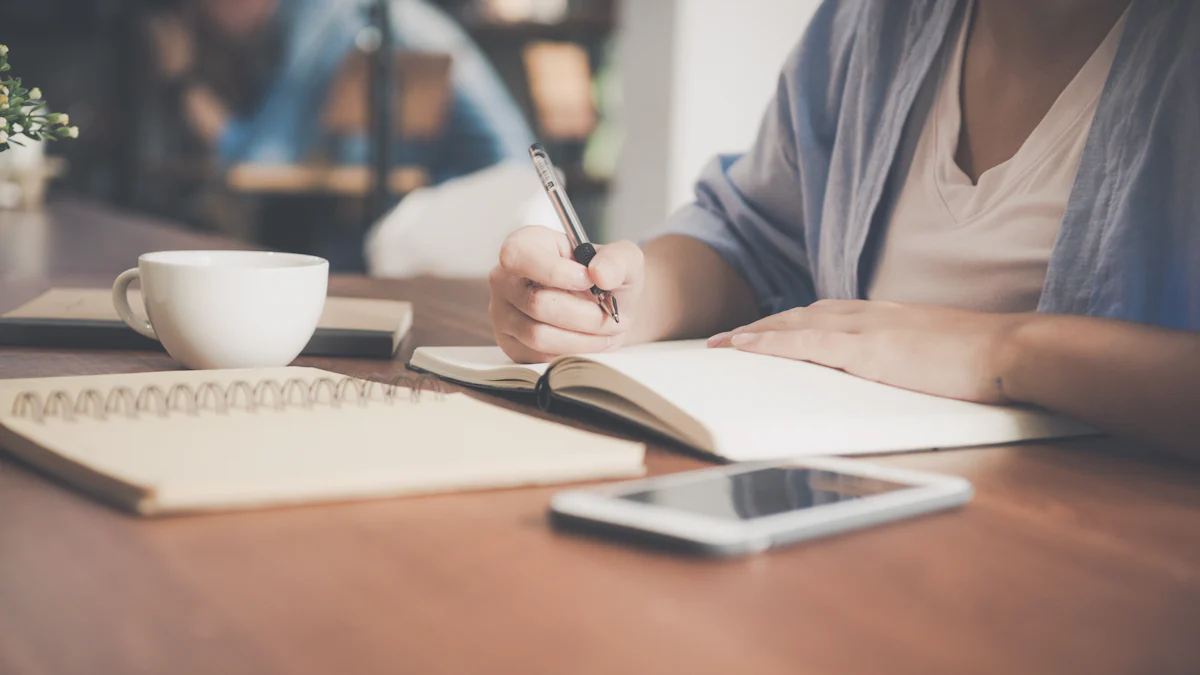
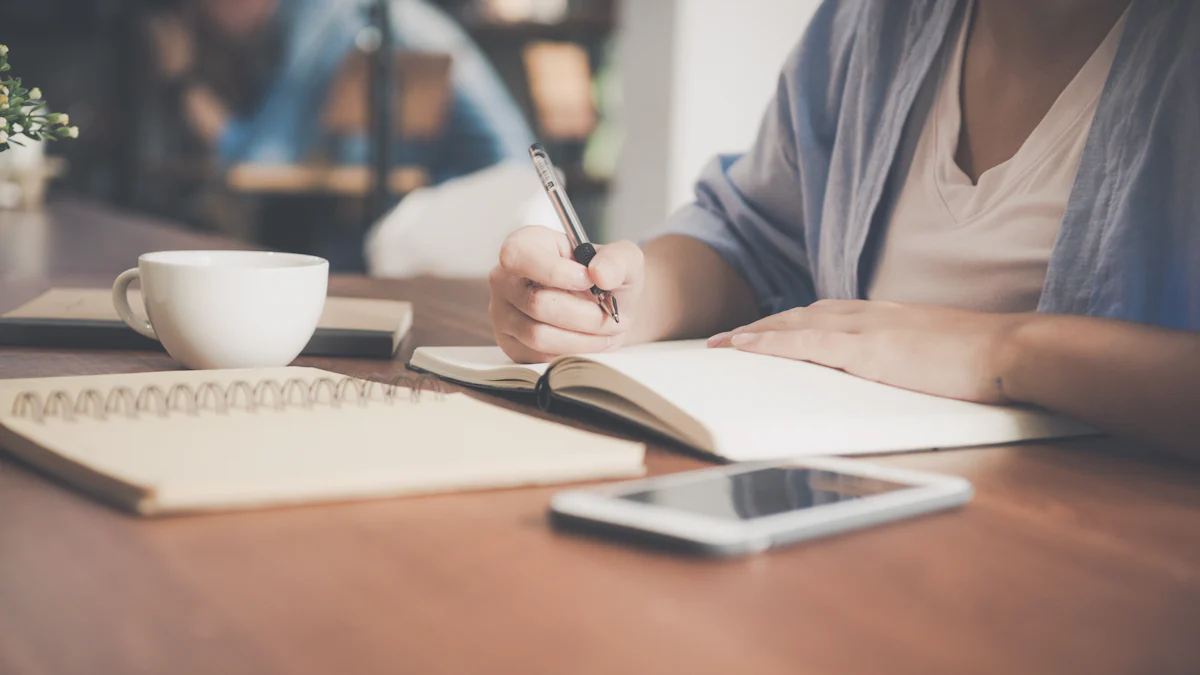
DataCamp courses offer a practical approach to learning data science. You might wonder about their effectiveness, especially if you’re considering enrolling. Many users express satisfaction with DataCamp, highlighting its engaging learning environment. The platform boasts a 60% course completion rate, significantly higher than the industry norm of 15%. This impressive statistic reflects the platform’s ability to keep learners engaged. Positive data camp reviews further emphasize user satisfaction, making it a compelling choice for those eager to enhance their skills in data science.
Pros and Cons of DataCamp
When you consider enrolling in a DataCamp course, understanding its pros and cons can help you make an informed decision. Let’s dive into the details.
Course Quality
Curriculum Design
DataCamp’s curriculum design stands out for its practical approach. The courses are structured to be bite-sized and digestible, making it easier for you to absorb complex topics. This design encourages consistent learning, which is crucial for mastering data science skills. The platform offers a variety of courses tailored to different skill levels, ensuring that both beginners and advanced learners find suitable content.
Instructor Expertise
DataCamp collaborates with subject matter experts in data science and analytics. These instructors bring a wealth of knowledge and experience to the table. According to Upskillwise, DataCamp instructors take ownership of their courses, ensuring high-quality content. This expertise translates into engaging lessons that keep you motivated and eager to learn more.
User Experience
Platform Usability
The usability of DataCamp’s platform is one of its strong points. You will find the interface intuitive and easy to navigate. This user-friendly design allows you to focus on learning without getting bogged down by technical difficulties. The platform’s seamless experience contributes to its high course completion rate, as noted in many data camp reviews.
Interactive Learning
DataCamp excels in providing an interactive learning environment. The platform combines videos with hands-on exercises, allowing you to apply what you’ve learned immediately. This approach not only reinforces your understanding but also makes learning enjoyable. BitDegree highlights the inclusion of real-world projects and a dedicated workspace for practice, which enhances the overall learning experience.
Accreditation
Certification Value
While DataCamp does not offer accredited certificates, the value of its certifications lies in the skills you acquire. Employers often recognize the practical skills gained through DataCamp courses, which can boost your job prospects. The platform’s focus on real-world applications ensures that you are well-prepared for industry challenges.
Industry Recognition
DataCamp has gained recognition in the industry for its specialized focus on data science. Many data camp reviews praise the platform for its comprehensive range of courses in machine learning and artificial intelligence. This recognition adds credibility to the skills you develop, making them valuable in the job market.
Comparison with Competitors
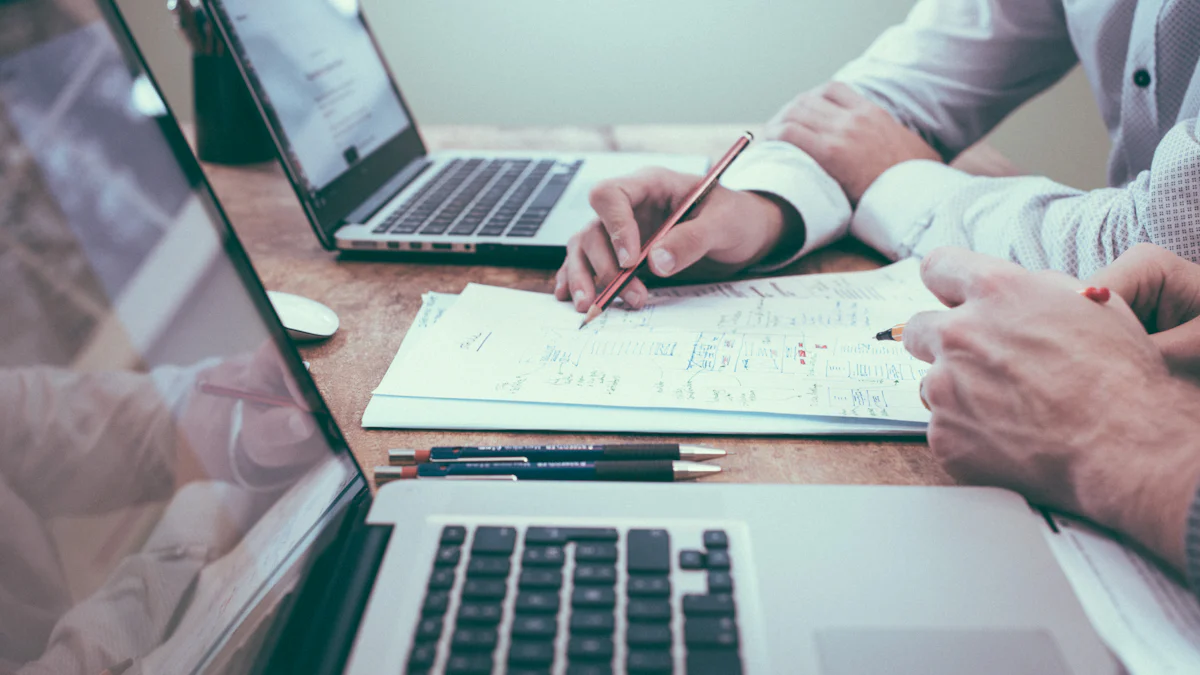
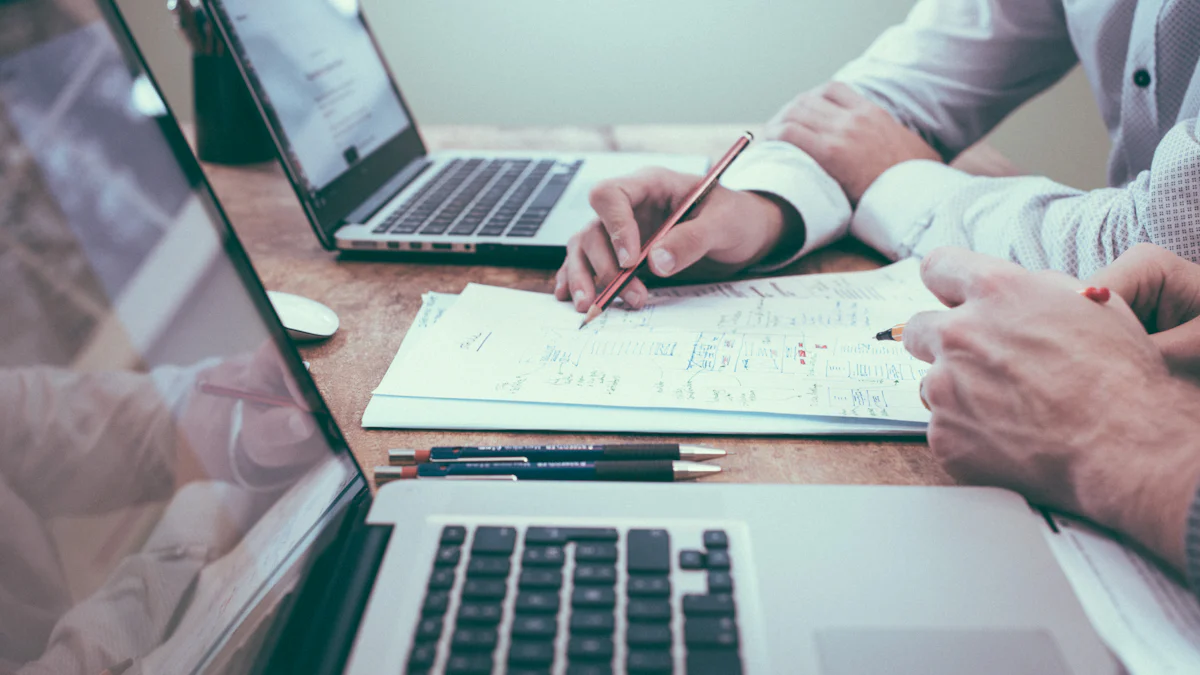
When you’re exploring online learning platforms, it’s essential to understand what makes each one unique. Let’s take a closer look at how DataCamp stacks up against its competitors.
Unique Features of DataCamp
Interactive Coding Environment
DataCamp offers an interactive coding environment that sets it apart from many other platforms. You get to write and run code directly in your browser, which means you can practice coding without needing to install any software. This feature makes learning more accessible and convenient. You can experiment with code and see the results instantly, which helps reinforce your understanding of the material.
Project-Based Learning
Another standout feature of DataCamp is its project-based learning approach. You don’t just learn theory; you apply what you’ve learned to real-world projects. This hands-on experience is invaluable because it prepares you for actual data science tasks. By working on projects, you gain practical skills that you can showcase to potential employers.
Competitor Analysis
Coursera vs. DataCamp
When comparing Coursera and DataCamp, you’ll notice some key differences. Coursera offers a wide range of courses across various fields, not just data science. It partners with top universities and organizations to provide high-quality content. If you’re looking for a broader educational experience, Coursera might be the way to go. However, if your focus is solely on data skills, DataCamp’s specialized courses might suit you better. DataCamp’s certificates focus primarily on data skills, while Coursera’s certificates span a broader range of fields.
Udacity vs. DataCamp
Udacity and DataCamp both offer specialized courses, but they differ in their approach. Udacity provides “Nanodegree” programs that are more comprehensive and often include mentorship and career services. These programs can be more intensive and time-consuming. On the other hand, DataCamp’s courses are modular and designed for quick upskilling. If you prefer a more flexible learning schedule, DataCamp might be the better choice. DataCamp focuses specifically on data science, while Udacity offers a curated selection of courses from top universities and organizations.
Detailed Analysis of Course Content, Pricing, and User Reviews
Course Content
Range of Topics
DataCamp offers a wide array of topics that cater to different interests and skill levels. Whether you’re a beginner or an advanced learner, you’ll find courses that suit your needs. The platform covers essential areas like Python, R, SQL, and data science. This variety ensures you can explore different facets of data science and find your niche. The courses are designed to be concise, allowing you to grasp complex concepts without feeling overwhelmed.
Depth of Material
The depth of material in DataCamp courses is commendable. Each course delves into the subject matter, providing you with a thorough understanding. The lessons are structured to build on each other, reinforcing your knowledge as you progress. This approach helps you develop a solid foundation in data science. You won’t just skim the surface; you’ll gain a deep understanding of the topics you study.
Pricing Structure
Subscription Plans
DataCamp offers several subscription plans to accommodate different budgets and learning goals. You can choose from monthly or annual plans, depending on how long you plan to use the platform. The flexibility in pricing allows you to select a plan that fits your financial situation. This way, you can focus on learning without worrying about breaking the bank.
Cost-Effectiveness
When it comes to cost-effectiveness, DataCamp provides good value for the money. The platform’s pricing is competitive compared to other online learning platforms. You get access to a wealth of resources, including interactive exercises and real-world projects. This hands-on experience enhances your learning and makes the investment worthwhile. Many users find that the skills they acquire justify the cost of the subscription.
User Reviews
Positive Feedback
DataCamp receives a lot of positive feedback from its users. In a survey of 363 DataCamp customers, nearly 80% gave the platform a 5-star rating. Users appreciate the varied and concise content, which covers a broad range of topics. The overall experience is rated 4.35 out of 5 by alumni, students, and applicants. This high level of satisfaction speaks to the quality of the courses and the effectiveness of the learning experience.
Common Criticisms
While DataCamp is well-received, it does face some criticisms. About 13% of users in the same survey gave a 1-star rating. Some common complaints include the lack of accredited certificates and occasional technical issues with the platform. However, these criticisms are relatively minor compared to the overall positive feedback. Most users find that the benefits of DataCamp outweigh these drawbacks, making it a popular choice for learning data science.
DataCamp offers a robust platform for learning data science skills. You can benefit from its interactive, hands-on approach, which is highly effective for those who learn best by doing. The platform provides a versatile learning experience with individual courses, Skill Tracks, and Career Tracks, making it suitable for everyone. While there are some limitations, like the lack of accredited certificates, the practical skills you gain hold significant value. Overall, DataCamp provides good value for learners seeking practical skills in data science and AI, making it a top choice for those focused on these fields.
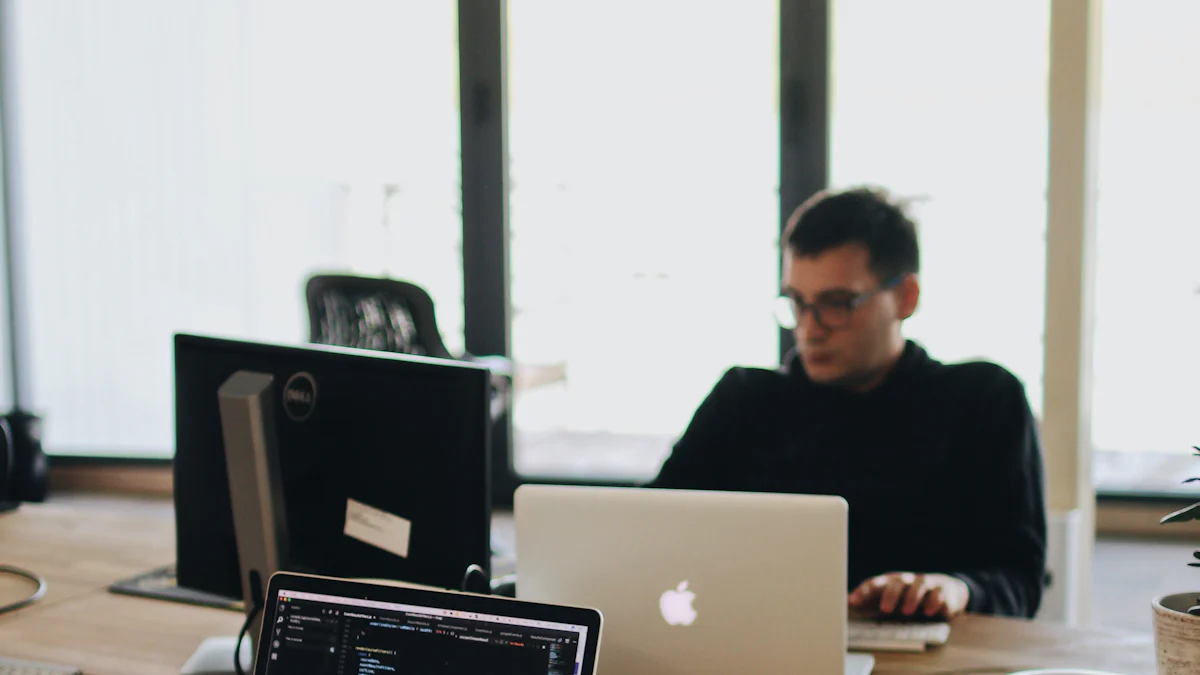
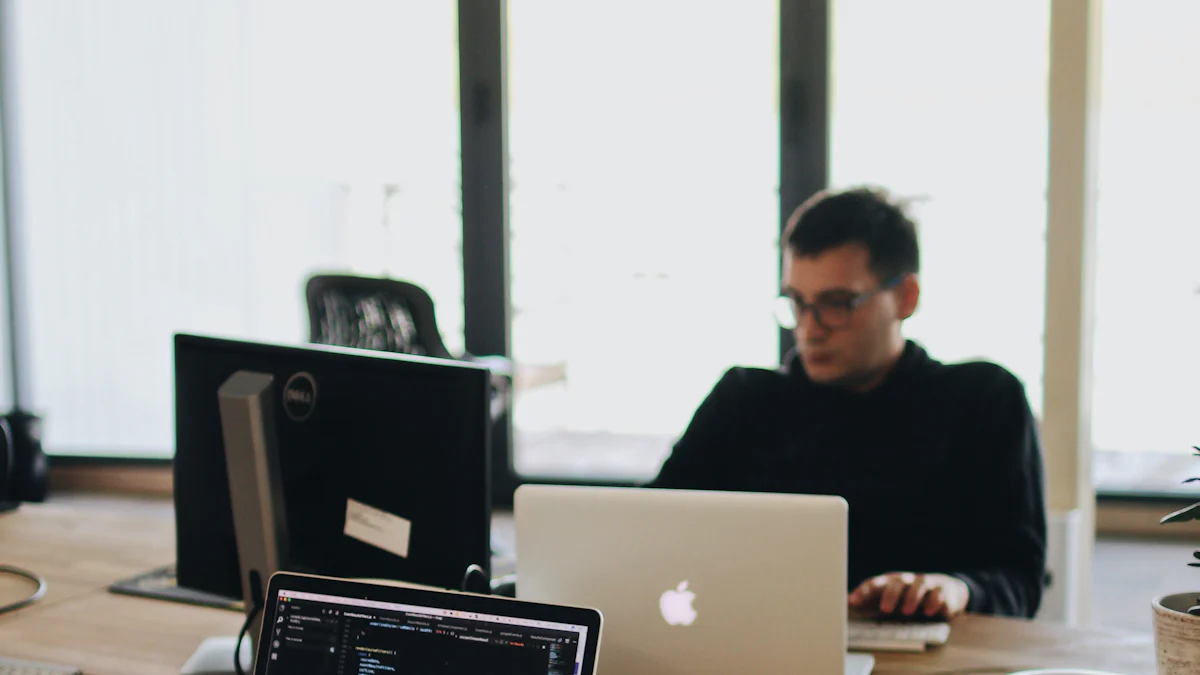
You might have noticed the buzz around data center jobs lately. This field is booming, with data creation expected to grow at a 23% compound annual growth rate through 2030. The demand for skilled professionals in data centers is on the rise, with job growth projected at 2% annually through 2023 and 3% by 2025. These roles are not just about numbers; they offer a variety of opportunities that are crucial to the tech industry. Whether you’re starting out or looking to advance, data center jobs provide a competitive edge and a clear path for career progression.
Overview of Data Centers
Definition and Functionality
Data centers are specialized facilities designed to house computer systems and associated components, such as telecommunications and storage systems. They provide a controlled environment to ensure the optimal performance of these systems. You can think of them as the backbone of modern digital infrastructure. They manage data storage, processing, and dissemination, making them essential for businesses that rely on digital operations.
The concept of data centers dates back to the 1940s. Back then, massive computer rooms were necessary to accommodate early computer systems like the ENIAC. These systems required a dedicated space due to their size and complexity. Over time, data centers have evolved significantly. From the colossal machines of the past, they have transformed into highly efficient facilities that support a wide range of technological needs.
Significance in Modern Technology
In today’s world, data centers play a crucial role in supporting the digital economy. They are the nerve centers for countless businesses, enabling everything from online shopping to cloud computing. During the 1990s, the dot-com boom led to a surge in demand for data centers. Companies needed fast internet connections and reliable systems to establish an online presence. This period marked the rise of the first true data centers, where multiple microcomputers and PC servers replaced traditional mainframes.
Data centers are now indispensable in modern technology. They provide the infrastructure necessary for the seamless operation of websites, applications, and services that you use daily. As technology continues to advance, the importance of data centers will only grow. They are at the heart of innovations like artificial intelligence, big data analytics, and the Internet of Things (IoT). By supporting these technologies, data centers drive progress and enable new possibilities in the digital age.
Key Data Center Job Roles
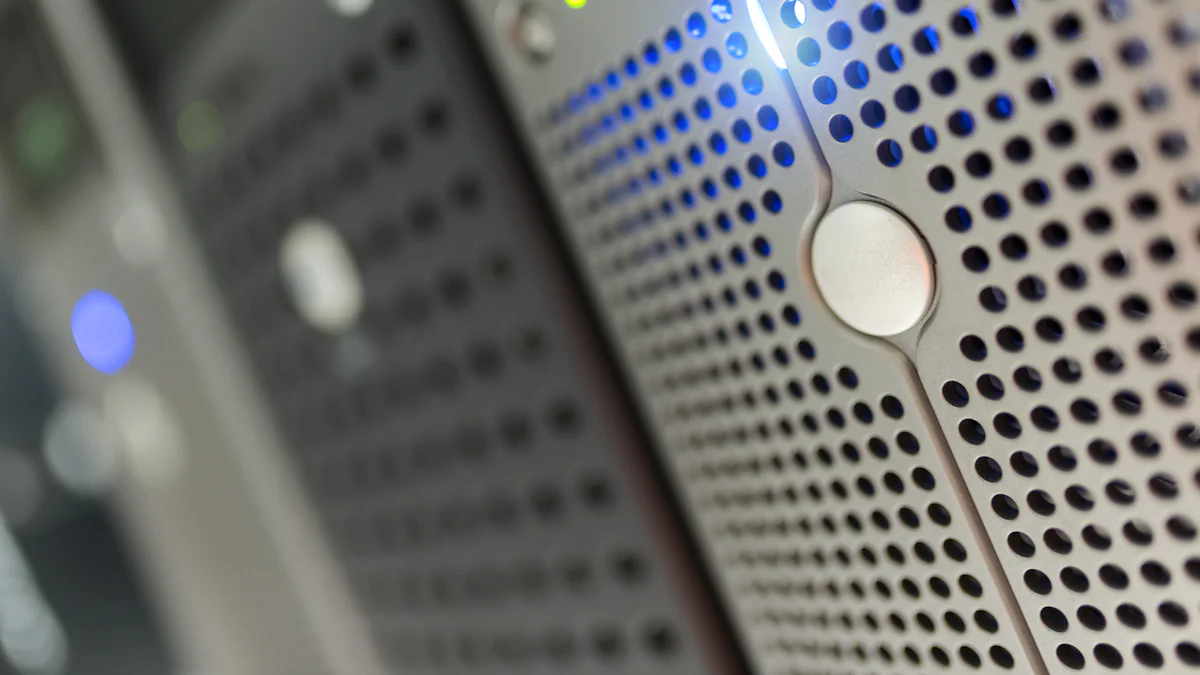
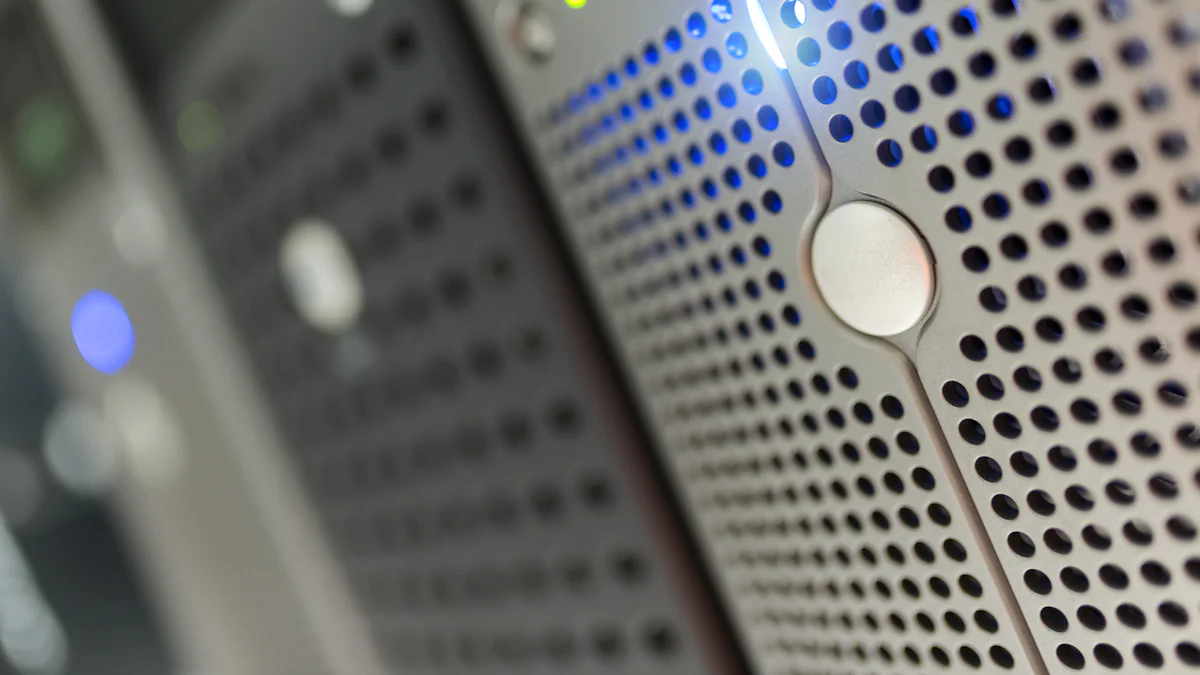
In the world of data center jobs, each role plays a vital part in ensuring smooth operations. Let’s dive into some of the key positions you might encounter.
Data Center Technician
Responsibilities
As a Data Center Technician, you are the backbone of the data center’s daily operations. Your main tasks include installing and maintaining servers and network equipment. You also monitor the performance of these systems to ensure everything runs smoothly. It’s your job to manage environmental controls, like temperature and humidity, to keep hardware in top shape. You also keep an eye on power supply systems to prevent unexpected failures.
Required Skills
To excel in this role, you need strong technical skills and a keen eye for detail. You should be passionate about technology and have a solid understanding of server environments. Analytical skills are crucial, as you’ll often troubleshoot issues and find solutions quickly. Being hands-on with equipment is a must, so practical experience is highly valued.
Data Center Technicians: “Their role is paramount in sustaining the seamless flow of data center operations, encompassing the management of environmental controls to ensure optimal hardware performance and the vigilant monitoring of power supply systems to mitigate any risk of unexpected failures.”
Career Progression
Starting as a Data Center Technician opens doors to various career paths. With experience, you can move up to roles like Data Center Engineer or even Data Center Manager. Continuous learning and gaining certifications can accelerate your career growth in this field.
Data Center Engineer
Responsibilities
As a Data Center Engineer, you design and implement the infrastructure that keeps data centers running efficiently. You work on complex systems, ensuring they meet the organization’s needs. Your role involves optimizing performance, managing capacity, and ensuring the reliability of data center operations.
Required Skills
You need a deep understanding of network and operating systems. Technical competence in your domain is essential. Strong problem-solving skills and attention to detail are crucial. You should also be comfortable working with various technologies and adapting to new ones as they emerge.
Terence Lim, Data Centers Expert: “The data centers, being a critical environment, requires a person who has a high level of professionalism, is passionate about technology, carries a strong analytical skill with attention to detail, and the technical competence in their domain.”
Career Progression
With experience, you can advance to senior engineering roles or transition into management positions. Specializing in areas like network engineering or systems architecture can also enhance your career prospects.
Data Center Manager
Responsibilities
As a Data Center Manager, you oversee the entire facility’s operations. You ensure everything runs smoothly, from managing staff to maintaining equipment. Your role involves strategic planning, budgeting, and implementing best practices to optimize efficiency.
Required Skills
A broad knowledge of data center operations is essential. You need to understand network and operating systems, as well as protocols and processes. Leadership skills are crucial, as you’ll manage a team and coordinate various activities.
“Overseeing the general running of the facilities, it’s imperative that data center managers have a wide range of knowledge of all things data center-related – from understanding about network and operating systems to knowing the correct protocols and processes.”
Career Progression
Data Center Managers can move into higher-level management roles or specialize in areas like data center design or consultancy. Continuous learning and staying updated with industry trends are key to advancing in this role.
Network Administrator
Responsibilities
As a Network Administrator, you play a crucial role in maintaining the data center’s network infrastructure. Your primary responsibilities include configuring and managing network hardware, such as routers and switches. You ensure that the network operates efficiently and securely. Monitoring network performance and troubleshooting issues are also part of your daily tasks. You work to prevent downtime and maintain seamless connectivity across the data center.
Required Skills
To excel as a Network Administrator, you need a solid understanding of networking protocols and systems. Familiarity with network security measures is essential. You should possess strong problem-solving skills and the ability to work under pressure. Communication skills are also important, as you often collaborate with other IT professionals to resolve network-related issues.
Expert Testimony: “The interviewee should demonstrate their working knowledge of the data center environment, including managing servers, data center infrastructure, and understanding basic networking and data protection.”
Career Progression
Starting as a Network Administrator opens doors to advanced roles in network engineering or IT management. With experience, you can specialize in areas like network security or cloud networking. Continuous learning and obtaining certifications, such as Cisco’s CCNA, can enhance your career prospects and lead to leadership positions within data center jobs.
Security Specialist
Responsibilities
As a Security Specialist, you safeguard the data center’s assets from cyber threats. Your responsibilities include implementing security protocols and monitoring for potential breaches. You conduct regular security audits and vulnerability assessments to identify and mitigate risks. Developing and enforcing security policies is also part of your role, ensuring compliance with industry standards.
Required Skills
To thrive as a Security Specialist, you need a deep understanding of cybersecurity principles and practices. Analytical skills are crucial for identifying and addressing security vulnerabilities. You should be detail-oriented and capable of thinking like a hacker to anticipate potential threats. Strong communication skills are necessary for educating staff about security best practices.
Terence Lim, Data Centers Expert: “The data centers, being a critical environment, requires a person who has a high level of professionalism, is passionate about technology, carries a strong analytical skill with attention to detail, and the technical competence in their domain.”
Career Progression
Security Specialists can advance to roles such as Security Manager or Chief Information Security Officer (CISO). Specializing in areas like ethical hacking or forensic analysis can further enhance your career in data center jobs. Staying updated with the latest security trends and obtaining certifications, such as CISSP, can accelerate your career growth.
Challenges in Data Center Jobs
Working in a data center can be rewarding, but it also comes with its own set of challenges. Let’s explore some of the hurdles you might face in this field.
Technical Challenges
In the world of data centers, technical challenges are a daily occurrence. You need to manage complex systems and ensure they run smoothly. This involves troubleshooting performance issues and maintaining network health. As an IT professional, you must have a deep understanding of network hardware and software. Your role often requires configuring security systems and addressing any technical glitches that arise.
IT Professionals in Data Center Industry: “Managing network hardware and software, monitoring network health, and troubleshooting performance issues are crucial tasks for IT professionals.”
Staying updated with the latest technologies is essential. The tech landscape evolves rapidly, and you must adapt to new tools and systems. Foundational IT certifications can help you stay ahead and enhance your technical skillset.
Operational Challenges
Operational challenges in data centers revolve around ensuring seamless day-to-day activities. You need to coordinate various tasks and manage resources efficiently. This includes overseeing the physical elements of network roles and deploying network systems. Your expertise in network management plays a vital role in maintaining operational integrity.
Data Center Technicians: “Mastering technical and interpersonal skills is crucial for data center technicians to handle operational challenges effectively.”
Balancing workloads and meeting deadlines can be demanding. You must prioritize tasks and work collaboratively with your team. Strong organizational skills and attention to detail are key to overcoming operational hurdles.
Security Challenges
Security is a top priority in data centers. You must protect sensitive data from cyber threats and unauthorized access. Implementing robust security protocols and conducting regular audits are essential. As a Security Specialist, you play a critical role in safeguarding the data center’s assets.
IT Professionals in Data Center Industry: “Configuring security systems and monitoring for potential breaches are vital responsibilities for IT professionals.”
Staying vigilant and proactive is crucial. You need to anticipate potential threats and address vulnerabilities promptly. Continuous learning and obtaining certifications in cybersecurity can enhance your ability to tackle security challenges effectively.
Navigating these challenges requires a combination of technical expertise, operational efficiency, and a strong focus on security. By honing your skills and staying informed, you can overcome these obstacles and thrive in the dynamic world of data centers.
Industry Trends and Future Opportunities
Emerging Technologies
In the fast-paced world of data centers, emerging technologies are reshaping how you work. Innovations like artificial intelligence (AI), machine learning, and edge computing are becoming integral to data center operations. AI and machine learning help automate processes, making your job more efficient. They can predict equipment failures before they happen, saving time and resources.
Edge computing is another game-changer. It brings data processing closer to where it’s generated, reducing latency and improving performance. This technology is crucial for applications that require real-time data processing, like autonomous vehicles and smart cities. As these technologies evolve, they create new opportunities for you to specialize and advance in your career.
Sustainability and Green Data Centers
Sustainability is no longer just a buzzword; it’s a necessity. Data centers consume a significant amount of energy, and the industry is under pressure to reduce its carbon footprint. You might find yourself working in a facility that prioritizes green practices. This includes using renewable energy sources, optimizing cooling systems, and implementing energy-efficient designs.
Green data centers not only benefit the environment but also reduce operational costs. By embracing sustainable practices, you contribute to a more eco-friendly future. This trend opens up opportunities for you to work on innovative projects that focus on sustainability, making your role even more impactful.
The Future of Data Center Careers
The future of data center careers looks promising. As technology continues to advance, the demand for skilled professionals like you will grow. You’ll find opportunities to work with cutting-edge technologies and tackle complex challenges. The industry offers diverse career paths, from technical roles to management positions.
To stay ahead, continuous learning is essential. Obtaining certifications and staying updated with industry trends will enhance your skills and career prospects. The dynamic nature of data centers means you’ll always have something new to learn and explore. Embrace these opportunities, and you’ll find a rewarding and fulfilling career in this ever-evolving field.
You’ve explored the essential roles within data centers and their significant impact on operations. Each position, from technician to manager, plays a vital part in maintaining the backbone of our digital world. As you consider your career path, data centers offer a dynamic field with endless opportunities for growth and innovation. The industry’s focus on sustainability and energy efficiency opens doors to exciting projects that make a difference. Dive deeper into this ever-evolving sector and seize the chance to shape the future of technology. Your journey in data center careers awaits!
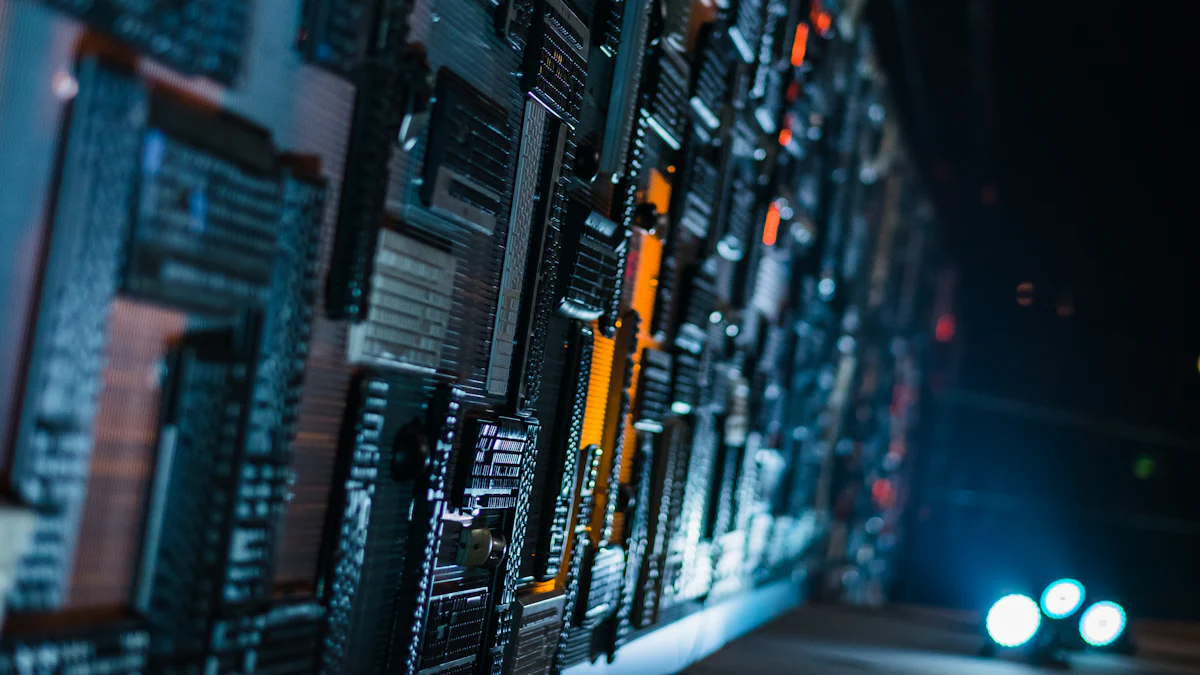
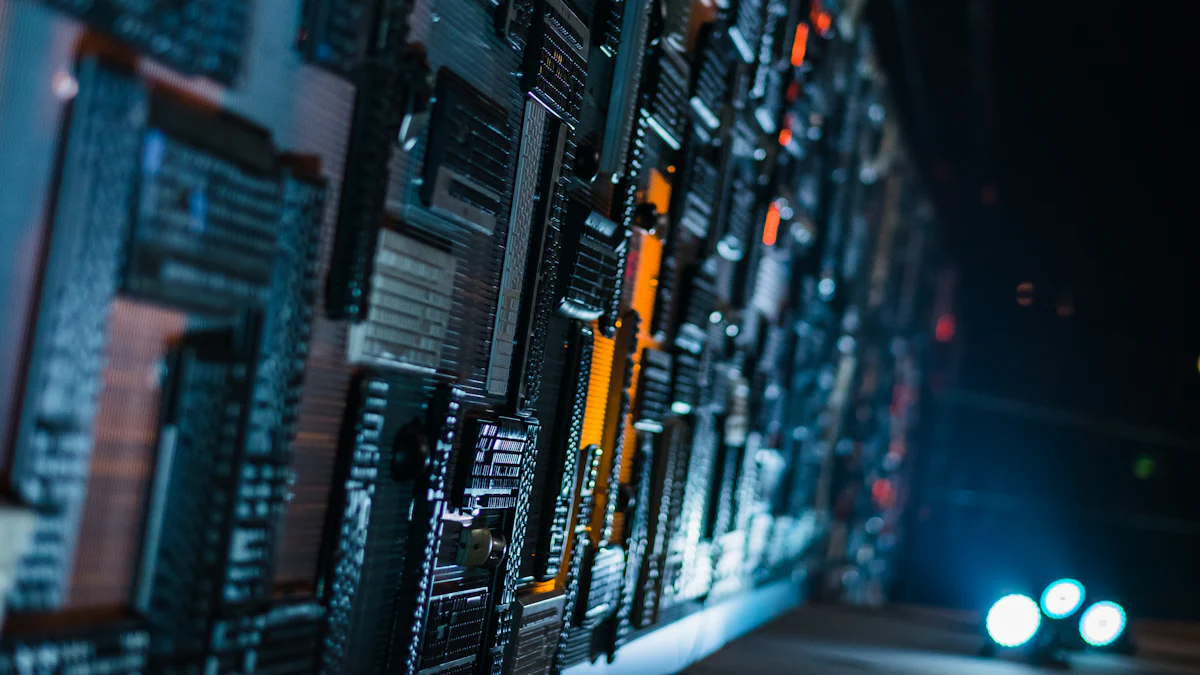
In the fast-paced world of real estate, managing documents efficiently and securely is crucial. You need a solution that not only protects sensitive information but also streamlines your transactions. Enter the data room real estate professionals rely on. These virtual data rooms have revolutionized real estate transactions by offering a secure, efficient, and compliant platform for document management. They simplify processes, enhance collaboration, and improve efficiency. By adopting a data room real estate solution, you can stay ahead in the competitive market, ensuring your transactions are both smooth and secure.
What Are Data Rooms?
In the realm of real estate, a data room serves as a pivotal tool for managing sensitive documents. You might wonder, what exactly is a data room? At its core, a data room is a secure online platform designed to store, manage, and share confidential information. It acts as a digital vault where you can safely keep and access important documents. This is particularly useful in high-stakes transactions like mergers and acquisitions, due diligence, and fundraising. By using a data room real estate professionals can ensure that their sensitive information remains protected while being easily accessible to authorized parties.
Definition and Purpose
A data room provides a controlled environment for document management. Its primary purpose is to facilitate the secure exchange of sensitive information among stakeholders. In real estate, this means you can share property documents, financial records, and legal contracts with potential buyers, investors, or partners without compromising security. The digital nature of data rooms eliminates the need for physical storage, making it easier for you to manage large volumes of documents efficiently. This not only enhances security but also streamlines the entire transaction process.
Types of Data Rooms
Data rooms come in various forms, each tailored to specific needs. The most common type is the Virtual Data Room. This online repository allows you to store and share confidential information securely. VDRs are widely used in real estate transactions due to their ease of access and robust security features. They provide a centralized platform where you can collaborate with internal and external teams, ensuring that everyone has access to the necessary documents.
Another type is the Real Estate Data Room, specifically designed for property transactions. This type of data room offers features that cater to the unique requirements of real estate deals, such as document version control and audit trails. By using a data room real estate professionals can manage their transactions more effectively, reducing the risk of errors and delays.
Enhanced Security
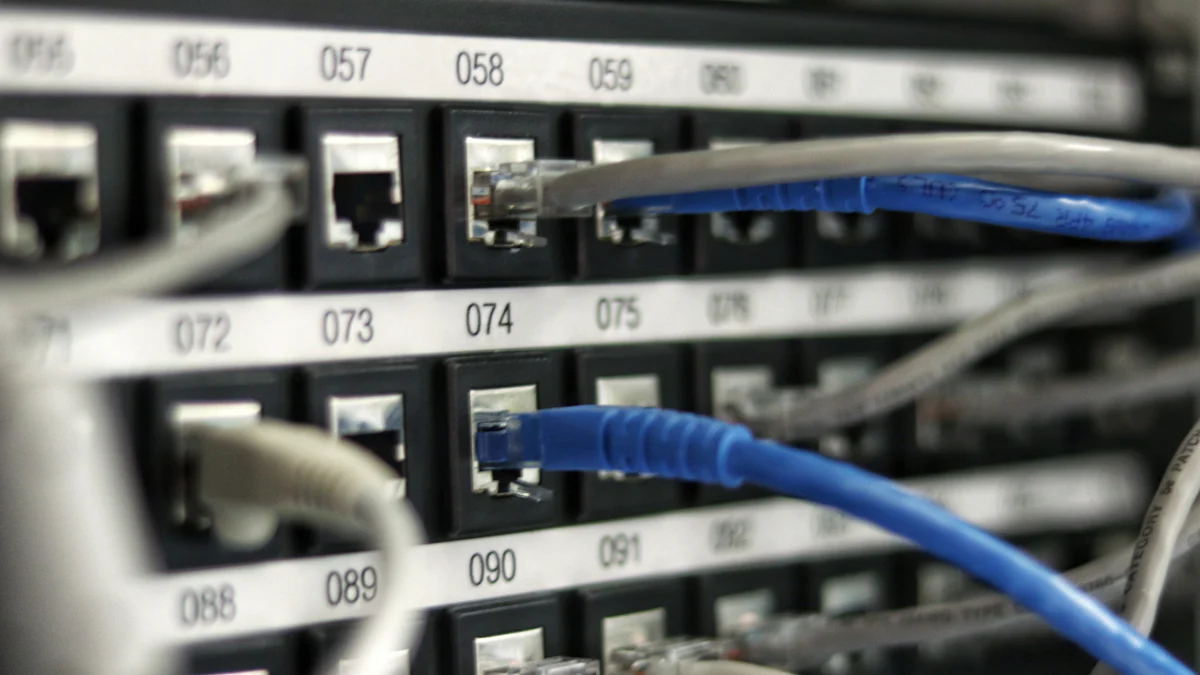
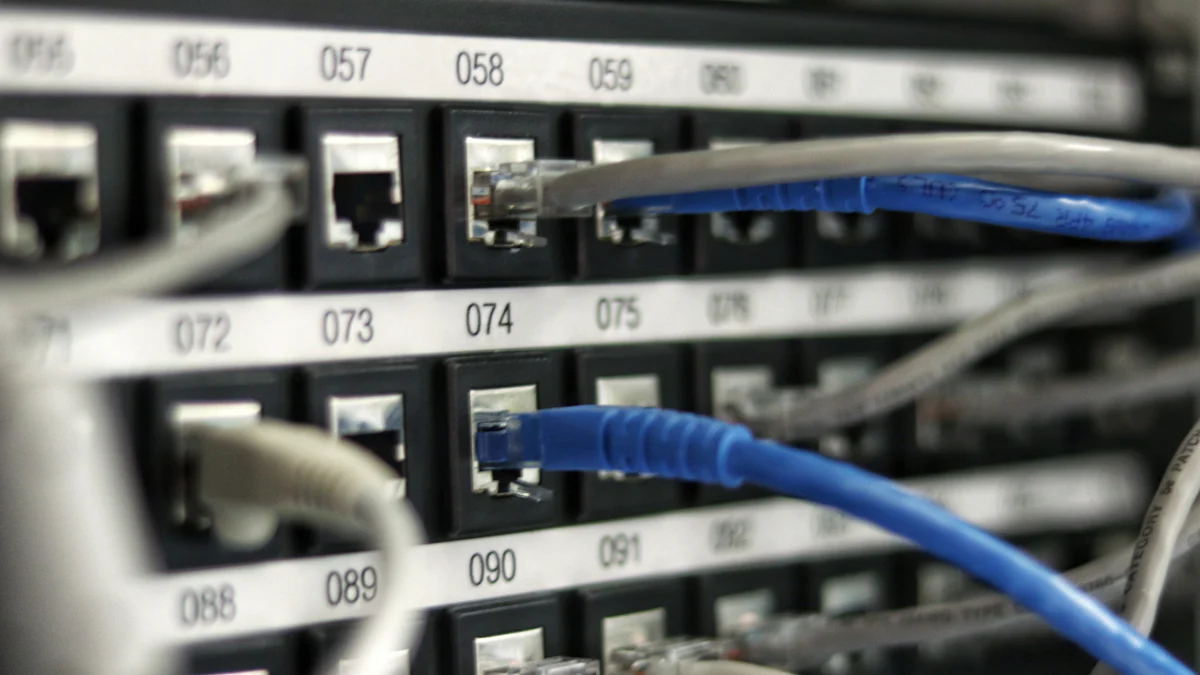
In the realm of real estate, safeguarding sensitive information is paramount. You need a solution that ensures your data remains confidential and protected from unauthorized access. A data room real estate professionals trust provides this level of security. By using virtual data rooms, you can shield your documents from potential breaches and cyberattacks.
Protecting Sensitive Information
Virtual data rooms offer robust security measures to protect your sensitive information. They employ advanced encryption technologies, ensuring that only authorized users can access your documents. This means you can confidently share property details, financial records, and legal contracts without worrying about data breaches. The airtight security provided by data room real estate solutions minimizes the risk of human errors and cyberattacks, which are common causes of data breaches.
“Data breaches can result in severe financial and reputational damage,” experts warn. This highlights the importance of choosing a secure platform for your real estate transactions.
Case Study: Security Breach Prevention
Consider a scenario where a real estate firm avoided a potential security breach by using a virtual data room. The firm had to share sensitive documents with multiple stakeholders during a high-stakes property transaction. By utilizing a data room real estate solution, they ensured that all information remained secure and accessible only to authorized parties. This proactive approach prevented unauthorized access and protected the firm’s reputation and financial interests.
Virtual data rooms not only provide enhanced security but also instill confidence in your clients and partners. By prioritizing the protection of sensitive information, you can focus on closing deals and growing your business without the constant worry of data breaches.
Improved Collaboration
In real estate, collaboration among stakeholders is crucial for successful transactions. A data room real estate solution enhances this collaboration by providing a centralized platform where all parties can access and review documents simultaneously. This eliminates the need for physical meetings and allows for real-time updates and discussions. You can streamline communication, ensuring that everyone stays informed and aligned throughout the process.
Streamlining Communication
Virtual data rooms offer several features that facilitate seamless communication. They include Q&A sections where you can address queries and provide clarifications directly within the platform. This feature ensures that all stakeholders have access to the same information, reducing misunderstandings and promoting transparency. Additionally, audit trails track document access and modifications, holding everyone accountable and maintaining a clear record of interactions.
By using a data room real estate professionals can manage multiple projects simultaneously. You can securely share files and communicate with partners and investors, keeping everyone on the same page. This streamlined communication helps you stay competitive in the fast-paced real estate market.
Case Study: Successful Team Collaboration
Consider a real estate firm that successfully managed a complex property transaction using a data room real estate solution. The firm had multiple stakeholders, including investors, legal advisors, and potential buyers. By utilizing a data room real estate solution, they provided all parties with access to necessary documents and facilitated real-time discussions through the platform’s Q&A section.
This approach eliminated the need for numerous physical meetings and ensured that everyone received timely updates. The audit trail feature allowed the firm to track document access and modifications, ensuring accountability and transparency. As a result, the transaction proceeded smoothly, with all stakeholders aligned and informed at every stage.
Incorporating a data room real estate solution into your workflow can significantly enhance collaboration. By streamlining communication and providing a secure platform for document sharing, you can ensure that your real estate transactions are efficient and successful.
Faster Transactions
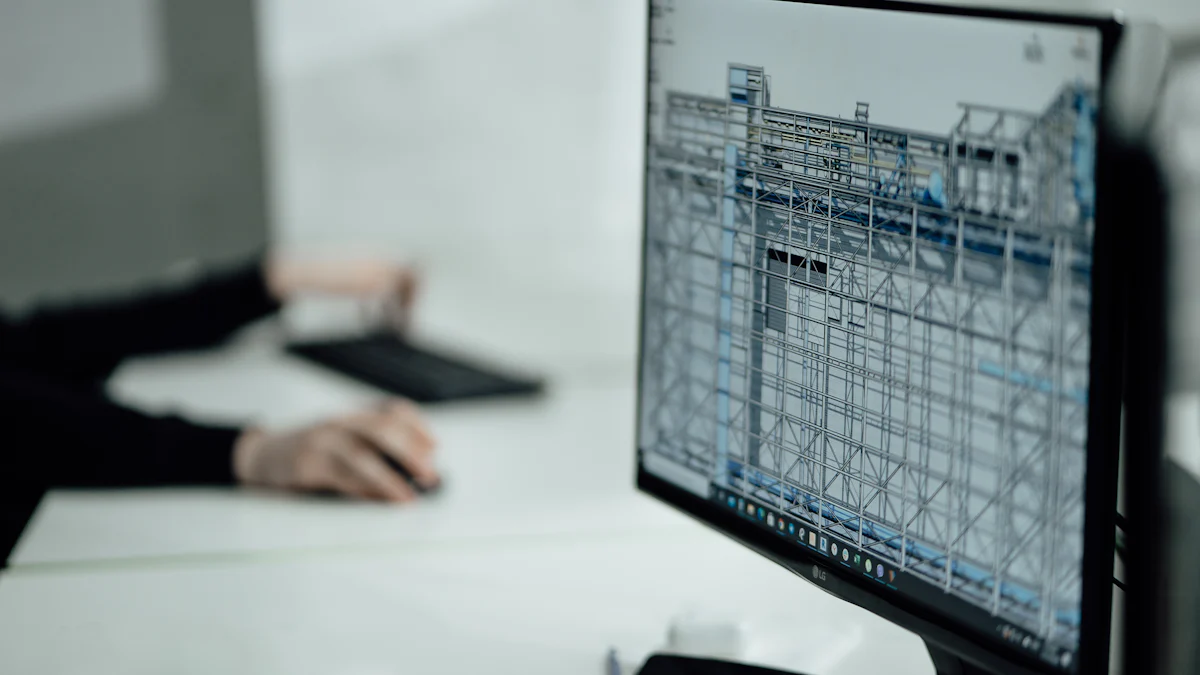
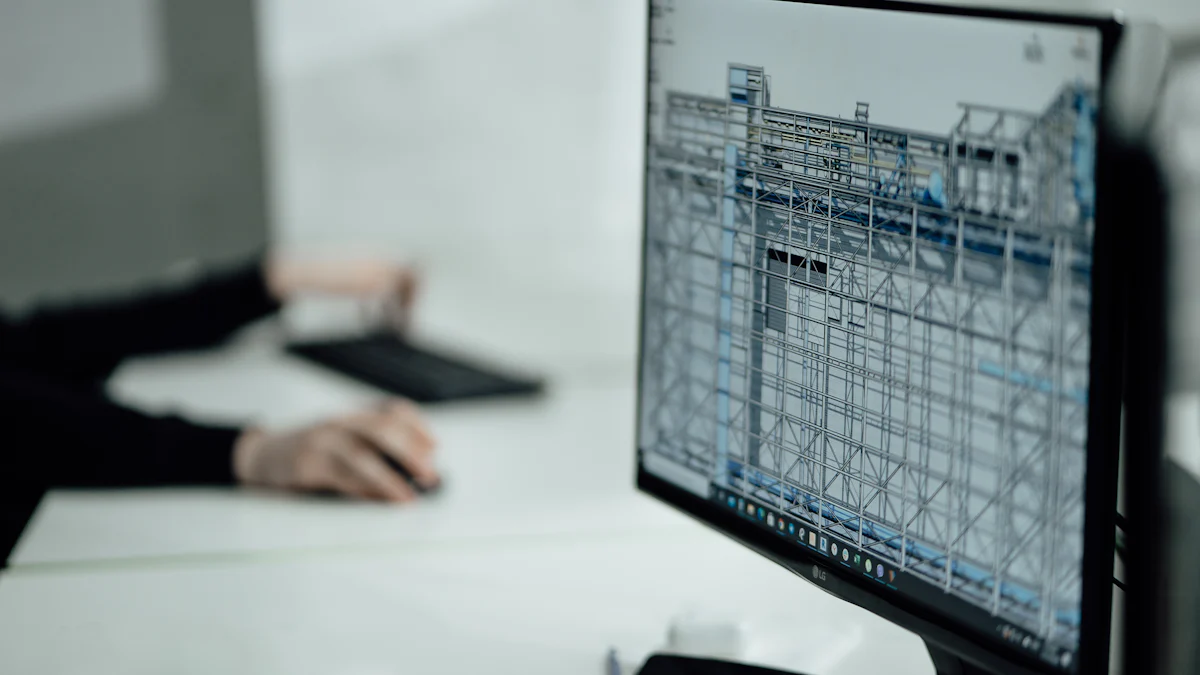
In real estate, time is of the essence. You need to close deals quickly to stay competitive. Virtual data rooms help you achieve this by significantly reducing transaction times.
Reducing Time Delays
Virtual data rooms streamline the transaction process. They provide a centralized platform for document management. You can access and share documents instantly. This eliminates the need for physical meetings and courier services. By using a virtual data room, you reduce the time spent on administrative tasks. This allows you to focus on closing deals.
Consider this: Transactions using virtual data rooms are 30% shorter than those without. This efficiency comes from the ability to manage documents in real-time. You can make quick decisions and respond promptly to inquiries. This reduces delays and keeps the transaction moving forward.
Case Study: Accelerated Deal Closure
Imagine a real estate firm facing a tight deadline for a property sale. The firm used a virtual data room to manage the transaction. They uploaded all necessary documents to the platform. Stakeholders accessed the documents simultaneously. This allowed for real-time discussions and quick decision-making.
The firm completed the transaction ahead of schedule. The virtual data room facilitated faster communication and document sharing. This accelerated the deal closure and satisfied all parties involved.
By adopting a virtual data room, you can achieve similar results. Faster transactions mean more opportunities and increased profitability. You stay ahead in the competitive real estate market.
Cost Efficiency
In the competitive world of real estate, managing costs effectively is crucial. Virtual data rooms offer a cost-efficient solution for document management and transaction processes. By adopting these digital platforms, you can significantly reduce operational expenses.
Reducing Operational Costs
Virtual data rooms eliminate the need for physical storage and paper-based processes. You no longer have to spend on printing, courier services, or maintaining physical archives. This shift to digital not only saves money but also streamlines your operations.
Key Benefits:
Reduced Printing Costs: All documents are stored digitally, eliminating the need for printing.
No Courier Fees: Instant document sharing means no more courier services.
Lower Storage Expenses: Digital storage replaces costly physical archives.
Real estate professionals have found virtual data rooms to be a reliable and cost-effective method for storing and sharing critical data. These platforms simplify business processes and allow you to control and analyze buyer interest through real-time statistics. This efficiency saves time and resources for you, your buyers, investors, and partners.
Case Study: Cost Savings in Real Estate
Consider a real estate firm that transitioned from traditional data management to a virtual data room. The firm previously spent a significant amount on printing and courier services for document distribution. By switching to a virtual data room, they eliminated these costs entirely.
The firm also benefited from reduced storage expenses. They no longer needed to maintain a physical archive, which required space and security measures. The digital platform provided a secure and organized environment for all their documents.
“Virtual data rooms have gained the trust of many big real estate firms,” notes a survey of real estate professionals. These platforms have proven to simplify business processes and save resources.
By adopting a virtual data room, you can achieve similar cost savings. This approach not only reduces expenses but also enhances your operational efficiency. In the fast-paced real estate market, cost efficiency can give you a competitive edge.
Choosing the Right Data Room Provider
Selecting the right data room real estate provider is crucial for ensuring smooth and secure transactions. With numerous options available, you must carefully evaluate each provider to find the one that best suits your needs.
Key Features to Consider
When choosing a data room real estate solution, focus on key features that enhance security, usability, and efficiency. Here are some essential features to look for:
Security Protocols: Ensure the provider offers robust security measures, such as encryption and two-factor authentication, to protect sensitive information.
User-Friendly Interface: A simple and intuitive interface allows you to navigate the platform easily, reducing the learning curve for your team.
Document Management Tools: Look for features like version control, audit trails, and bulk upload capabilities to streamline document handling.
Collaboration Features: Tools like Q&A sections and real-time notifications facilitate seamless communication among stakeholders.
Customization Options: The ability to tailor the platform to your specific needs can enhance its effectiveness in managing data room real estate transactions.
Evaluating Providers
To ensure you choose the best data room real estate provider, conduct a thorough evaluation. Here are steps to guide you:
Research Reputation: Investigate the provider’s reputation by reading user reviews and expert opinions. Platforms like G2, TrustRadius, and Capterra offer valuable insights into the provider’s performance in terms of ease of use, security, and support.
“Always research the reputation of VDR providers by reading user reviews and expert opinions,” advise the DealRoom Experts. This step helps you understand how the provider performs in real-world scenarios.
Test the Platform: Take advantage of trial periods to test the platform’s capabilities. During this time, explore all features and assess their functionality.
The Data Room Rating Experts recommend conducting a “crash test” during the trial period. This involves testing the platform’s work and capabilities in every possible way to ensure it meets your needs.
Assess Customer Support: Evaluate the provider’s customer support services. Prompt and effective support can be crucial during critical transactions.
Compare Pricing: Analyze the pricing structure to ensure it aligns with your budget. Consider both the initial costs and any additional fees for extra features or support.
By carefully considering these factors, you can select a data room real estate provider that enhances your transaction processes, ensuring security and efficiency.
Data room real estate solutions offer numerous benefits. They enhance security, improve collaboration, and expedite transactions. By choosing the right provider, you ensure these advantages align with your specific needs. Conduct a thorough trial period to test the platform’s capabilities. This step guarantees the data room real estate solution meets your expectations. Consider integrating data rooms into your real estate transactions. They provide a secure and efficient way to manage documents, keeping you competitive in the market. Embrace this technology to streamline your processes and protect sensitive information.
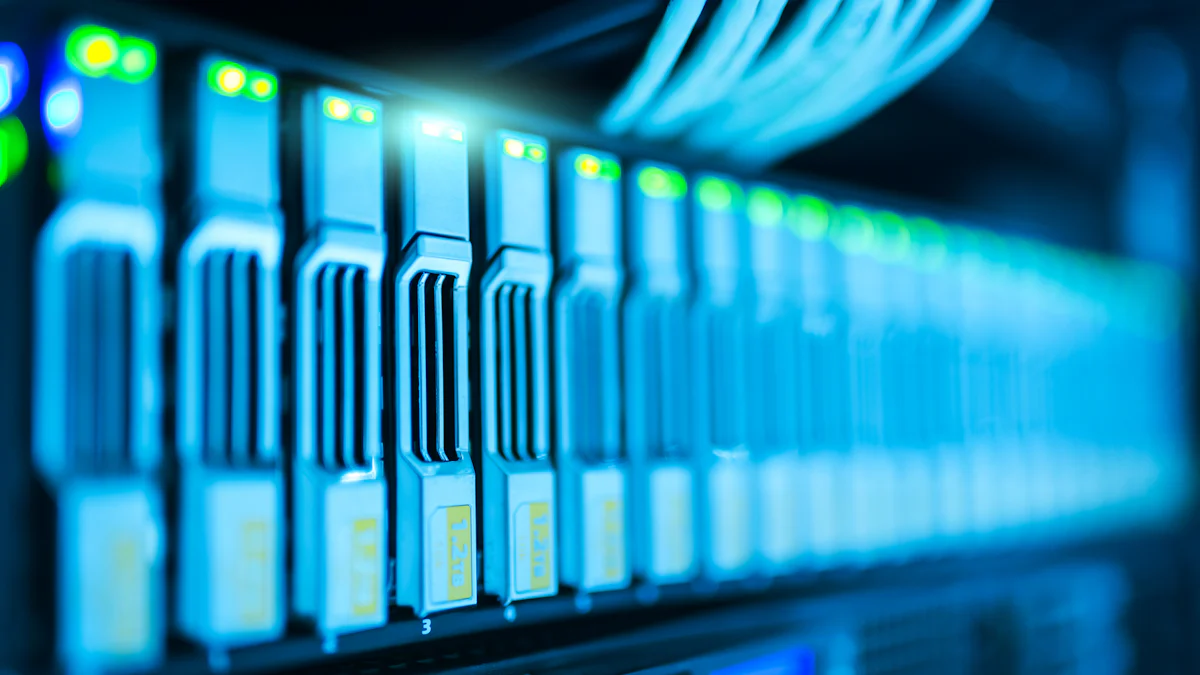
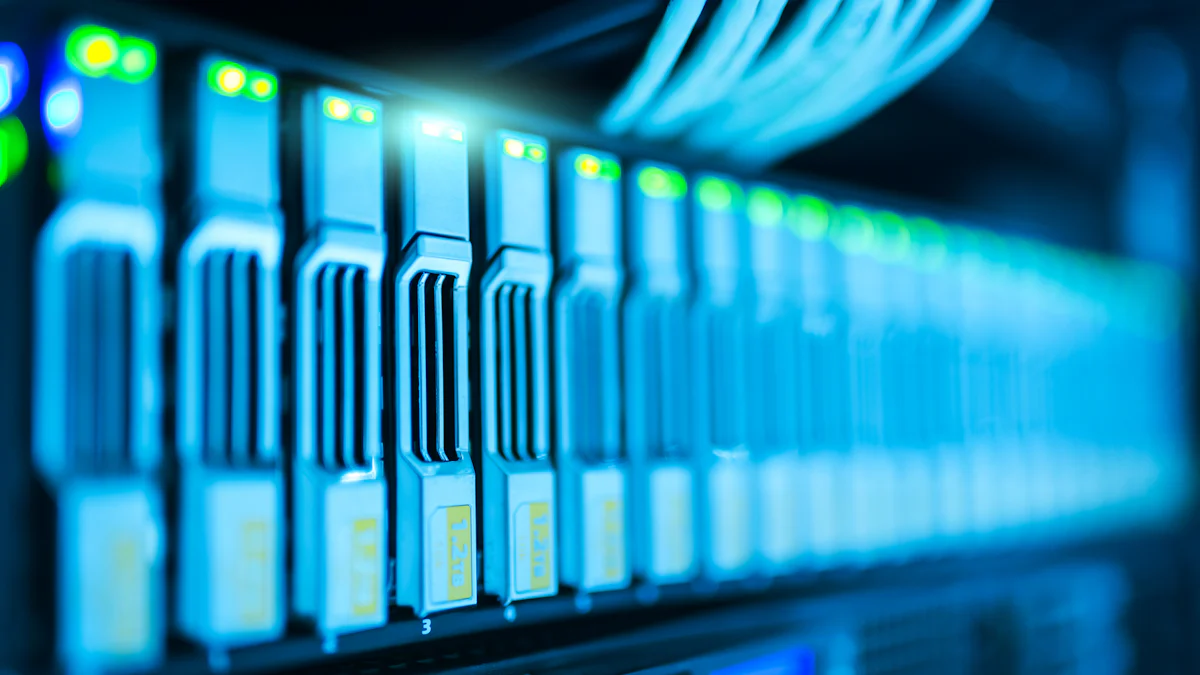
Securing data centers has become paramount in today’s digital landscape. With a staggering 72% increase in security breaches since 2021, the need for robust data center security best practices cannot be overstated. Data breaches have exposed over 42 million records between March 2021 and February 2022, impacting more than 349 million people in 2023 alone. The average cost of a data breach has surged to $4.35 million, highlighting the severe financial consequences of inadequate security measures. Implementing data center security best practices is essential to mitigate these risks and safeguard sensitive information effectively.
Implementing Multi-Factor Authentication (MFA)
Understanding MFA
Definition and Importance of MFA
Multi-Factor Authentication (MFA) serves as a critical component in data center security best practices. It requires users to provide multiple forms of verification before granting access. This method significantly reduces the risk of unauthorized access, even if a password is compromised. Maxim, a security expert, emphasizes, “MFA is one of the single most effective security controls available.” By implementing MFA, organizations can protect sensitive data and maintain robust security protocols.
Types of MFA Methods
MFA employs various methods to verify user identity. Common types include:
Something You Know: This involves passwords or PINs.
Something You Have: This includes devices like smartphones or security tokens.
Something You Are: This refers to biometric verification, such as fingerprint or facial recognition.
Each method adds a layer of security, making it harder for attackers to gain unauthorized access. Nick Biasini, head of outreach at Cisco Talos, notes, “Basic MFA with SMS-based notification is the least secure, but better than no MFA at all.”
Steps to Implement MFA
Assessing Current Authentication Systems
Organizations must first evaluate their existing authentication systems. This assessment helps identify vulnerabilities and areas for improvement. By understanding the current setup, companies can determine the most suitable MFA solutions to enhance their data center security best practices.
Selecting Appropriate MFA Solutions
Choosing the right MFA solution involves considering the organization’s specific needs and security requirements. Murtaza Hafizji, a product marketing consultant, suggests combining elements like one-time passwords, mobile authenticator apps, and biometric analysis. This combination ensures secure access to applications, regardless of their location. Implementing MFA across all access points, from physical entry to administrative logins, strengthens the overall security framework.
Role-Based Access Control (RBAC)
Overview of RBAC
Definition and Benefits of RBAC
Role-Based Access Control (RBAC) stands as a cornerstone in data center security best practices. It assigns permissions to users based on their roles within an organization. This method streamlines access management by grouping permissions, which reduces the complexity of managing individual user rights. By implementing RBAC, organizations can enhance security, improve compliance, and reduce administrative overhead. Gartner, a leading research and advisory company, states, “RBAC is a proven approach to managing user access, offering both simplicity and scalability.”
Comparison with Other Access Control Models
RBAC differs significantly from other access control models. In comparison to Discretionary Access Control (DAC), RBAC centralizes permission management, while DAC relies on individual user permissions. This centralization makes RBAC more efficient for larger organizations. When compared to Access Control Lists (ACL), RBAC simplifies management by associating permissions with roles rather than individual users. Additionally, Attribute-Based Access Control (ABAC) offers more granular control using attributes, but RBAC remains simpler and is often preferred by small to medium-sized organizations. These distinctions highlight RBAC’s effectiveness in data center security best practices.
Implementing RBAC
Identifying Roles and Permissions
Implementing RBAC begins with identifying roles within the organization. Each role should align with specific job functions and responsibilities. Once roles are defined, organizations must assign appropriate permissions to each role. This process ensures that users have access only to the resources necessary for their duties, thereby enhancing data center security best practices. NIST, the National Institute of Standards and Technology, recommends a thorough analysis of organizational roles to ensure accurate permission assignments.
Regularly Reviewing and Updating Roles
Regular reviews and updates of roles and permissions are crucial for maintaining effective RBAC. As organizations evolve, roles and responsibilities may change, necessitating adjustments in access controls. Regular audits help identify outdated or unnecessary permissions, reducing the risk of unauthorized access. By continuously refining RBAC policies, organizations can uphold robust data center security best practices and adapt to changing security landscapes.
Conducting Regular Security Audits
Importance of Security Audits
Regular security audits play a pivotal role in maintaining data center security best practices. They help organizations identify vulnerabilities and threats that could compromise sensitive information. By conducting thorough assessments, companies can pinpoint weaknesses in their security infrastructure and take corrective measures. This proactive approach minimizes the risk of data breaches and ensures the integrity of data center operations.
Security audits also ensure compliance with regulations. Many industries, such as financial services, must adhere to strict data protection standards. For instance, the Gramm-Leach-Bliley Act (GLBA) mandates high standards for safeguarding financial information. Similarly, the Payment Card Industry Data Security Standard (PCI DSS) requires stringent security measures for processing payment card data. Non-compliance with these regulations can result in severe penalties and damage to an organization’s reputation. Therefore, regular audits are essential for verifying adherence to these standards and maintaining client trust.
Steps for Effective Audits
To conduct effective security audits, organizations should follow a structured approach. The first step involves planning and scheduling audits. Companies must determine the scope of the audit, identify the systems and processes to be evaluated, and set a timeline for completion. This preparation ensures a comprehensive assessment of the data center’s security posture.
Once the audit is underway, analyzing audit results becomes crucial. Organizations should meticulously review the findings to identify areas of concern. This analysis helps prioritize security improvements and allocate resources effectively. Implementing changes based on audit results strengthens data center security best practices and enhances overall protection.
Incorporating industry standards, such as SOC 2 (Service Organization Control 2), can further bolster audit effectiveness. SOC 2 focuses on trust services principles, ensuring that data centers demonstrate a commitment to protecting customer data. By aligning audits with these standards, organizations can assure customers of their information security and internal controls.
Ensuring Physical Security
Key Elements of Physical Security
Access Control Systems
Access control systems form the backbone of physical security in data centers. They ensure that only authorized personnel can enter sensitive areas. These systems often incorporate Access control systems biometric scanners and keycard access, providing multiple layers of verification. For instance, initial entry might require a fingerprint scan, followed by a keycard swipe. This dual-layer approach significantly reduces the risk of unauthorized access. Security personnel play a crucial role in monitoring these systems, ensuring that all entries and exits are logged and verified.
Surveillance and Monitoring
Surveillance and monitoring systems act as vigilant watchdogs for data centers. High-definition video surveillance systems cover both indoor and outdoor areas, providing real-time monitoring. These systems integrate with building alarm systems to alert security personnel of any unauthorized access attempts. Intrusion detection systems (IDS) further enhance security by identifying unusual activities and triggering alerts. Security officers continuously monitor these feeds, ensuring immediate response to potential threats. This comprehensive surveillance network acts as a deterrent to unauthorized access and helps maintain the integrity of the data center.
Best Practices for Physical Security
Securing Entry Points
Securing entry points is vital for maintaining robust physical security. Data centers often employ perimeter security measures such as tall fences made of steel and concrete. These barriers prevent unauthorized access and protect the facility from external threats. Camera-monitored entrance gates and security guard patrols further ensure that only authorized personnel can enter. Additionally, bollards and other protective measures safeguard the exterior from potential vehicular threats. By fortifying entry points, data centers can effectively control access and enhance overall security.
Implementing Environmental Controls
Environmental controls play a significant role in safeguarding data centers. These controls regulate temperature, humidity, and airflow, ensuring optimal conditions for equipment operation. Proper environmental management prevents overheating and reduces the risk of equipment failure. Additionally, fire suppression systems and smoke detectors provide critical protection against fire hazards. Regular maintenance and testing of these systems ensure their effectiveness. By implementing robust environmental controls, data centers can protect their infrastructure and maintain uninterrupted operations.
Network Security Measures
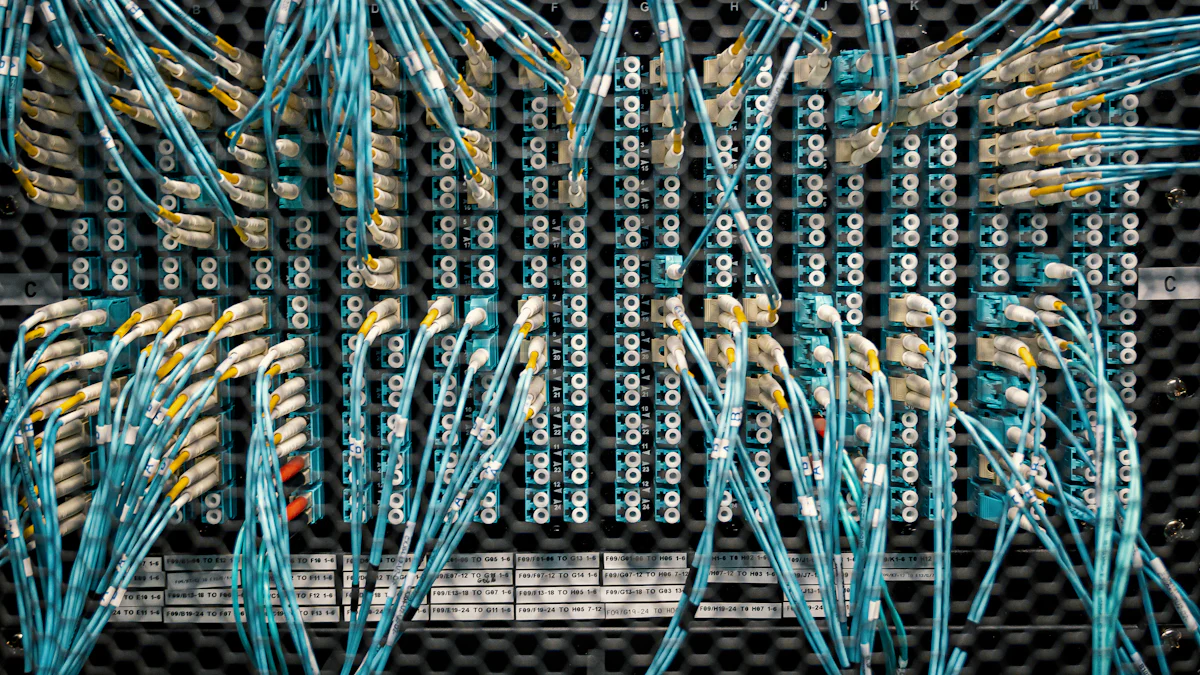
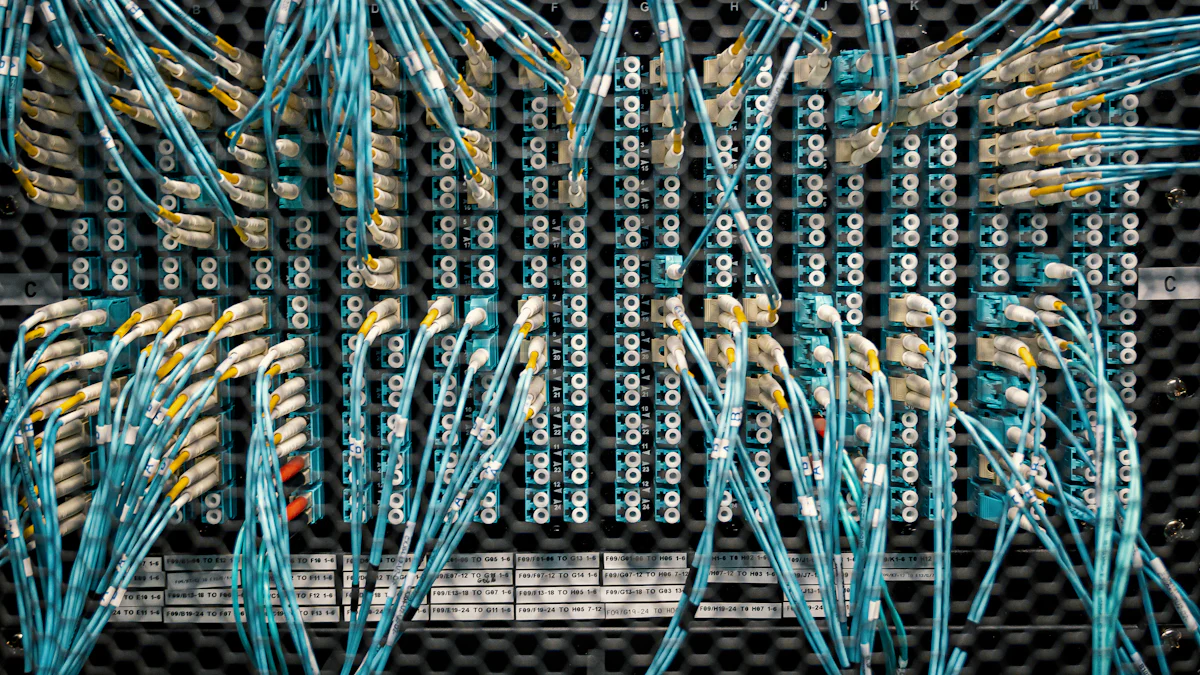
Protecting Network Infrastructure
Firewalls and Intrusion Detection Systems
Firewalls serve as the first line of defense in network security. They monitor incoming and outgoing traffic, blocking unauthorized access while allowing legitimate communication. Organizations should configure firewalls to minimize vulnerabilities and protect sensitive data. Intrusion Detection Systems (IDS) complement firewalls by identifying suspicious activities within the network. IDS analyze traffic patterns and alert security teams to potential threats. By deploying both firewalls and IDS, organizations can create a robust security framework that safeguards their network infrastructure.
Network Segmentation
Network segmentation divides a network into smaller, isolated segments. This approach limits the spread of potential threats and enhances security. Each segment operates independently, reducing the risk of a single breach compromising the entire network. Organizations should implement segmentation to protect sensitive data and critical systems. By isolating these assets, they can prevent unauthorized access and minimize the impact of security incidents. Network segmentation also improves performance by reducing congestion and optimizing resource allocation.
Best Practices for Network Security
Regularly Updating and Patching Systems
Regular updates and patches are crucial for maintaining network security. Software vendors release patches to address vulnerabilities and improve system performance. Organizations must prioritize timely updates to protect against emerging threats. Delayed patching can leave systems exposed to cyberattacks. Security teams should establish a patch management process to ensure all systems remain up-to-date. This proactive approach reduces the risk of exploitation and strengthens the overall security posture.
Monitoring Network Traffic
Continuous monitoring network traffic is essential for detecting anomalies and potential threats. Security teams should use advanced tools to analyze traffic patterns and identify unusual activities. Real-time monitoring enables quick response to security incidents, minimizing damage and preventing data breaches. Organizations should implement automated monitoring solutions to enhance efficiency and accuracy. By maintaining vigilant oversight of network traffic, they can ensure the integrity and security of their data center operations.
Data Encryption and Protection
Importance of Data Encryption
Protecting Data at Rest and in Transit
Data encryption stands as a cornerstone in data center security best practices. It ensures that sensitive information remains confidential and inaccessible to unauthorized users. Encrypting data both at rest and in transit is crucial. At rest, data resides on storage devices, while in transit, it moves across networks. IT professionals emphasize the importance of using robust encryption algorithms to protect data privacy. They recommend Advanced Encryption Standard (AES) for data at rest and Transport Layer Security (TLS) for data in transit. These encryption methods prevent unauthorized access and breaches, safeguarding valuable assets. importance of data center security
Encryption Standards and Protocols
Adhering to encryption standards and protocols forms an integral part of data center security best practices. Organizations must implement up-to-date encryption algorithms to ensure data confidentiality and integrity. IT professionals highlight the significance of secure data transmission protocols, such as TLS, to protect data during transfer. Compliance with regulations like the General Data Protection Regulation (GDPR) mandates encryption to minimize data breach risks. By following these standards, businesses can maintain robust security measures and protect sensitive information effectively.
Implementing Data Protection Strategies
Backup and Recovery Solutions
Implementing backup and recovery solutions is vital for data protection. Regular backups ensure that data remains available even in the event of a breach or system failure. Organizations should establish a comprehensive backup strategy that includes both on-site and off-site storage. This approach provides redundancy and enhances data center security best practices. IT professionals recommend testing recovery procedures regularly to ensure data can be restored quickly and efficiently. By prioritizing backup and recovery, businesses can minimize downtime and maintain operational continuity. cutting-edge cybersecurity tools
Data Loss Prevention (DLP) Tools
Data Loss Prevention (DLP) tools play a critical role in safeguarding sensitive information. These tools monitor data flows and prevent unauthorized access or transmission. DLP solutions help organizations identify and protect confidential data, ensuring compliance with data protection regulations. IT professionals advocate for the integration of DLP tools into existing security frameworks to enhance data center security best practices. By implementing DLP solutions, businesses can prevent data breaches and protect their valuable assets from unauthorized access.
Implementing comprehensive data center security best practices is crucial in today’s digital age. Organizations must regularly assess their security measures to identify vulnerabilities and make necessary improvements. Continuous monitoring plays a vital role in maintaining data safety and ensuring prompt threat detection. Security management requires ongoing vigilance, as threats constantly evolve. Staying informed about emerging risks allows organizations to adapt their strategies effectively. By prioritizing data center security best practices, companies can protect sensitive information and maintain robust security controls. This proactive approach not only mitigates risks but also ensures the integrity of data center operations.
Building a data center from scratch can seem daunting, but understanding each step is crucial. When you build a data center, it’s important to consider the growing demand for these facilities, which now account for about 1-1.5% of global power consumption. This figure is expected to rise, highlighting the importance of efficient design. A well-executed project not only meets your current needs but also adapts to future demands. By focusing on efficiency and sustainability, you can build a data center that supports your business objectives while minimizing environmental impact.
Assessing Needs
Before you build a data center, you must assess your needs thoroughly. This step ensures that your data center aligns with your business objectives and can adapt to future demands. Proper evaluation of both present and anticipated needs is crucial for designing a facility that grows with your business.
Define Purpose and Scope
Identify Business Objectives
Start by identifying your business objectives. Ask yourself what you aim to achieve with the data center. Do you need it for data storage, processing, or both? Understanding your goals will guide the entire project. It helps you focus on what’s essential and avoid unnecessary features that could inflate costs.
Determine Capacity Requirements
Next, determine your capacity requirements. Consider the volume of data you expect to handle now and in the future. Right-sizing your data center is vital. It prevents overspending on space and resources you don’t need. By managing demand effectively, you can optimize costs and ensure your data center remains efficient.
Establish Requirements
Technical Specifications
Once you’ve defined your purpose and scope, establish the technical specifications. These include the types of servers, storage solutions, and networking equipment you’ll need. Ensure that your choices support scalability. This way, your data center can accommodate growth without major overhauls.
Budget Constraints
Finally, consider your budget constraints. Building a data center involves significant investment. You must balance your technical needs with financial realities. Prioritize essential features and explore cost-saving strategies. For instance, demand management can help reduce unnecessary expenses. By planning strategically, you can build a data center that meets your needs without breaking the bank.
Designing Infrastructure
Designing the infrastructure of your data center is a critical step. It involves making decisions that will impact the efficiency, scalability, and reliability of your facility. Let’s explore the key components of this process.
Location Selection
Choosing the right location for your data center is essential. You need to consider several factors to ensure optimal performance and accessibility.
Geographic Considerations
When selecting a location, think about geographic factors. Areas prone to natural disasters like earthquakes or floods may pose risks. Choose a site with a stable climate to minimize environmental challenges. This decision can significantly affect the long-term reliability of your data center.
Accessibility and Connectivity
Accessibility is another crucial factor. Your data center should be easily reachable for maintenance and upgrades. Additionally, ensure robust connectivity. Proximity to major network hubs can enhance data transfer speeds and reduce latency. This ensures seamless operations and better service delivery.
Design Standards
Adhering to design standards helps you create a data center that meets industry benchmarks. This ensures your facility remains competitive and efficient.
Industry Best Practices
Follow industry best practices when designing your data center. These include using modular designs and implementing energy-efficient technologies. Schneider Electric Data Center Networking Solutions offer tools that optimize functionality while reducing energy usage. By adopting such solutions, you can enhance the overall quality of your data center.
Scalability and Flexibility
Design your data center with scalability in mind. Data Center Scalability and Equipment Layout emphasize the importance of accommodating future growth. Plan equipment locations and layouts to allow for easy expansion. This approach prevents costly redesigns and ensures your data center can handle increased workloads.
Power and Cooling
Power and cooling systems are vital for maintaining operational stability. They ensure your data center runs efficiently and reliably.
Energy Efficiency
Focus on energy efficiency when selecting power and cooling equipment. Power Supply and Cooling Equipment for Data Centers includes efficient UPS systems and precision cooling units. These solutions, with smart adaptive controls, can reduce operational costs and maintain optimal conditions. Energy storage systems, like batteries and flywheels, also help optimize energy usage by storing excess energy during off-peak hours.
Redundancy and Reliability
Ensure redundancy in your power and cooling systems. This means having backup systems in place to prevent downtime during failures. Reliable systems maintain continuous operations, safeguarding your data and services. Prioritize solutions that offer both redundancy and reliability to build a data center that meets high-performance standards.
By carefully considering these aspects, you can design a data center that not only meets your current needs but also adapts to future demands. This approach ensures your facility remains efficient, reliable, and ready for growth.
Security Measures
Ensuring robust security measures is crucial when you build a data center. You must protect both the physical infrastructure and the digital assets housed within. Let’s delve into the essential security components.
Physical Security
Physical security forms the first line of defense for your data center. You need to implement measures that prevent unauthorized access and protect against physical threats. Consider the following strategies:
Access Control Systems: Install advanced access control systems. These systems use key cards, biometric scanners, or PIN codes to restrict entry. Only authorized personnel should have access to sensitive areas.
Surveillance Cameras: Deploy surveillance cameras throughout the facility. Continuous monitoring deters potential intruders and provides evidence in case of security breaches.
Security Personnel: Employ trained security personnel. They can monitor access points and respond swiftly to any suspicious activity.
Perimeter Fencing: Erect strong perimeter fencing around the data center. This physical barrier acts as a deterrent to unauthorized entry.
By implementing these measures, you can safeguard your data center from physical threats and ensure its operational integrity.
Cybersecurity Protocols
In addition to physical security, you must establish robust cybersecurity protocols. These protocols protect your data center from digital threats and ensure the confidentiality, integrity, and availability of your data. Consider the following practices:
Firewalls and Intrusion Detection Systems (IDS): Deploy firewalls and IDS to monitor network traffic. These tools help detect and block unauthorized access attempts.
Regular Software Updates: Keep all software and systems updated. Regular updates patch vulnerabilities and protect against emerging threats.
Data Encryption: Encrypt sensitive data both at rest and in transit. Encryption ensures that even if data is intercepted, it remains unreadable to unauthorized parties.
Access Management: Implement strict access management policies. Use role-based access control to ensure that users only have access to the data necessary for their roles.
Incident Response Plan: Develop a comprehensive incident response plan. This plan outlines steps to take in the event of a security breach, minimizing damage and ensuring a swift recovery.
By prioritizing these cybersecurity protocols, you can protect your data center from digital threats and maintain the trust of your clients and stakeholders.
Regulatory Compliance
Navigating the regulatory compliance landscape is crucial when building a data center. You must ensure that your facility complies with both local and international standards. This not only protects your business but also builds trust with clients and stakeholders.
Understanding Regulations
Local and International Standards
You need to familiarize yourself with the relevant standards that apply to your data center. These standards vary by location and can impact the design and operation of your facility. For instance, some regions have specific requirements for energy efficiency and environmental impact. By understanding these standards, you can design a data center that meets legal requirements and operates efficiently.
Data Protection Laws
Data protection laws are another critical aspect of regulatory compliance. These laws govern how you collect, store, and process data. They aim to protect personal information and ensure privacy. You must adhere to regulations such as the General Data Protection Regulation (GDPR) in Europe or the California Consumer Privacy Act (CCPA) in the United States. Compliance with these laws not only avoids legal penalties but also enhances your reputation as a trustworthy data handler.
Implementing Compliance Measures
Documentation and Audits
To ensure compliance, you must maintain thorough documentation. This includes records of your data center’s design, operations, and security measures. Regular audits help verify that your facility meets all regulatory requirements. They also identify areas for improvement. By keeping detailed records and conducting audits, you can demonstrate compliance and make informed decisions about your data center’s operations.
Continuous Monitoring
Continuous monitoring is essential for maintaining compliance. You need to implement systems that track your data center’s performance and security. This includes monitoring energy usage, network traffic, and access controls. By doing so, you can quickly identify and address any issues that arise. Continuous monitoring ensures that your data center remains compliant and operates efficiently.
TierPoint, an expert in data center physical security, emphasizes that compliance is a key aspect of data center security. By focusing on both physical and logical security measures, you can protect your facility from unauthorized access and cyber threats.
By understanding and implementing these regulatory compliance measures, you can build a data center that not only meets legal requirements but also operates efficiently and securely. This approach ensures that your facility remains a valuable asset to your business and a trusted partner to your clients.
Procuring Equipment
Procuring the right equipment is a pivotal step in building a data center. The choices you make here will directly impact the performance and reliability of your facility. Let’s explore how to evaluate vendors and select the appropriate hardware.
Vendor Evaluation
Choosing the right vendor is crucial for the success of your data center. You need to ensure that the vendors you select can meet your requirements and provide reliable support.
Criteria for Selection
When evaluating vendors, consider several key criteria:
Product Quality: Ensure that the vendor offers high-quality products that meet industry standards. Reliable equipment minimizes downtime and maintenance costs.
Cost-Effectiveness: Compare prices among different vendors. Look for competitive pricing without compromising on quality.
Scalability: Choose vendors that offer scalable solutions. This ensures that your data center can grow with your business needs.
Compliance: Verify that the vendor complies with relevant regulations. For instance, if your data center handles personal information, ensure the vendor adheres to the General Data Protection Regulation (GDPR) or the Payment Card Industry Data Security Standard (PCI DSS).
Vendor Reliability and Support
Vendor reliability is another critical factor. You need vendors who can provide consistent support and service:
Technical Support: Ensure that the vendor offers robust technical support. This includes 24/7 assistance and quick response times to resolve issues promptly.
Reputation: Research the vendor’s reputation in the industry. Look for reviews and testimonials from other clients to gauge their reliability.
Warranty and Maintenance: Check the warranty and maintenance services offered by the vendor. A good warranty policy can save you from unexpected repair costs.
By carefully evaluating vendors based on these criteria, you can select partners who will contribute to the long-term success of your data center.
Hardware Selection
Selecting the right hardware is essential for building a data center that meets your performance and capacity needs. Let’s look at the key components you need to consider.
Servers and Storage
Servers and storage form the backbone of your data center. You need to choose equipment that aligns with your data processing and storage requirements:
Server Types: Decide on the type of servers you need. Options include rack servers, blade servers, and tower servers. Each type has its advantages, so choose based on your specific needs.
Storage Solutions: Consider your storage needs. Options include hard disk drives (HDDs), solid-state drives (SSDs), and cloud storage. SSDs offer faster data access speeds, while cloud storage provides scalability.
Redundancy: Implement redundancy in your server and storage setup. This ensures data availability and minimizes the risk of data loss.
Networking Equipment
Networking equipment is vital for ensuring seamless connectivity within your data center:
Switches and Routers: Choose high-performance switches and routers. These devices manage data traffic and ensure efficient communication between servers.
Cabling: Select the appropriate cabling infrastructure. Options include fiber optic cables for high-speed data transfer and copper cables for shorter distances.
Network Security: Implement robust network security measures. This includes firewalls and intrusion detection systems to protect against cyber threats.
By selecting the right hardware, you can build a data center that meets your current needs and adapts to future demands. This approach ensures that your facility remains efficient, reliable, and ready for growth.
Installation and Configuration
Setting up your data center involves both physical installation and software configuration. This phase ensures that all components work together seamlessly to deliver optimal performance.
Setup Process
Physical Installation
Begin with the physical installation of your data center equipment. This step involves placing servers, storage units, and networking devices in their designated locations. Ensure that you follow the layout plan meticulously to facilitate efficient airflow and easy access for maintenance. Use Power and Cooling Solutions like uninterruptible power supplies (UPS) and power distribution units (PDUs) to maintain a stable power supply. These solutions help protect your equipment from power fluctuations and outages.
For cooling, consider using the Vertiv Liebert series. These cooling products have become industry standards due to their reliability and efficiency. They help maintain optimal temperature and humidity levels, which are crucial for the longevity of your equipment.
Software Configuration
After the physical setup, proceed with software configuration. Install the necessary operating systems and applications on your servers. Configure network settings to ensure seamless communication between devices. Implement security protocols to protect your data from unauthorized access. Regularly update software to patch vulnerabilities and enhance performance.
Testing and Validation
Once installation and configuration are complete, you must test and validate your data center to ensure it meets performance and security standards.
Performance Testing
Conduct performance testing to evaluate the efficiency of your data center. Test the speed and reliability of data processing and storage systems. Monitor power and cooling systems to ensure they operate within optimal parameters. Identify any bottlenecks or inefficiencies and address them promptly to enhance overall performance.
Security Assessments
Security assessments are vital to safeguarding your data center. Perform vulnerability scans to identify potential security risks. Test your cybersecurity protocols to ensure they effectively protect against threats. Regularly review access controls and update them as needed to prevent unauthorized access. By conducting thorough security assessments, you can maintain the integrity and confidentiality of your data.
By following these steps, you can successfully install and configure your data center. This process ensures that your facility operates efficiently and securely, meeting both current and future demands.
Network and Connectivity
Establishing a robust network and connectivity is crucial for your data center. It ensures seamless communication within the facility and with external networks. Let’s explore how to set up an internal network and establish external connectivity.
Internal Network Setup
Creating an efficient internal network is essential for smooth operations. It involves configuring the Local Area Network (LAN) and segmenting the network for security and performance.
LAN Configuration
You need to configure the LAN to connect all devices within your data center. Start by selecting the appropriate network switches and routers. These devices manage data traffic and ensure efficient communication between servers. Use high-quality Ethernet cables to connect devices. This setup minimizes latency and enhances data transfer speeds.
Implement a structured cabling system. It organizes cables neatly and allows for easy maintenance. Label each cable clearly to avoid confusion during troubleshooting. Regularly monitor the network to identify and resolve any issues promptly.
Network Segmentation
Network segmentation divides your network into smaller, isolated segments. This approach enhances security and performance. You can control access to each segment, reducing the risk of unauthorized access. It also limits the spread of potential threats within the network.
Use Virtual Local Area Networks (VLANs) to segment your network. VLANs allow you to group devices based on function or department. This setup improves traffic management and reduces congestion. Regularly review and update your segmentation strategy to adapt to changing needs.
External Connectivity
Connecting your data center to external networks is vital for accessing the internet and other resources. It involves selecting internet service providers and establishing redundant connections.
Internet Service Providers
Choose reliable internet service providers (ISPs) to ensure consistent connectivity. Evaluate ISPs based on their service quality, speed, and reliability. Consider their reputation and customer reviews. A good ISP provides high-speed internet with minimal downtime.
Negotiate service level agreements (SLAs) with your chosen ISP. SLAs outline the expected service quality and response times for issues. Ensure that the ISP offers robust technical support to address any connectivity problems promptly.
Redundant Connections
Redundant connections provide backup in case of primary connection failure. They ensure continuous connectivity and prevent downtime. Use multiple ISPs to establish redundant connections. This setup diversifies your network paths and reduces the risk of complete outages.
Implement automatic failover systems. These systems switch to backup connections automatically when the primary connection fails. Regularly test your redundant connections to ensure they function correctly.
By focusing on network and connectivity, you can build a data center that operates efficiently and securely. This approach ensures seamless communication within the facility and with external networks, supporting your business objectives effectively.
Disaster Recovery
Disaster recovery is a crucial aspect of data center management. It ensures that your operations continue smoothly even in the face of unexpected disruptions. By planning effectively, you can minimize downtime and protect your valuable data.
Continuity Planning
Continuity planning involves preparing for potential disruptions to maintain business operations. It focuses on creating strategies that ensure your data center remains functional during emergencies.
Backup Solutions
Implementing robust backup solutions is essential for data protection. You should regularly back up your data to prevent loss during system failures or disasters. Consider using both on-site and off-site backups for added security. On-site backups provide quick access to data, while off-site backups protect against local disasters. Cloud storage offers a flexible and scalable option for off-site backups. Regularly test your backup systems to ensure they function correctly and can restore data efficiently.
Failover Strategies
Failover strategies are vital for maintaining service availability. They involve switching to backup systems when primary systems fail. You should design your data center with redundancy in mind. Use redundant servers, storage devices, and networking equipment to ensure continuous operations. Implement automatic failover mechanisms to minimize downtime. Regularly test your failover systems to verify their effectiveness and make necessary adjustments.
Security Measures
Security measures play a critical role in disaster recovery. They help detect and respond to threats, ensuring the integrity and availability of your data.
Threat Detection
Threat detection involves identifying potential security risks before they cause harm. You should deploy advanced monitoring tools to track network activity and detect anomalies. Firewalls and intrusion detection systems (IDS) are essential components of threat detection. They monitor incoming and outgoing traffic, blocking unauthorized access attempts. Regularly update your security software to protect against emerging threats. Conduct vulnerability assessments to identify and address potential weaknesses in your systems.
Incident Response
Incident response is the process of managing and mitigating security breaches. You should develop a comprehensive incident response plan outlining steps to take during a security incident. This plan should include procedures for identifying, containing, and eradicating threats. Train your staff to recognize and respond to security incidents promptly. Conduct regular drills to test your incident response capabilities and improve your strategies. By preparing for potential threats, you can minimize damage and ensure a swift recovery.
Data Center Networking vs. Data Center Storage Devices: Networking focuses on connectivity and communication, while storage devices handle data retention. Both are crucial for effective disaster recovery, ensuring data accessibility and protection.
By prioritizing disaster recovery, you can build a resilient data center that withstands disruptions and maintains operational continuity. This approach safeguards your data and supports your business objectives, providing peace of mind in an unpredictable world.
Environmental Impact
Understanding the environmental impact of your data center is crucial. You can make a significant difference by adopting sustainable practices and reducing your carbon footprint. Let’s explore how you can achieve this.
Sustainability Considerations
Green Technologies
Incorporating green technologies into your data center can greatly enhance its sustainability. You should consider using energy-efficient servers and cooling systems. These technologies reduce energy consumption and lower operational costs. For instance, liquid cooling systems are more efficient than traditional air cooling. They use less energy and provide better temperature control. By investing in green technologies, you contribute to a healthier planet and improve your data center’s efficiency.
Energy-Efficient Practices
Adopting energy-efficient practices is another way to minimize your data center’s environmental impact. Start by optimizing your power usage. Implement power management software to monitor and control energy consumption. This software helps identify areas where you can reduce energy use. Additionally, consider using virtualization to consolidate workloads. Virtualization reduces the number of physical servers needed, which lowers energy consumption. Regularly review and update your energy management strategies to ensure continuous improvement.
Reducing Carbon Footprint
Renewable Energy Sources
Switching to renewable energy sources is an effective way to reduce your data center’s carbon footprint. You can use solar panels or wind turbines to generate clean energy. These sources provide a sustainable alternative to fossil fuels. Many data centers are now investing in renewable energy to power their operations. By doing so, you not only reduce greenhouse gas emissions but also decrease your reliance on non-renewable resources. Explore partnerships with renewable energy providers to secure a stable and eco-friendly energy supply.
Waste Management
Effective waste management is essential for minimizing your data center’s environmental impact. You should implement recycling programs for electronic waste. Proper disposal of old servers, cables, and other equipment prevents harmful substances from entering the environment. Additionally, consider using biodegradable materials for packaging and shipping. This reduces the amount of waste generated by your data center. Regularly assess your waste management practices to identify areas for improvement and ensure compliance with environmental regulations.
“Data centers should always err on the side of caution,” emphasizes the importance of disaster recovery in data center planning. By focusing on sustainability and reducing your carbon footprint, you can build a resilient data center that supports business continuity and minimizes environmental impact.
Cost Analysis
Analyzing the costs involved in building and operating a data center is crucial. This section will guide you through understanding the initial investment, operational expenses, and future trends that can impact your financial planning.
Initial Investment
Construction and Equipment Costs
When you embark on building a data center, construction and equipment costs form a significant part of your initial investment. You need to budget for the physical infrastructure, including the building, power systems, and cooling solutions. The choice of equipment, such as servers, storage devices, and networking gear, also impacts your costs. Opting for energy-efficient technologies can reduce long-term expenses. Sustainable Practices and Technologies for Data Centers highlight that incorporating efficient cooling mechanisms and renewable energy sources can minimize environmental impact and operational costs.
Licensing and Permits
Securing the necessary licenses and permits is another essential step. You must comply with local regulations and obtain permits for construction, operation, and environmental impact. These legal requirements ensure that your data center meets safety and environmental standards. Failing to secure the proper documentation can lead to delays and additional costs. Planning ahead and consulting with legal experts can streamline this process and prevent unforeseen expenses.
Operational Expenses
Maintenance and Staffing
Once your data center is operational, maintenance and staffing become ongoing expenses. Regular maintenance ensures that your equipment functions optimally and reduces the risk of downtime. You need skilled personnel to manage and maintain the facility. Investing in training and development for your staff can enhance their efficiency and reduce turnover. By prioritizing maintenance and staffing, you can ensure the smooth operation of your data center.
Energy Consumption
Energy consumption is a major operational expense for data centers. According to Global Energy Consumption and Greenhouse Gas Emissions from Data Centers, these facilities account for about 4% of global energy consumption. Implementing energy-efficient practices can significantly reduce your energy costs. Consider using smart energy management systems and renewable energy sources to optimize power usage. Monitoring your energy consumption regularly helps identify areas for improvement and cost savings.
Future Trends
Technological Advancements
Staying informed about technological advancements is vital for future-proofing your data center. Innovations in server technology, cooling systems, and energy management can enhance efficiency and reduce costs. Adopting new technologies early can give you a competitive edge and improve your data center’s performance. Keep an eye on industry trends and invest in research and development to stay ahead.
Market Demands
Understanding market demands is crucial for adapting your data center to changing needs. As data consumption grows, the demand for efficient and sustainable data centers increases. Efficiency and Sustainability of Data Centers emphasize the importance of addressing environmental concerns and adopting sustainable practices. By aligning your data center with market demands, you can attract more clients and ensure long-term success.
In conclusion, a thorough cost analysis helps you plan effectively for building and operating a data center. By considering initial investments, operational expenses, and future trends, you can make informed decisions that support your business objectives and ensure financial sustainability.
Building a data center from scratch involves several key steps. You start by assessing your needs and designing the infrastructure. Then, you procure equipment and ensure regulatory compliance. Installation and configuration follow, along with establishing network connectivity and disaster recovery plans. Ongoing maintenance and updates are crucial for optimal performance. Stay informed about industry trends and innovations to keep your data center competitive. If you’re ready to embark on this journey, consider your budget and long-term goals. A mid-sized data center can cost between $2 million and $5 million, so plan accordingly.
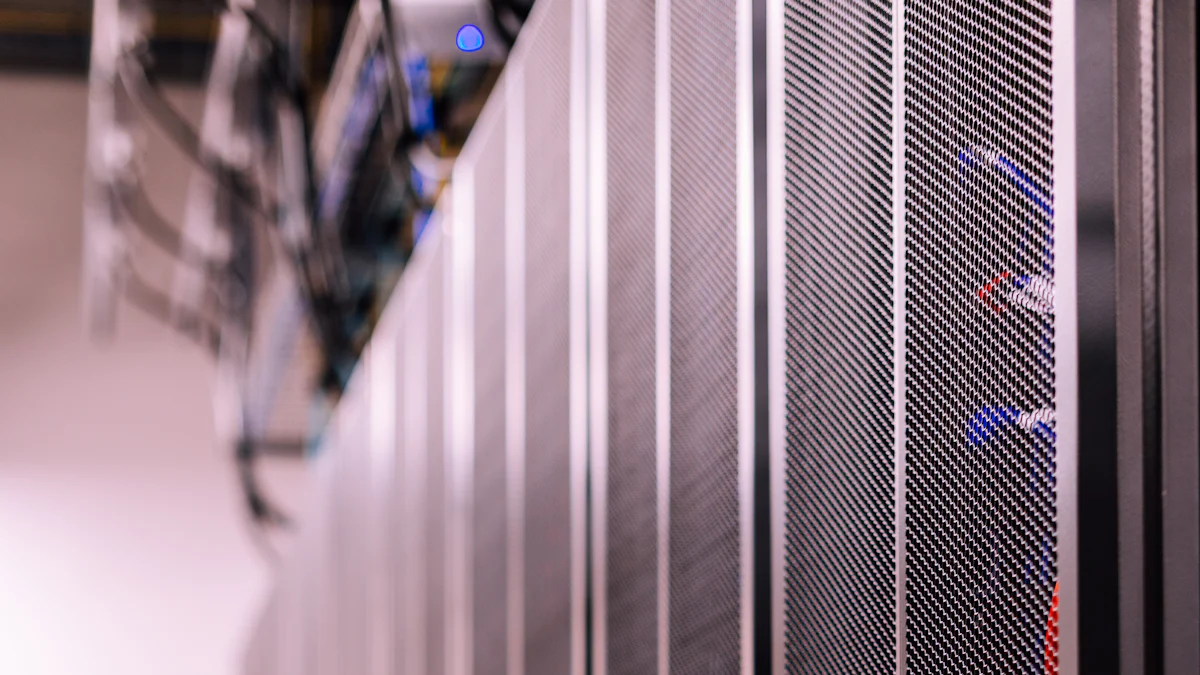
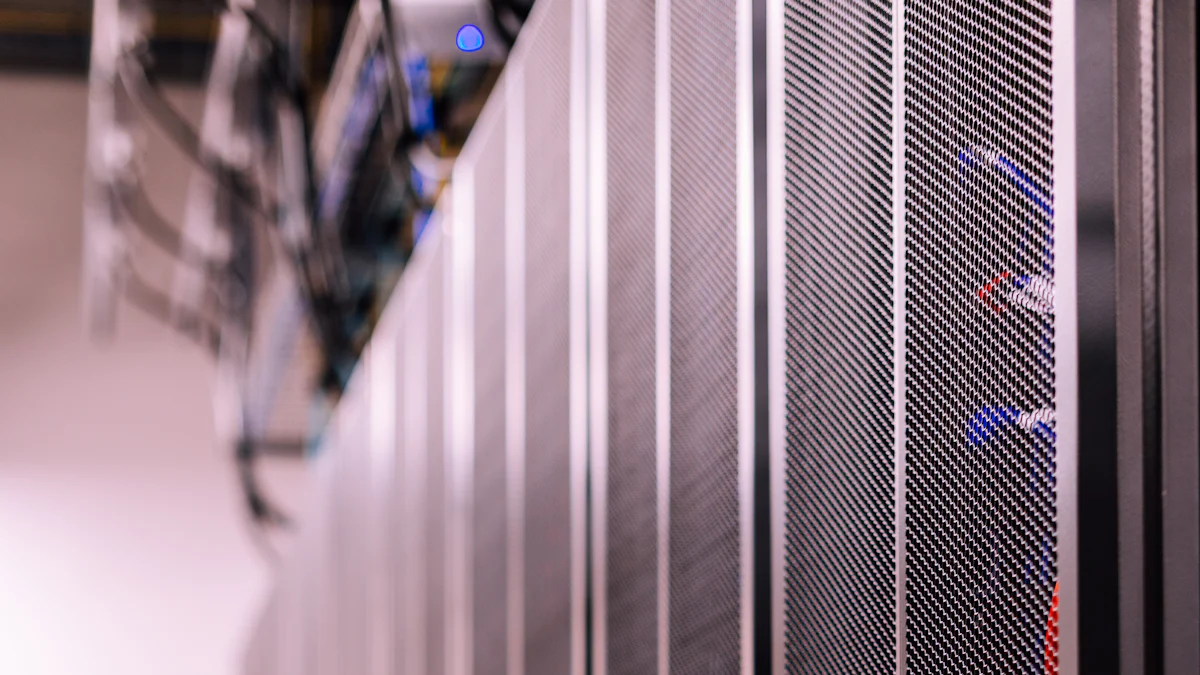
Managing a data center can be complex, but openDCIM simplifies the process. This powerful tool offers a comprehensive solution for tracking assets and managing infrastructure efficiently. You will find openDCIM particularly beneficial as it integrates seamlessly with financial systems, saving both time and money. Its popularity among IT professionals is growing due to its ability to streamline networks and support sustainable growth. With features tailored to meet diverse business needs, openDCIM stands out as a preferred choice for data center management. By using openDCIM, you ensure accurate inventory management and enhance operational efficiency.
Preparing for openDCIM Installation
Before you dive into the installation of openDCIM, it’s crucial to ensure that your system meets the necessary requirements. This preparation will help you avoid potential issues and streamline the installation process.
System Requirements
To successfully install openDCIM, your system must meet specific criteria. Here’s what you need:
Web Host: Your server should run Apache 2.x or higher. Ensure that your site is SSL-enabled for secure data transmission.
Database: A MySQL 5.x or higher database is essential. This database will store all your data center information.
PHP Version: PHP 5.3 or higher is required. This scripting language supports the dynamic features of openDCIM.
User Authentication: Implement a robust user authentication system to manage access and maintain security.
Web-Based Client: Access openDCIM through a web-based client, allowing you to manage your data center from anywhere.
Pre-Installation Checklist
Before proceeding with the installation, go through this checklist to ensure everything is in place:
Verify System Compatibility: Double-check that your server meets all the system requirements listed above.
Backup Data: If you’re upgrading from a previous version, back up your existing data to prevent any loss.
Download openDCIM: Obtain the latest version of openDCIM from the official website. This ensures you have the most recent features and security updates.
Prepare the Server: Configure your server settings to accommodate openDCIM. This includes setting up the database and ensuring PHP is correctly installed.
Check Network Configuration: Ensure your network settings allow for seamless communication between openDCIM and other systems.
By following these steps, you prepare your environment for a smooth openDCIM installation. Proper preparation minimizes errors and enhances the efficiency of your data center management.
Installing openDCIM
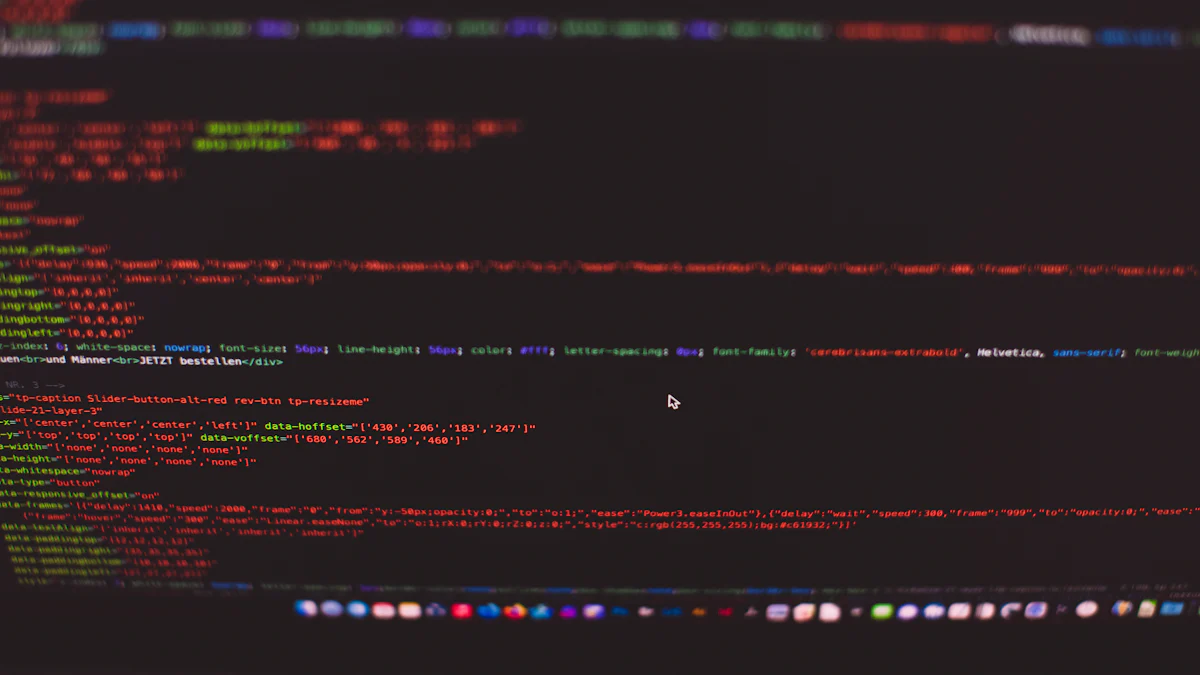
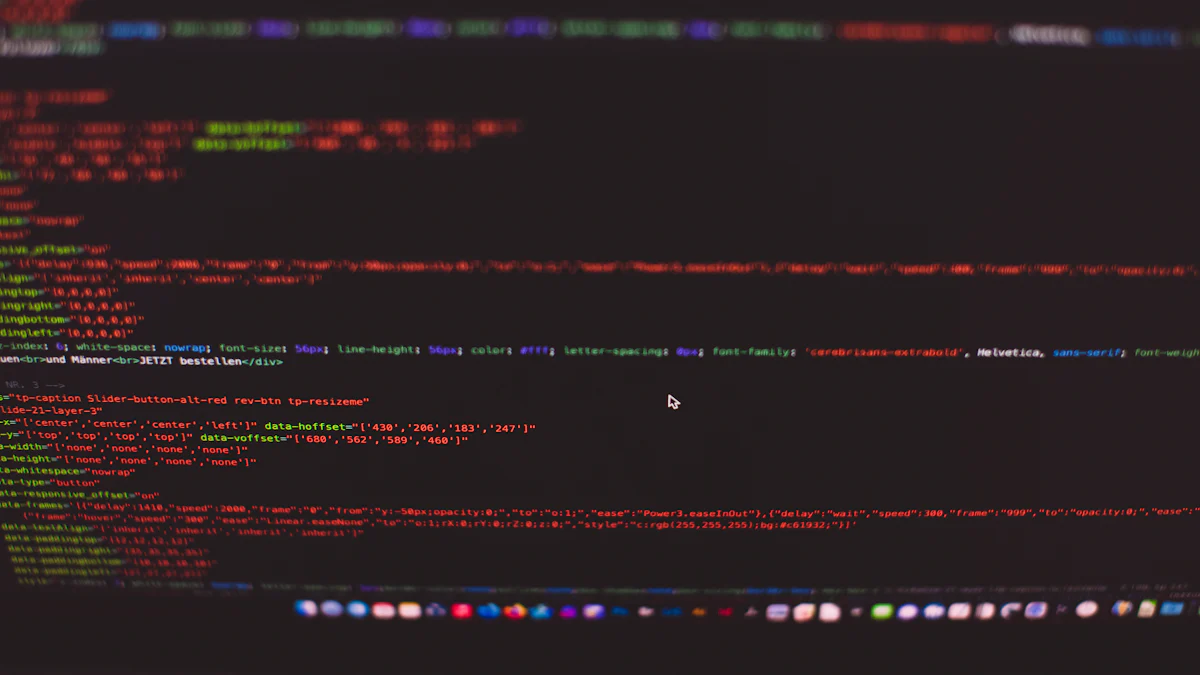
Once you have prepared your system, you can proceed with the installation of openDCIM. This section will guide you through downloading and installing this powerful tool for data center management.
Downloading openDCIM
To begin, you need to download the openDCIM package. Follow these steps to ensure a smooth download process:
Visit the Official Website: Navigate to the official openDCIM website or its GitHub repository. These platforms host the latest version of openDCIM, ensuring you access the most recent features and security updates.
Select the Latest Version: Choose the most recent package link for openDCIM. This ensures compatibility with your system and provides the latest enhancements.
Download the Package: Click on the download link to obtain the openDCIM package. Save it to a location on your server where you can easily access it later.
“openDCIM, created by Scott Milliken, is available for download from the official openDCIM website and GitHub.”
By following these steps, you ensure that you have the correct version of openDCIM ready for installation.
Installation Process
With the openDCIM package downloaded, you can now proceed with the installation. Here’s how to install openDCIM on your server:
Access the Server Directory: Open your server’s terminal and navigate to the directory where you saved the openDCIM package. For example, if you wish to install openDCIM under the
/var/www
directory, use the command line to move to this location.Extract the Package: Use a command such as
tar -xzf
followed by the package name to extract the contents. This action unpacks the files necessary for installation.Configure the Web Host: Ensure your web host runs Apache 2.x or higher with SSL enabled. This setup is crucial for secure data transmission.
Set Up the Database: Create a MySQL database for openDCIM. This database will store all your data center information. Use commands like
CREATE DATABASE
in MySQL to set this up.Run the Installation Script: Open a web browser and enter the URL pointing to the openDCIM directory on your server. This action initiates the web-based installation script. Follow the on-screen instructions to complete the installation.
Verify Installation: Once the installation completes, verify that openDCIM is functioning correctly. Check that you can access the dashboard and that all features are operational.
By carefully following these steps, you install openDCIM efficiently, setting the stage for effective data center management.
Configuring openDCIM
Once you have installed openDCIM, the next step involves configuring it to suit your data center’s specific needs. This configuration process ensures that openDCIM operates efficiently and aligns with your management objectives.
Initial Setup
Begin by setting up openDCIM to reflect your data center’s infrastructure. This initial setup is crucial for accurate asset tracking and management.
Define Data Center Locations: Start by entering the physical locations of your data centers. This information helps in organizing and managing assets across different sites.
Add Cabinets and Racks: Input the details of all cabinets and racks within your data centers. This step allows openDCIM to map out your physical infrastructure accurately.
Input Asset Information: Enter the details of all assets, including servers, switches, and other equipment. Accurate asset information is vital for effective inventory management.
Set User Permissions: Configure user permissions to control access to different sections of openDCIM. This feature enhances security by ensuring that only authorized personnel can modify critical data.
Integrate with Financial Systems: If applicable, integrate openDCIM with your financial systems. This integration streamlines processes such as budgeting and cost tracking.
“OpenDCIM provides highly efficient and reliable data center services, supporting asset tracking and integrating with popular financial systems.”
By completing these steps, you lay a solid foundation for using openDCIM effectively.
Customizing Settings
After the initial setup, customize openDCIM settings to optimize its functionality for your specific requirements.
Adjust Environmental Monitoring: Configure settings for monitoring environmental factors like temperature and humidity. This feature helps in maintaining optimal conditions for your equipment.
Set Power Management Preferences: Customize power management settings to monitor and control energy consumption. Efficient power management reduces costs and supports sustainability goals.
Configure Alerts and Notifications: Set up alerts for critical events such as equipment failures or environmental changes. Timely notifications enable prompt responses to potential issues.
Tailor Reporting Features: Customize reporting features to generate insights that are most relevant to your operations. Tailored reports aid in decision-making and strategic planning.
Update Software Regularly: Keep openDCIM updated to benefit from the latest features and security enhancements. Regular updates ensure that the software remains reliable and efficient.
By customizing these settings, you enhance openDCIM’s ability to manage your data center effectively. This customization ensures that the software meets your unique operational needs.
Exploring Key Features of openDCIM
Asset Management
OpenDCIM offers a robust asset management system that simplifies tracking and managing your data center components. You can efficiently inventory items on-site, gaining access to crucial details such as installation dates, average power parameters, and device types in seconds. This feature helps you maintain an accurate record of all assets, ensuring that you can easily locate and manage them.
Efficient Tracking: OpenDCIM allows you to track assets of any size effectively. You can monitor equipment location, power usage, and capacity planning, all integrated into one centralized platform.
Integration with Financial Systems: The tool integrates seamlessly with popular financial systems like FreshBooks and QuickBooks. This integration helps you manage finances while keeping track of assets, saving both time and money.
User-Friendly Interface: The intuitive interface makes it easy for you to navigate and manage your data center assets. You can quickly access important information and make informed decisions about your infrastructure.
“OpenDCIM provides powerful features for improved asset tracking and increased efficiency when managing data centers of all sizes.”
By utilizing these asset management features, you can enhance the efficiency of your data center operations and ensure that your infrastructure remains organized and well-maintained.
Power and Environmental Monitoring
Monitoring power and environmental conditions is crucial for maintaining optimal data center performance. OpenDCIM provides comprehensive tools to help you achieve this goal.
Power Management: You can customize power management settings to monitor and control energy consumption. This feature supports sustainability goals by reducing costs and optimizing resource utilization.
Environmental Monitoring: OpenDCIM allows you to configure settings for monitoring environmental factors like temperature and humidity. Maintaining optimal conditions helps protect your equipment and ensures reliable performance.
Alerts and Notifications: Set up alerts for critical events such as equipment failures or environmental changes. Timely notifications enable you to respond promptly to potential issues, minimizing downtime and maintaining operational efficiency.
Real-Time Information: The platform provides real-time information about components connected inside cabinets. This capability makes managing large installations easier and more efficient.
“OpenDCIM offers versatile compatibility options, allowing integration into network hardware inventorying systems, giving technical specialists real-time information about components.”
By leveraging these power and environmental monitoring features, you can ensure that your data center operates smoothly and efficiently, safeguarding your valuable assets and resources.
Best Practices for Using openDCIM
Regular Maintenance
Regular maintenance is crucial for ensuring that openDCIM operates smoothly and efficiently. By following a consistent maintenance schedule, you can prevent potential issues and extend the lifespan of your data center infrastructure.
Update Software Regularly: Keep openDCIM updated to benefit from the latest features and security enhancements. Regular updates ensure that the software remains reliable and efficient.
Perform Routine Backups: Regularly back up your data to prevent loss in case of system failures or cyberattacks. This practice safeguards your valuable information and ensures business continuity.
Monitor System Performance: Regularly check the performance of openDCIM to identify any bottlenecks or inefficiencies. Monitoring helps you address issues promptly and maintain optimal system functionality.
Review Asset Inventory: Periodically review and update your asset inventory to ensure accuracy. Accurate records help in effective asset management and decision-making.
Conduct Regular Audits: Perform regular audits of your data center operations to ensure compliance with industry standards and regulations. Audits help identify areas for improvement and enhance overall efficiency.
“Regular maintenance of openDCIM not only ensures smooth operation but also extends the lifespan of your data center infrastructure.”
By adhering to these maintenance practices, you can optimize the performance of openDCIM and enhance the efficiency of your data center operations.
Security Enhancements
Enhancing security is vital for protecting your data center from unauthorized access and cyber threats. Implementing Enhancing security is vital ensures the safety and integrity of your systems.
Implement Access Controls: Use robust access controls to manage who can access different sections of openDCIM. This measure prevents unauthorized access and protects sensitive information.
Use Encryption Protocols: Encrypt data to secure it during transmission and storage. Encryption protects your data from being intercepted or accessed by unauthorized parties.
Enable Multi-Factor Authentication: Implement multi-factor authentication to add an extra layer of security. This feature requires users to provide additional verification, reducing the risk of unauthorized access.
Conduct Regular Security Audits: Perform regular security audits to identify vulnerabilities and ensure compliance with industry regulations. Audits help you strengthen your security posture and protect against potential threats.
Monitor Compliance: Continuously monitor compliance with industry standards and regulations. Compliance monitoring ensures that your data center operations adhere to legal and ethical requirements.
“OpenDCIM provides users with peace of mind by using secure technologies such as vaulted storage, encryption algorithms, and multi-factor authentication.”
By implementing these security enhancements, you can protect your data center from external threats and ensure compliance with industry standards.
Troubleshooting Common Issues
When using openDCIM, you might encounter some common issues. Understanding how to troubleshoot these problems can help you maintain smooth operations in your data center.
Installation Errors
Installation errors can occur if your system does not meet the necessary requirements or if there are configuration issues. Here’s how you can address these errors:
Verify System Requirements: Ensure your server meets all the specified requirements for openDCIM. Check the web host, database, and PHP version. These components must align with openDCIM’s needs to function correctly.
Check Configuration Files: Review your configuration files for any errors. Incorrect settings can lead to installation failures. Pay attention to database connection details and file paths.
Consult Documentation: Refer to the openDCIM documentation for guidance. The documentation provides detailed instructions and troubleshooting tips for common installation issues.
Seek Community Support: Engage with the openDCIM community. Many users share their experiences and solutions to similar problems. You can find forums and discussion groups online.
Reinstall if Necessary: If errors persist, consider reinstalling openDCIM. Ensure you follow each step carefully to avoid repeating the same mistakes.
“OpenDCIM is becoming an invaluable resource for tech professionals across industries, offering easy integration with third-party software applications.”
By following these steps, you can resolve installation errors and get openDCIM up and running efficiently.
Performance Optimization
Optimizing the performance of openDCIM ensures that your data center operates at its best. Here are some strategies to enhance performance:
Regular Updates: Keep openDCIM updated. Updates often include performance improvements and bug fixes. Staying current ensures you benefit from these enhancements.
Monitor Resource Usage: Track the resource usage of openDCIM. High CPU or memory usage can indicate inefficiencies. Use monitoring tools to identify and address these issues.
Optimize Database Performance: Ensure your database is optimized. Regularly clean up unnecessary data and index tables to improve query performance.
Adjust Configuration Settings: Fine-tune openDCIM’s configuration settings. Adjusting parameters like cache size and timeout settings can improve responsiveness.
Conduct Performance Audits: Perform regular audits of openDCIM’s performance. Identify bottlenecks and areas for improvement. Audits help maintain optimal functionality.
“OpenDCIM can benefit businesses through improved operations, reduced costs, and increased visibility over IT activities.”
By implementing these optimization strategies, you can enhance openDCIM’s performance and ensure efficient data center management.
You have now explored the essential steps for using opendcim effectively in your data center management. By following this guide, you can streamline operations and enhance asset tracking. OpenDCIM offers significant advantages, such as integration with financial systems and comprehensive IT infrastructure management. These features save time and reduce costs. As you continue to use opendcim, consider exploring its extensive features and engaging with community resources. This exploration will help you unlock the full potential of opendcim and further improve your data center operations.